On the use of Vision-Language models for Visual Sentiment Analysis: a study on CLIP
2023 11TH INTERNATIONAL CONFERENCE ON AFFECTIVE COMPUTING AND INTELLIGENT INTERACTION, ACII(2023)
摘要
This work presents a study on how to exploit the CLIP embedding space to perform Visual Sentiment Analysis. We experiment with two architectures built on top of the CLIP embedding space, which we denote by CLIP-E. We train the CLIP-E models with WEBEmo, the largest publicly available and manually labeled benchmark for Visual Sentiment Analysis, and perform two sets of experiments. First, we test on WEBEmo and compare the CLIP-E architectures with state-of-the-art (SOTA) models and with CLIP Zero-Shot. Second, we perform cross dataset evaluation, and test the CLIP-E architectures trained with WEBEmo on other Visual Sentiment Analysis benchmarks. Our results show that the CLIP-E approaches outperform SOTA models in WEBEmo fine grained categorization, and they also generalize better when tested on datasets that have not been seen during training. Interestingly, we observed that for the FI dataset, CLIP Zero-Shot produces better accuracies than SOTA models and CLIP-E trained on WEBEmo. These results motivate several questions that we discuss in this paper, such as how we should design new benchmarks and evaluate Visual Sentiment Analysis, and whether we should keep designing tailored Deep Learning models for Visual Sentiment Analysis or focus our efforts on better using the knowledge encoded in large vision-language models such as CLIP for this task. Our code is available at https://github.com/cristinabustos16/CLIP-E.
更多查看译文
关键词
visual sentiment analysis,vision-language models,zero-shot classification
AI 理解论文
溯源树
样例
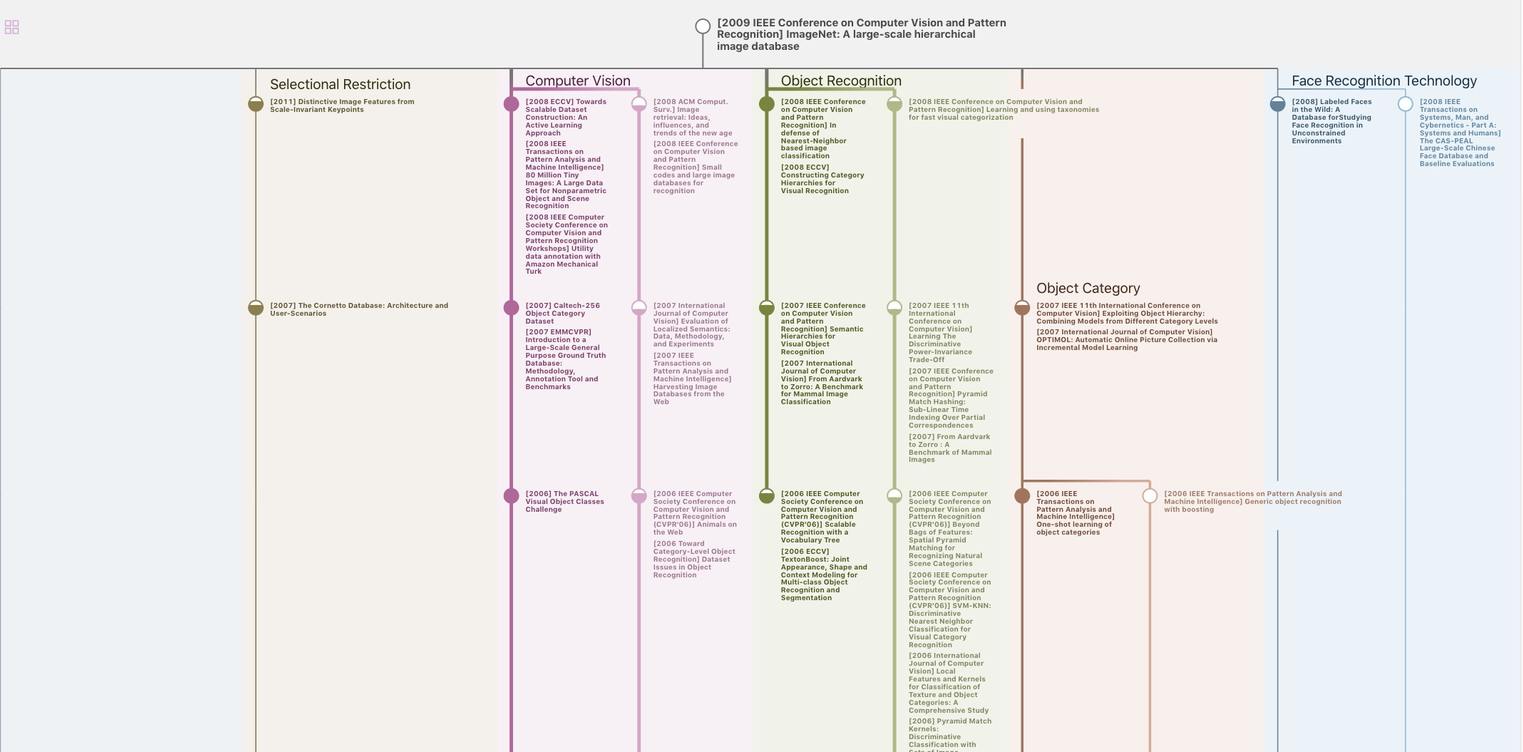
生成溯源树,研究论文发展脉络
Chat Paper
正在生成论文摘要