Multi-view Contrastive Learning for Entity Typing over Knowledge Graphs.
CoRR(2023)
摘要
Knowledge graph entity typing (KGET) aims at inferring plausible types of entities in knowledge graphs. Existing approaches to KGET focus on how to better encode the knowledge provided by the neighbors and types of an entity into its representation. However, they ignore the semantic knowledge provided by the way in which types can be clustered together. In this paper, we propose a novel method called Multi-view Contrastive Learning for knowledge graph Entity Typing (MCLET), which effectively encodes the coarse-grained knowledge provided by clusters into entity and type embeddings. MCLET is composed of three modules: i) Multi-view Generation and Encoder module, which encodes structured information from entity-type, entity-cluster and cluster-type views; ii) Cross-view Contrastive Learning module, which encourages different views to collaboratively improve view-specific representations of entities and types; iii) Entity Typing Prediction module, which integrates multi-head attention and a Mixture-of-Experts strategy to infer missing entity types. Extensive experiments show the strong performance of MCLET compared to the state-of-the-art
更多查看译文
关键词
entity typing,knowledge,multi-view
AI 理解论文
溯源树
样例
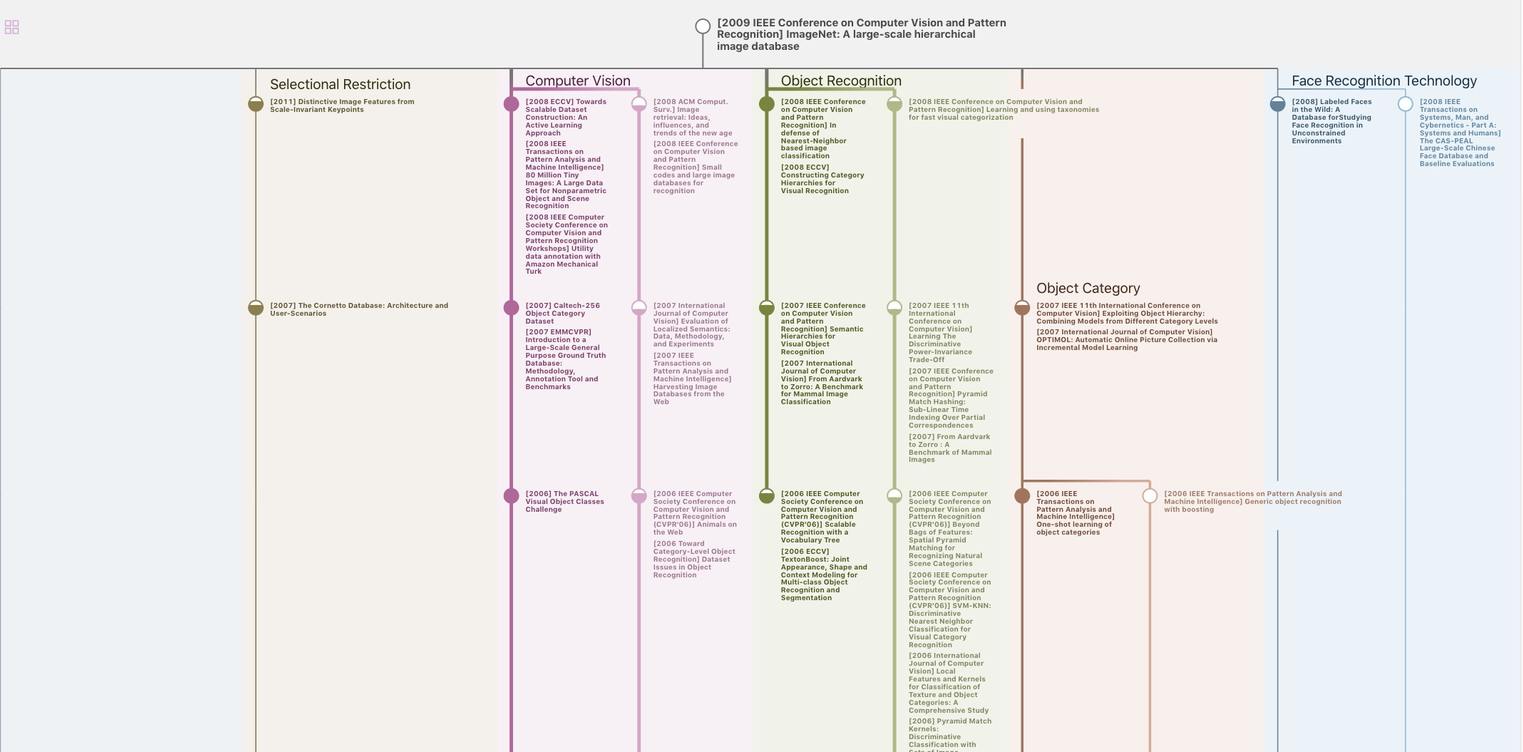
生成溯源树,研究论文发展脉络
Chat Paper
正在生成论文摘要