A Self-Supervised Learning Solution With Momentum-Based Siamese Network for Robotic Visual Task
IEEE Access(2023)
摘要
How a robot induces causality, abstracts information from states, and plans for tasks are major challenges in artificial intelligence. However, habitually used deep reinforcement learning is not a cure-all solution. This study proposes MoSN, a momentum-based Siamese network for a robotic visual task. Instead of trying to learn representations that abstract all the variation factors of the environment as in most prior studies, MoSN labels its observations as their causes so that it can learn representations of visual states in a self-supervised manner. These representations are then used to enhance perceptual and cognitive abilities and to perform our robotic visual task when a robot takes over a new environment. In particular, this study proposes a momentum-based Siamese network with augmentation methods and a hybrid explicit contrastive loss to improve the combinatorial generalization capability of our model. We validate MoSN in real-world environments equipped with lights and light switches and attempt to derive the cause-and-effect relationship between switch states and visual states. MoSN is compared with previous methods in both static and dynamic environments with both fixed and dynamic viewing angles. MoSN outperforms previous methods in most cases.
更多查看译文
关键词
Artificial intelligence,causality,cause-and-effect,momentum-based Siamese network,self-supervised
AI 理解论文
溯源树
样例
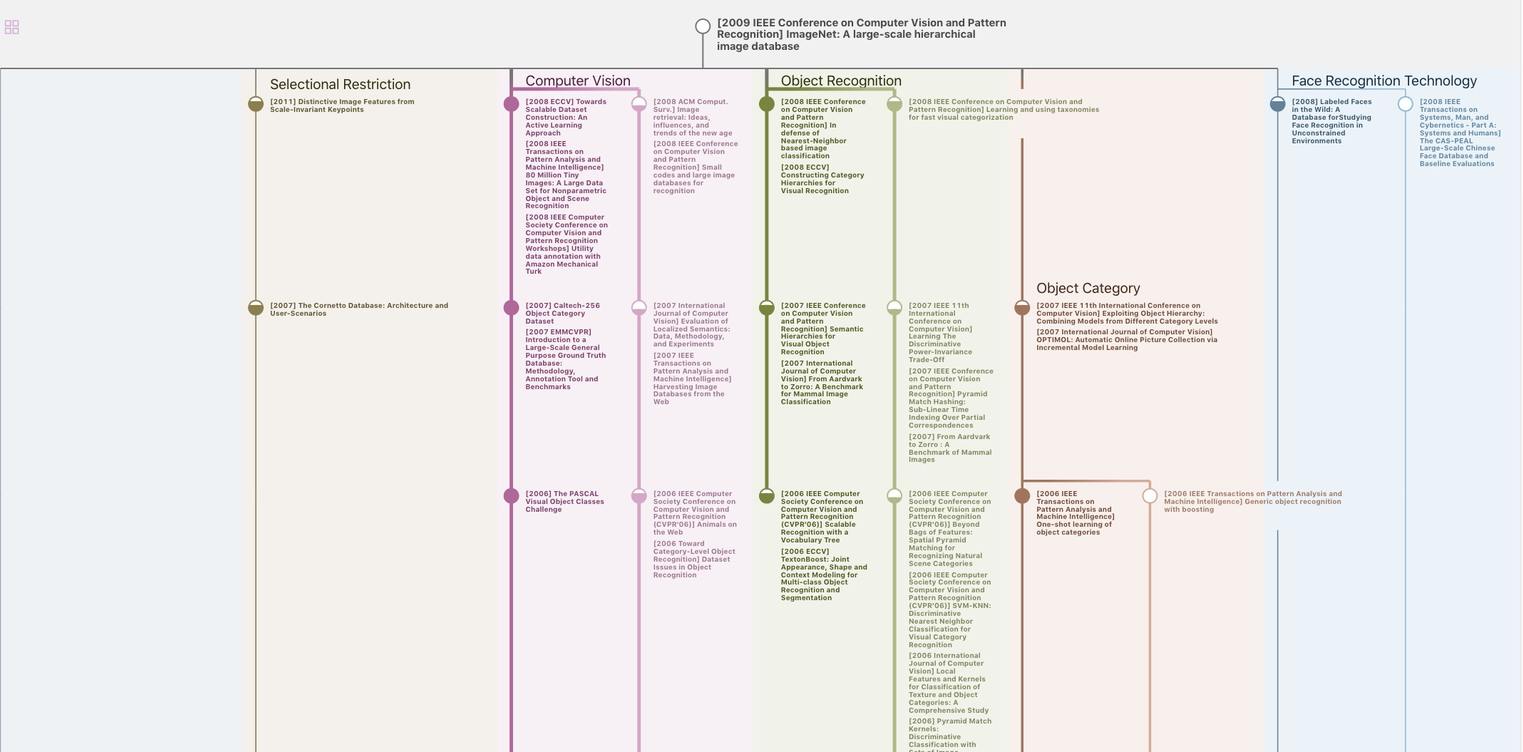
生成溯源树,研究论文发展脉络
Chat Paper
正在生成论文摘要