Achieving optimal complexity guarantees for a class of bilevel convex optimization problems
arxiv(2023)
摘要
We design and analyze a novel accelerated gradient-based algorithm for a class of bilevel optimization problems. These problems have various applications arising from machine learning and image processing, where optimal solutions of the two levels are interdependent. That is, achieving the optimal solution of an upper-level problem depends on the solution set of a lower-level optimization problem. We significantly improve existing convergence rates to $O(\epsilon^{-0.5})$ for both suboptimality and infeasibility error metrics. In addition, contrary to existing methods that require solving the optimization problem sequentially (initially solving an optimization problem to approximate the solution of the lower-level problem followed by a second algorithm), our algorithm concurrently solves the optimization problem. To the best of our knowledge, the proposed algorithm has the fastest known iteration complexity, which matches the rate of the fastest method for single-level optimization. We conduct numerical experiments on sparse linear regression problems to demonstrate the efficacy of our approach.
更多查看译文
AI 理解论文
溯源树
样例
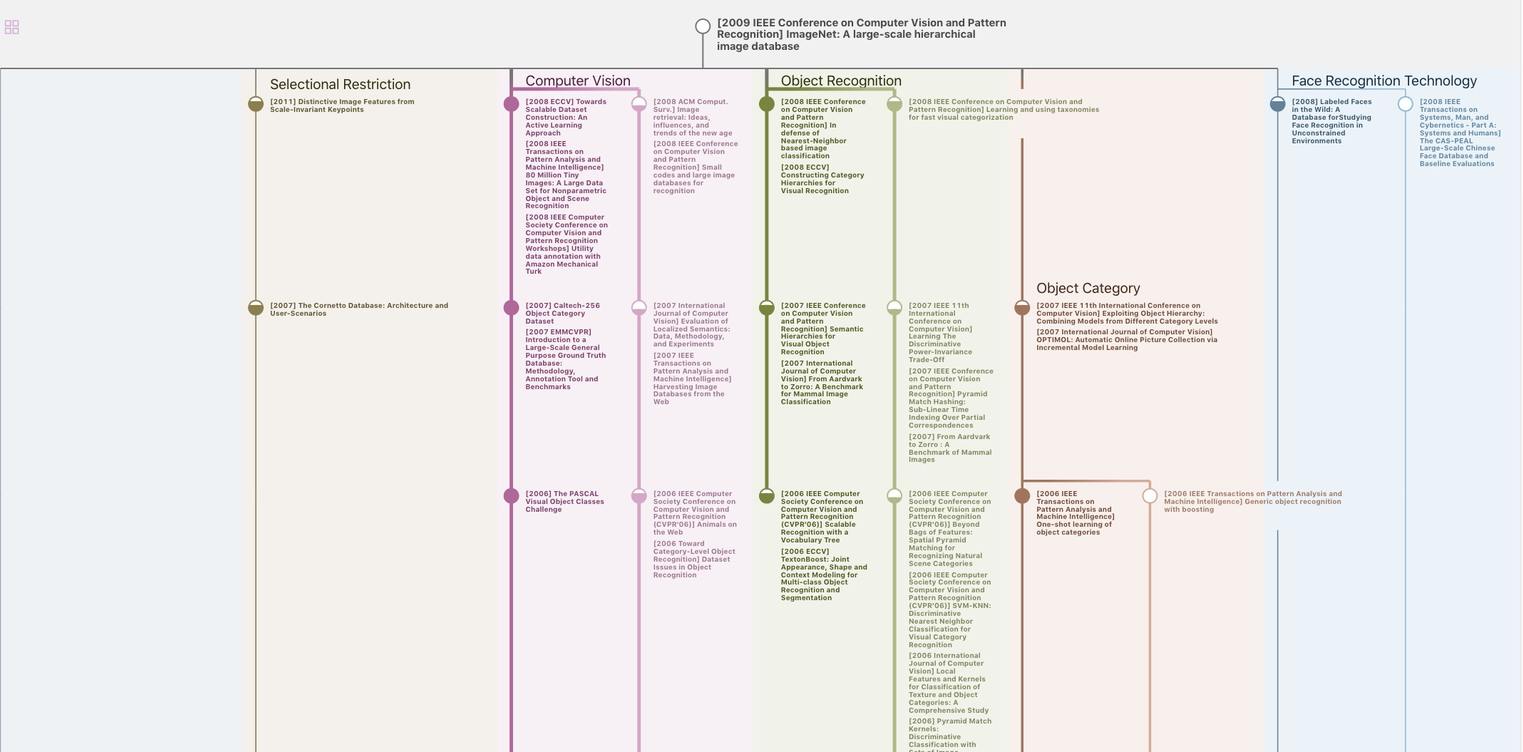
生成溯源树,研究论文发展脉络
Chat Paper
正在生成论文摘要