Optimal Excess Risk Bounds for Empirical Risk Minimization on $p$-norm Linear Regression
NeurIPS(2023)
摘要
We study the performance of empirical risk minimization on the $p$-norm linear regression problem for $p \in (1, \infty)$. We show that, in the realizable case, under no moment assumptions, and up to a distribution-dependent constant, $O(d)$ samples are enough to exactly recover the target. Otherwise, for $p \in [2, \infty)$, and under weak moment assumptions on the target and the covariates, we prove a high probability excess risk bound on the empirical risk minimizer whose leading term matches, up to a constant that depends only on $p$, the asymptotically exact rate. We extend this result to the case $p \in (1, 2)$ under mild assumptions that guarantee the existence of the Hessian of the risk at its minimizer.
更多查看译文
关键词
optimal excess risk bounds,empirical risk minimization
AI 理解论文
溯源树
样例
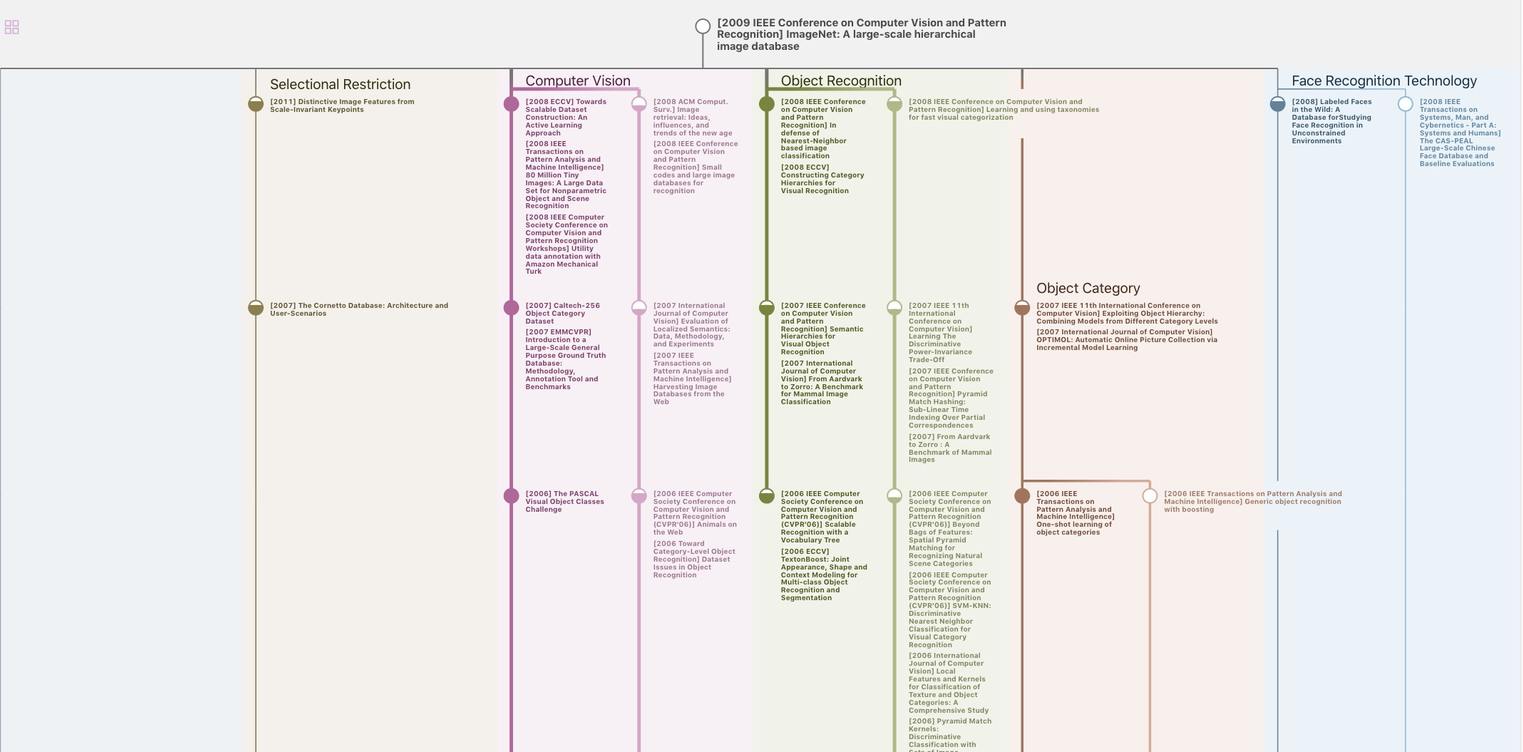
生成溯源树,研究论文发展脉络
Chat Paper
正在生成论文摘要