ActioNet: A Lightweight Architecture for Efficient Action Recognition
VRST '23: Proceedings of the 29th ACM Symposium on Virtual Reality Software and Technology(2023)
摘要
We present ActioNet, a groundbreaking lightweight neural network architecture optimized for action recognition tasks, particularly in resource-constrained environments such as drones and edge devices. Utilizing a strategically modified 3D U-Net encoder followed by fully connected layers for fine-grained classification, ActioNet manages to achieve a promising validation accuracy of 72%. This is accomplished with a notably compact model size of just 46MB, making it uniquely suitable for devices with limited computational capabilities. Although ActioNet may not surpass state-of-the-art models in terms of sheer accuracy, it distinguishes itself through its fast inference times and small footprint. These attributes make real-time action recognition not only feasible but also efficient in constrained operational settings. We argue that ActioNet serves as a meaningful contribution to the emerging field of efficient deep learning and provides a solid foundation for future advancements in lightweight action recognition models.
更多查看译文
关键词
Action recognition,lightweight architecture,drones,convolutional neural networks,3D convolutions,real-time processing
AI 理解论文
溯源树
样例
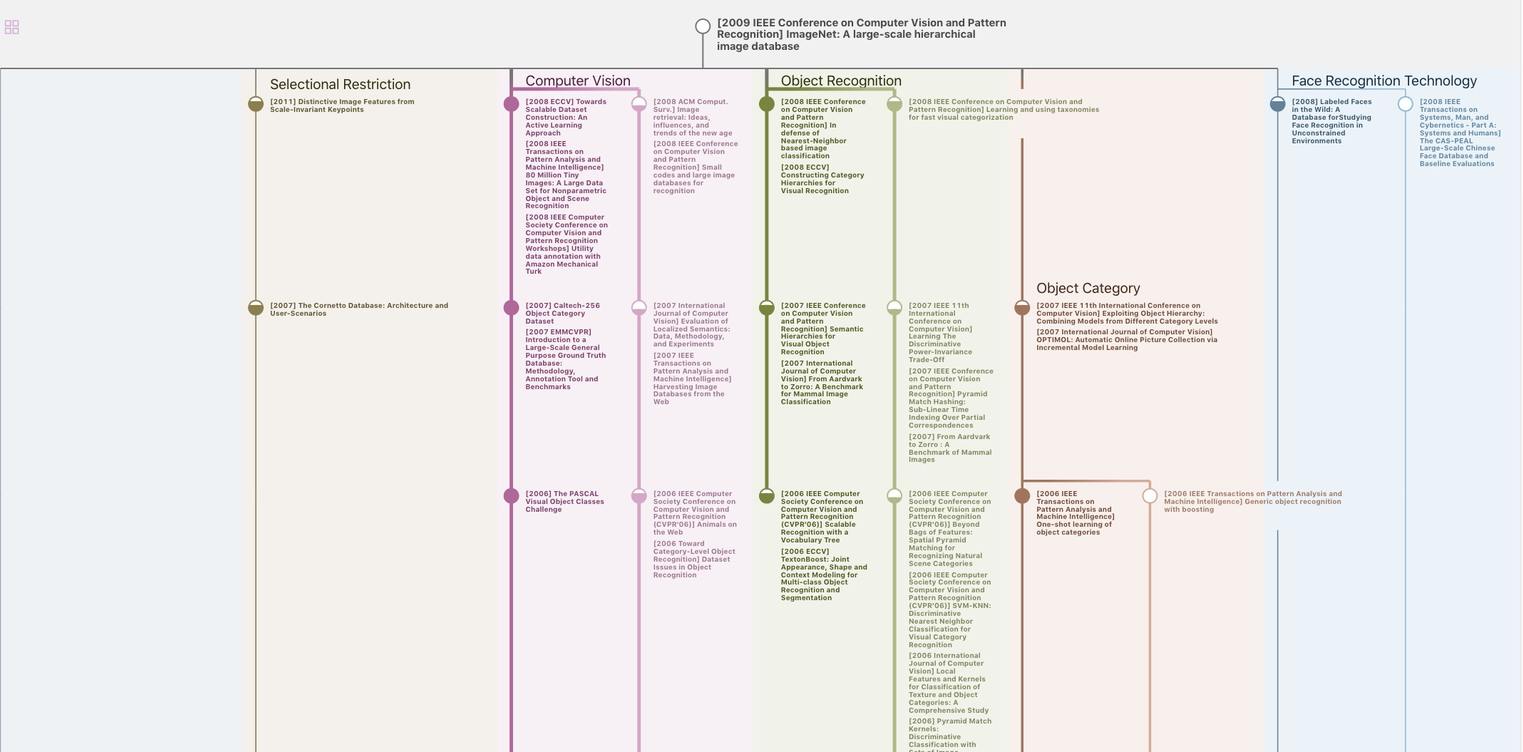
生成溯源树,研究论文发展脉络
Chat Paper
正在生成论文摘要