SQ Lower Bounds for Learning Mixtures of Linear Classifiers
NeurIPS(2023)
摘要
We study the problem of learning mixtures of linear classifiers under Gaussian covariates. Given sample access to a mixture of $r$ distributions on $\mathbb{R}^n$ of the form $(\mathbf{x},y_{\ell})$, $\ell\in [r]$, where $\mathbf{x}\sim\mathcal{N}(0,\mathbf{I}_n)$ and $y_\ell=\mathrm{sign}(\langle\mathbf{v}_\ell,\mathbf{x}\rangle)$ for an unknown unit vector $\mathbf{v}_\ell$, the goal is to learn the underlying distribution in total variation distance. Our main result is a Statistical Query (SQ) lower bound suggesting that known algorithms for this problem are essentially best possible, even for the special case of uniform mixtures. In particular, we show that the complexity of any SQ algorithm for the problem is $n^{\mathrm{poly}(1/\Delta) \log(r)}$, where $\Delta$ is a lower bound on the pairwise $\ell_2$-separation between the $\mathbf{v}_\ell$'s. The key technical ingredient underlying our result is a new construction of spherical designs that may be of independent interest.
更多查看译文
关键词
learning mixtures,lower bounds
AI 理解论文
溯源树
样例
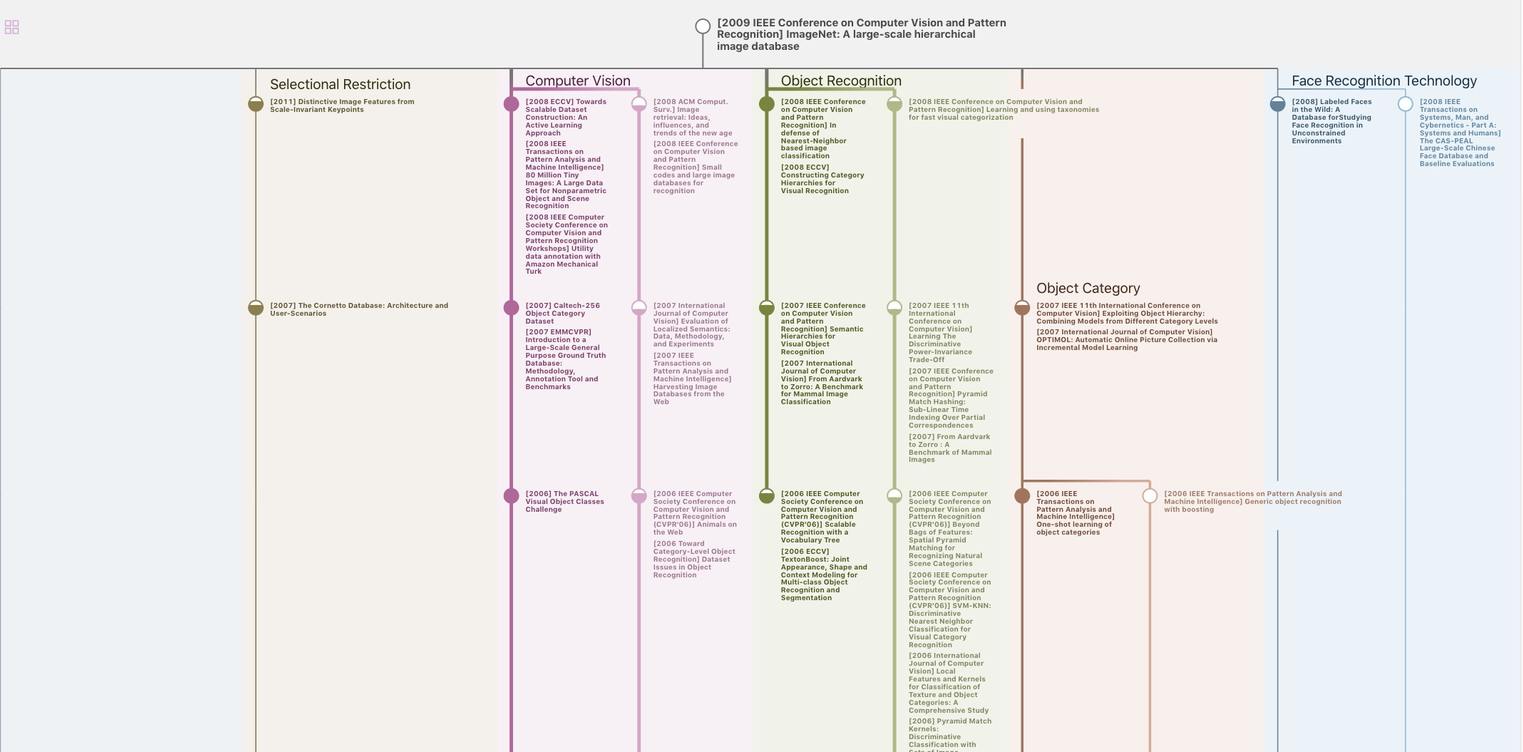
生成溯源树,研究论文发展脉络
Chat Paper
正在生成论文摘要