Curvature Aligned Simplex Gradient: Principled Sample Set Construction For Numerical Differentiation
CoRR(2023)
摘要
The simplex gradient, a popular numerical differentiation method due to its flexibility, lacks a principled method by which to construct the sample set, specifically the location of function evaluations. Such evaluations, especially from real-world systems, are often noisy and expensive to obtain, making it essential that each evaluation is carefully chosen to reduce cost and increase accuracy. This paper introduces the curvature aligned simplex gradient (CASG), which provably selects the optimal sample set under a mean squared error objective. As CASG requires function-dependent information often not available in practice, we additionally introduce a framework which exploits a history of function evaluations often present in practical applications. Our numerical results, focusing on applications in sensitivity analysis and derivative free optimization, show that our methodology significantly outperforms or matches the performance of the benchmark gradient estimator given by forward differences (FD) which is given exact function-dependent information that is not available in practice. Furthermore, our methodology is comparable to the performance of central differences (CD) that requires twice the number of function evaluations.
更多查看译文
关键词
curvature aligned simplex gradient,principled sample set construction
AI 理解论文
溯源树
样例
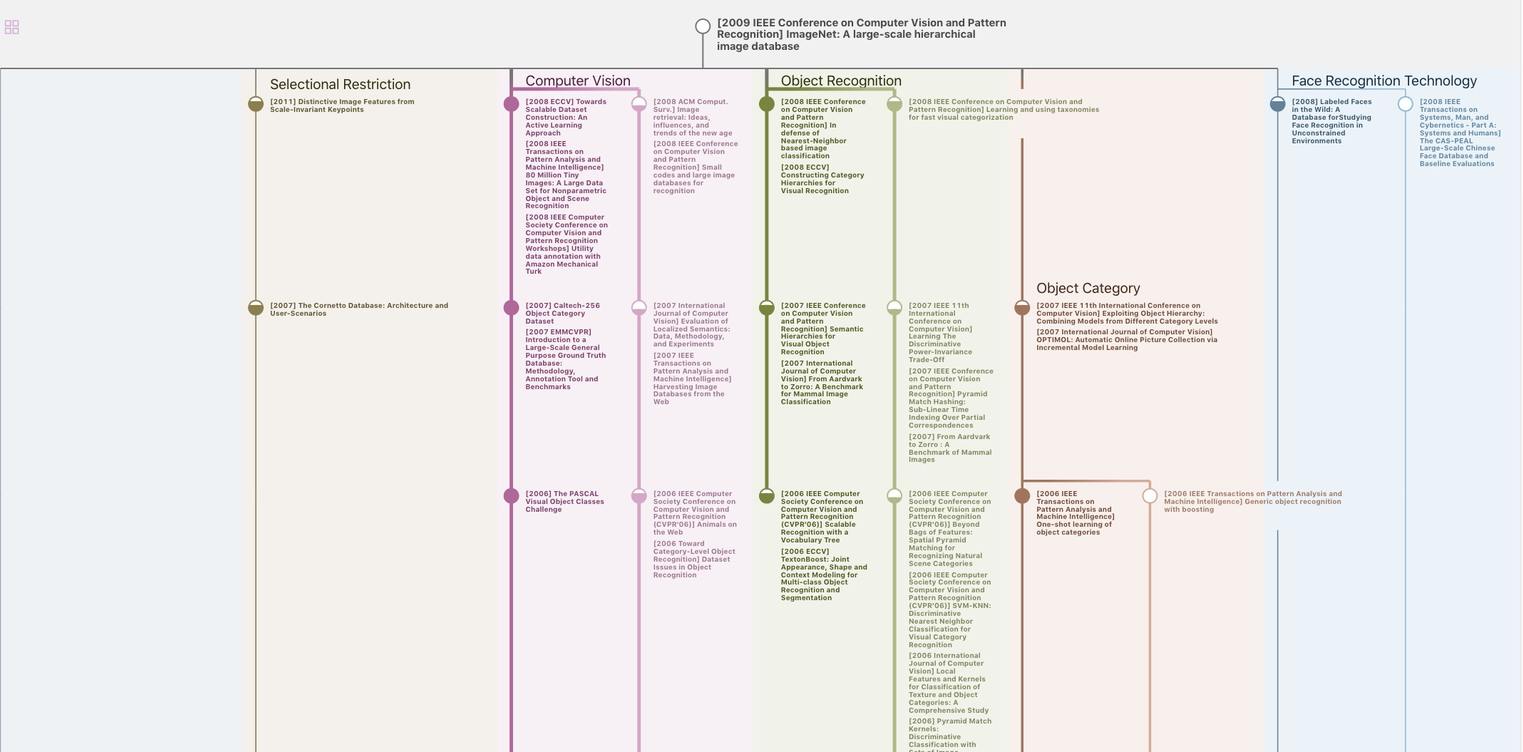
生成溯源树,研究论文发展脉络
Chat Paper
正在生成论文摘要