Examinations of Biases by Model Misspecification and Parameter Reliability of Reinforcement Learning Models
Computational Brain & Behavior(2023)
摘要
Reinforcement learning models have the potential to clarify meaningful individual differences in the decision-making process. This study focused on two aspects regarding the nature of a reinforcement learning model and its parameters: the problems of model misspecification and reliability. Online participants, N = 453, completed self-report measures and a probabilistic learning task twice 1.5 months apart, and data from the task were fitted using several reinforcement learning models. To address the problem of model misspecification, we compared the models with and without the influence of choice history, or perseveration. Results showed that the lack of a perseveration term in the model led to a decrease in learning rates for win and loss outcomes, with slightly different influences depending on outcome volatility, and increases in inverse temperature. We also conducted simulations to examine the mechanism of the observed biases and revealed that failure to incorporate perseveration directly affected the estimation bias in the learning rate and indirectly affected that in inverse temperature. Furthermore, in both model fittings and model simulations, the lack of perseveration caused win-stay probability underestimation and loss-shift probability overestimation. We also assessed the parameter reliability. Test–retest reliabilities were poor (learning rates) to moderate (inverse temperature and perseveration magnitude). A learning effect was noted in the inverse temperature and perseveration magnitude parameters, showing an increment of the estimates in the second session. We discuss possible misinterpretations of results and limitations considering the estimation biases and parameter reliability.
更多查看译文
关键词
Reinforcement learning model,Model misspecification,Choice history,Perseveration,Parameter estimation bias,Parameter reliability
AI 理解论文
溯源树
样例
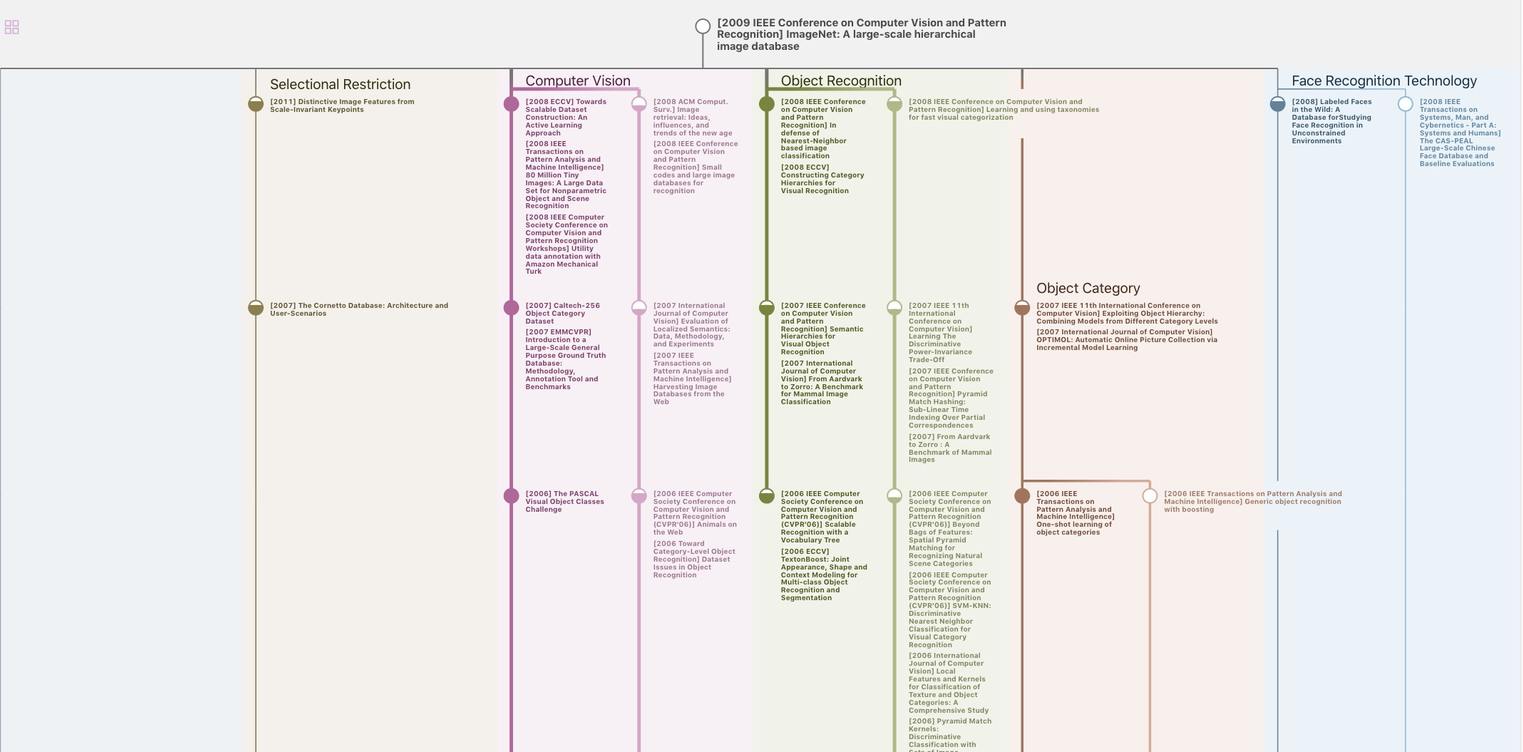
生成溯源树,研究论文发展脉络
Chat Paper
正在生成论文摘要