Not Just Learning from Others but Relying on Yourself: A New Perspective on Few-Shot Segmentation in Remote Sensing
CoRR(2023)
摘要
Few-shot segmentation (FSS) is proposed to segment unknown class targets with just a few annotated samples. Most current FSS methods follow the paradigm of mining the semantics from the support images to guide the query image segmentation. However, such a pattern of `learning from others' struggles to handle the extreme intra-class variation, preventing FSS from being directly generalized to remote sensing scenes. To bridge the gap of intra-class variance, we develop a Dual-Mining network named DMNet for cross-image mining and self-mining, meaning that it no longer focuses solely on support images but pays more attention to the query image itself. Specifically, we propose a Class-public Region Mining (CPRM) module to effectively suppress irrelevant feature pollution by capturing the common semantics between the support-query image pair. The Class-specific Region Mining (CSRM) module is then proposed to continuously mine the class-specific semantics of the query image itself in a `filtering' and `purifying' manner. In addition, to prevent the co-existence of multiple classes in remote sensing scenes from exacerbating the collapse of FSS generalization, we also propose a new Known-class Meta Suppressor (KMS) module to suppress the activation of known-class objects in the sample. Extensive experiments on the iSAID and LoveDA remote sensing datasets have demonstrated that our method sets the state-of-the-art with a minimum number of model parameters. Significantly, our model with the backbone of Resnet-50 achieves the mIoU of 49.58% and 51.34% on iSAID under 1-shot and 5-shot settings, outperforming the state-of-the-art method by 1.8% and 1.12%, respectively. The code is publicly available at https://github.com/HanboBizl/DMNet.
更多查看译文
关键词
remote sensing,segmentation,learning,few-shot
AI 理解论文
溯源树
样例
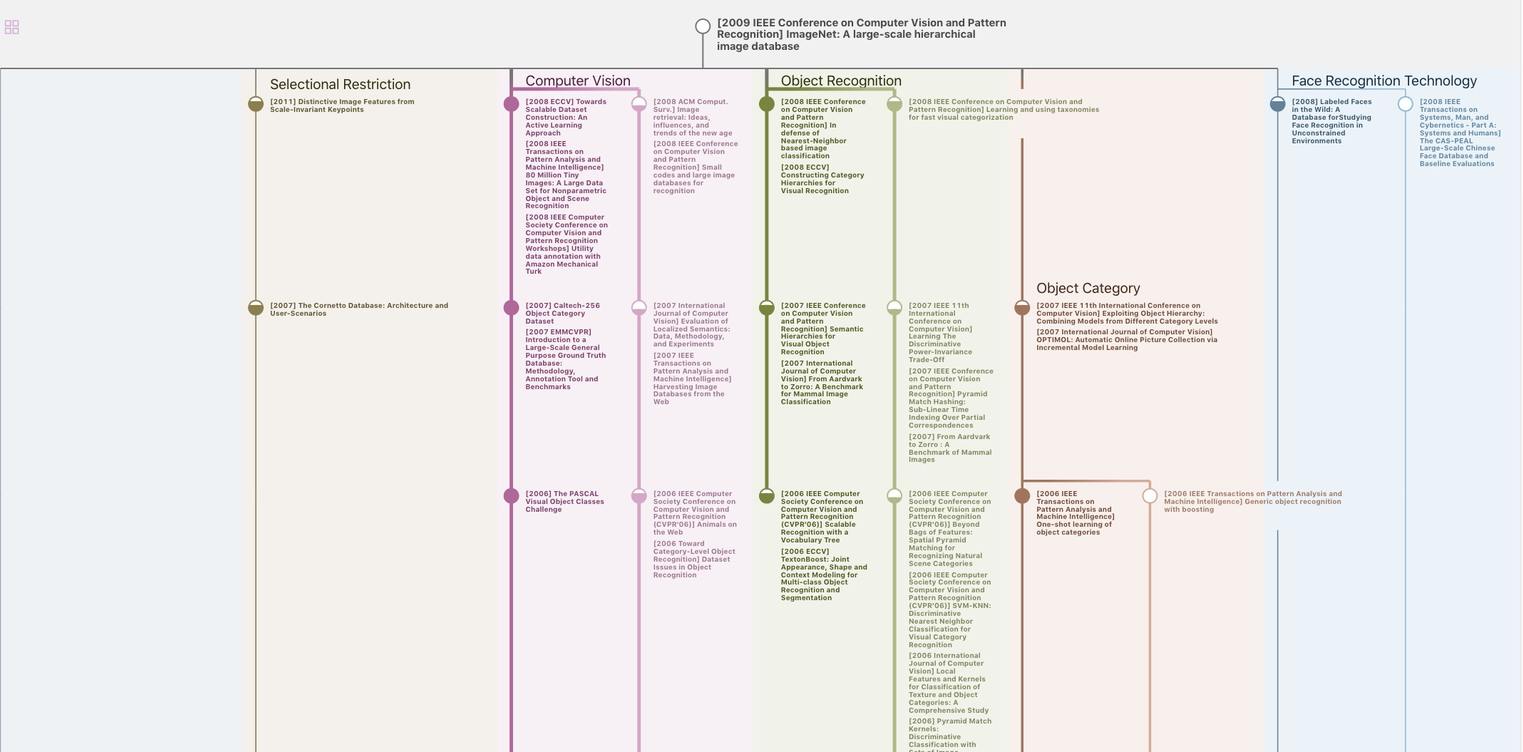
生成溯源树,研究论文发展脉络
Chat Paper
正在生成论文摘要