Alleviating Training Bias with Less Cost via Multi-expert De-biasing Method in Scene Graph Generation
PROCEEDINGS OF THE 1ST INTERNATIONAL WORKSHOP ON MULTIMEDIA CONTENT GENERATION AND EVALUATION, MCGE 2023: New Methods and Practice(2023)
摘要
Scene graph generation (SGG) methods have suffered from a severe training bias towards frequent (head) predicate classes. Recent works owe it to the long-tailed distribution of predicates and alleviate the long-tailed problem to conduct de-biasing. However, the "unbiased" models are in turn biased to tail predicate classes, resulting in a significant performance loss on head predicate classes. The main cause of such a trade-off between head and tail predicates is the fact that multiple predicates from the head or tail ones can be labeled as the ground-truth. To this end, we propose a multi-expert de-biasing method (MED) for SGG that can produce unbiased scene graphs with minor influence on recognizing head predicates. We avoid the dilemma of balancing between head and tail predicates by adaptively classifying the predicates with multiple complementary models. Experiments on the Visual Genome dataset show that MED provides significant gains on mRecall@K without harming the performance on Recall@K, and achieves a state-of-the-art on the mean of Recall@K and mRecall@K.
更多查看译文
关键词
Scene graph generation,long-tailed distribution,multi-expert network,de-biasing
AI 理解论文
溯源树
样例
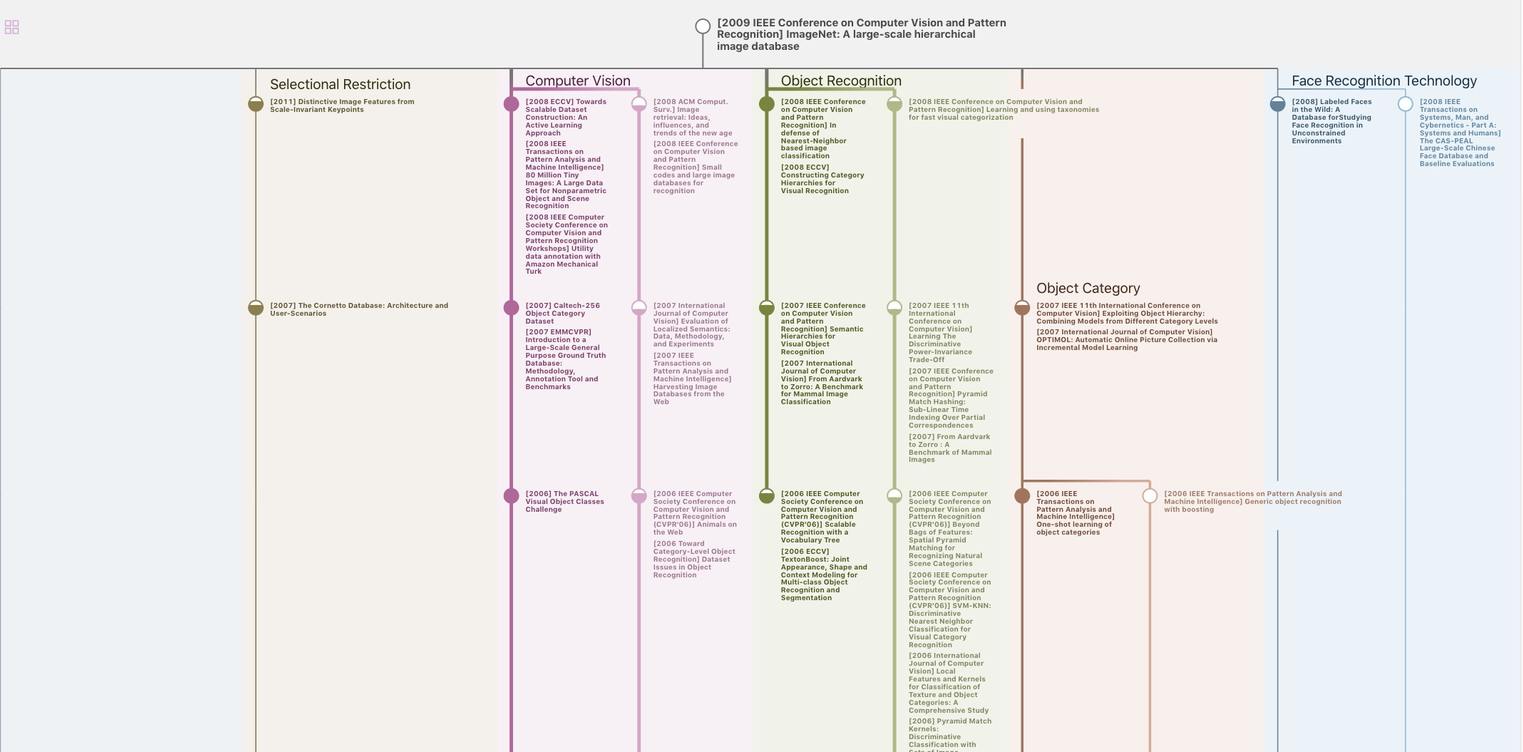
生成溯源树,研究论文发展脉络
Chat Paper
正在生成论文摘要