Quality-Guaranteed and Cost-Effective Population Health Profiling: A Deep Active Learning Approach.
ACM Transactions on Computing for Healthcare(2023)
摘要
Reliability and cost are two primary considerations for profiling population-scale prevalence ( PPP ) of multiple non-communicable diseases ( NCDs ). In this paper, we exploit intra-disease and inter-disease correlation in different traditionally-sensed-areas ( TS-A ) to reduce the number of profiling tasks required without compromising data reliability. Specifically, we propose a novel approach called Compressive Population Health TS-A Selection ( CPH-TS ), which blends the state-of-the-art profile inference, data augmentation and active learning in a unified deep learning framework. It can actively select the minimum number of TS-A regions for profiling task allocation in each profiling cycle, while deducing the missing data on the unprofiled regions with a probabilistic guarantee of reliability. We evaluate our approach on real-world prevalence datasets of London, which shows the effectiveness of CPH-TS . In general, CPH-TS assigned 11.1-27.3% fewer tasks than baselines, assigning tasks to only 34.7% of the sub-regions while the profiling error was below 5% for 95% of the cycles.
更多查看译文
关键词
population health,deep,quality-guaranteed,cost-effective
AI 理解论文
溯源树
样例
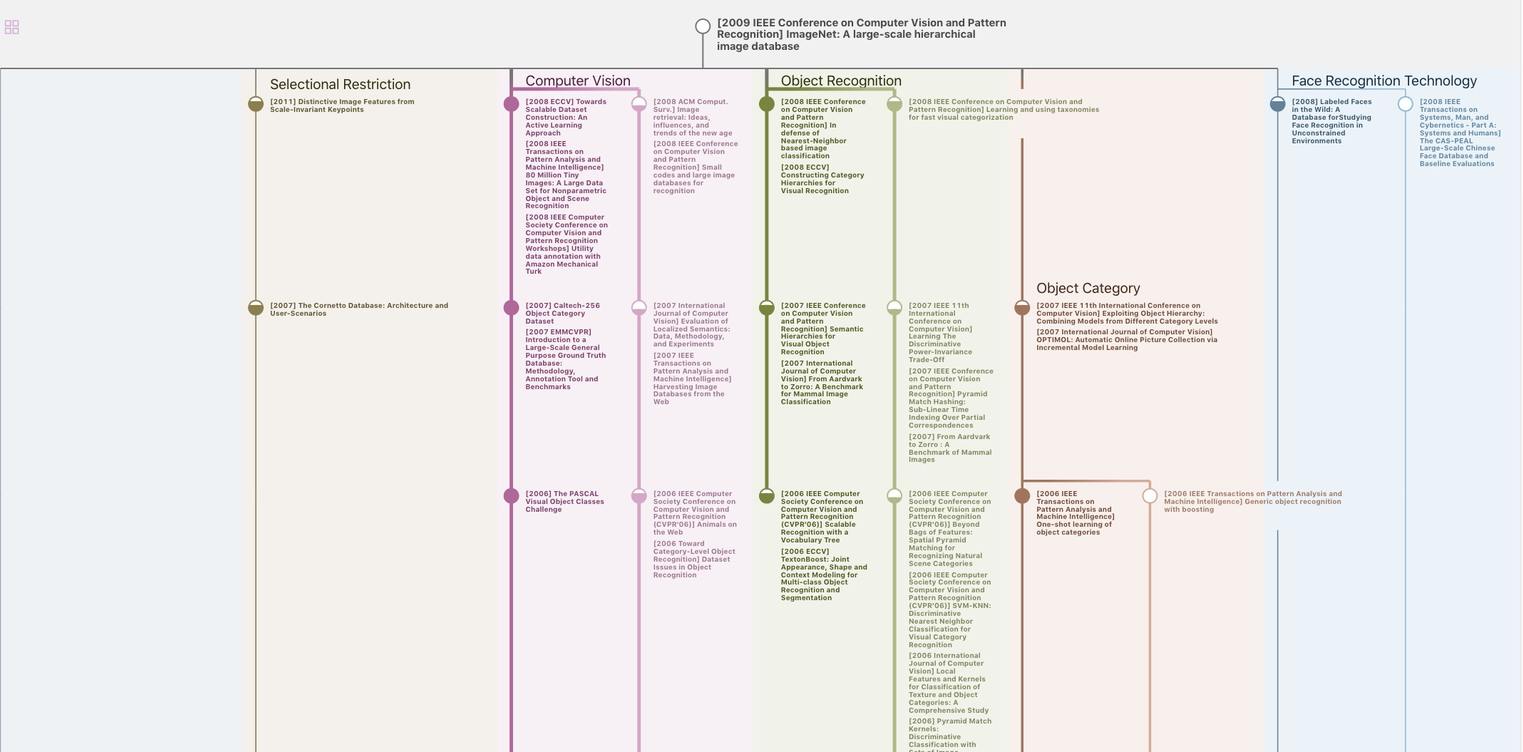
生成溯源树,研究论文发展脉络
Chat Paper
正在生成论文摘要