Diverse Diffusion: Enhancing Image Diversity in Text-to-Image Generation
CoRR(2023)
摘要
Latent diffusion models excel at producing high-quality images from text. Yet, concerns appear about the lack of diversity in the generated imagery. To tackle this, we introduce Diverse Diffusion, a method for boosting image diversity beyond gender and ethnicity, spanning into richer realms, including color diversity.Diverse Diffusion is a general unsupervised technique that can be applied to existing text-to-image models. Our approach focuses on finding vectors in the Stable Diffusion latent space that are distant from each other. We generate multiple vectors in the latent space until we find a set of vectors that meets the desired distance requirements and the required batch size.To evaluate the effectiveness of our diversity methods, we conduct experiments examining various characteristics, including color diversity, LPIPS metric, and ethnicity/gender representation in images featuring humans.The results of our experiments emphasize the significance of diversity in generating realistic and varied images, offering valuable insights for improving text-to-image models. Through the enhancement of image diversity, our approach contributes to the creation of more inclusive and representative AI-generated art.
更多查看译文
关键词
image diversity,generation,diffusion,text-to-image
AI 理解论文
溯源树
样例
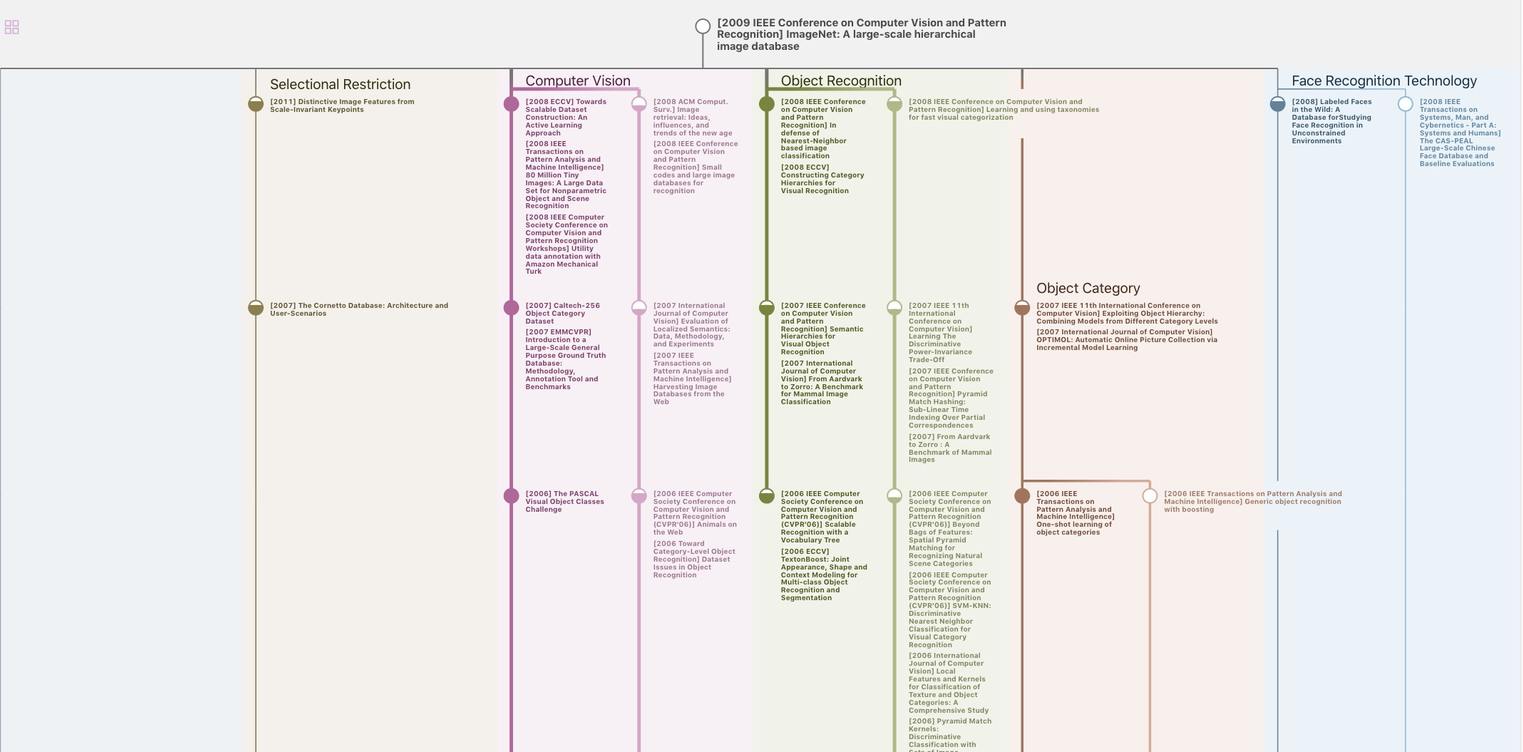
生成溯源树,研究论文发展脉络
Chat Paper
正在生成论文摘要