Unsupervised learning for medical data: A review of probabilistic factorization methods.
Statistics in medicine(2023)
摘要
We review popular unsupervised learning methods for the analysis of high-dimensional data encountered in, for example, genomics, medical imaging, cohort studies, and biobanks. We show that four commonly used methods, principal component analysis, K-means clustering, nonnegative matrix factorization, and latent Dirichlet allocation, can be written as probabilistic models underpinned by a low-rank matrix factorization. In addition to highlighting their similarities, this formulation clarifies the various assumptions and restrictions of each approach, which eases identifying the appropriate method for specific applications for applied medical researchers. We also touch upon the most important aspects of inference and model selection for the application of these methods to health data.
更多查看译文
关键词
clustering, dimension reduction, health-care research, latent variable discovery, probabilistic matrix factorization, topic model, unsupervised learning
AI 理解论文
溯源树
样例
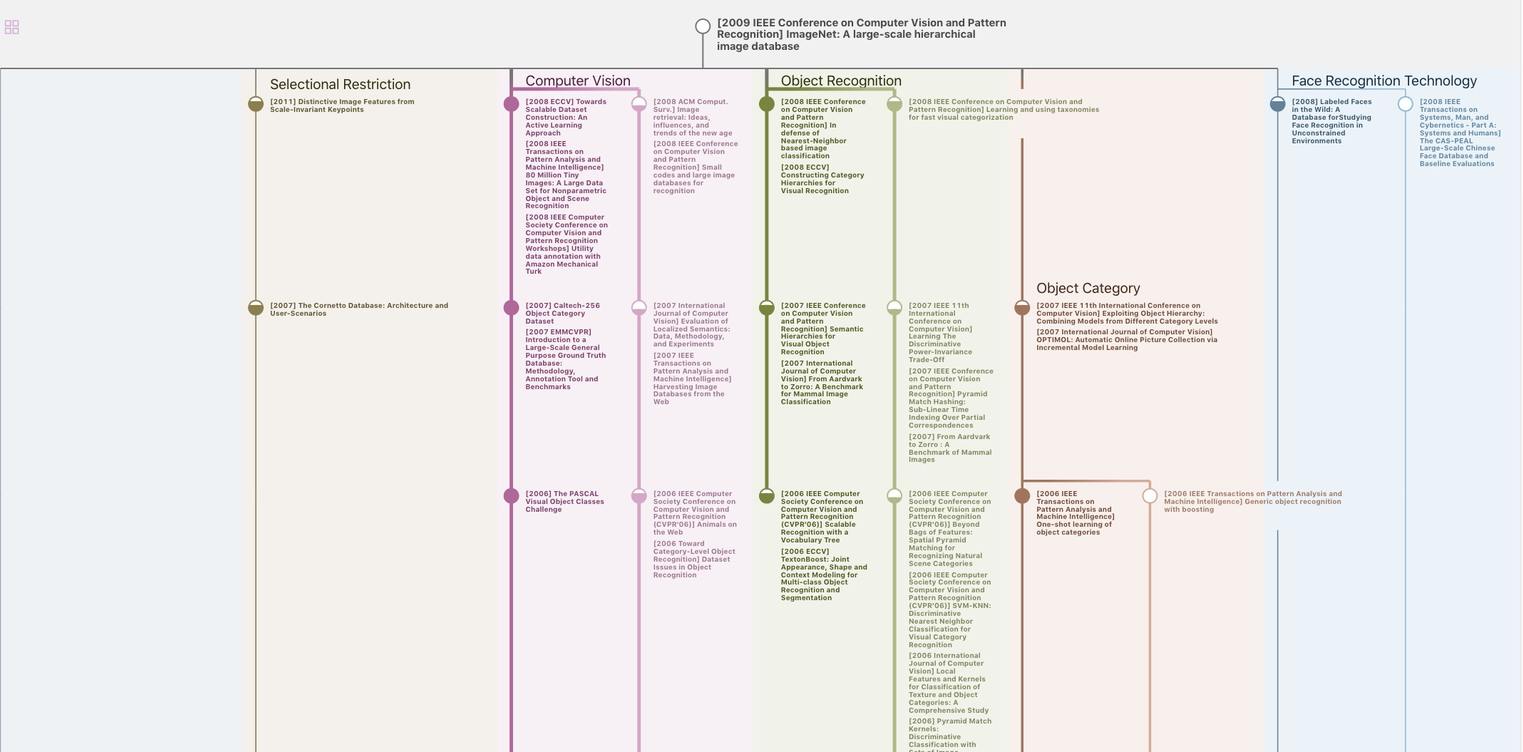
生成溯源树,研究论文发展脉络
Chat Paper
正在生成论文摘要