Personalized Soups: Personalized Large Language Model Alignment via Post-hoc Parameter Merging
CoRR(2023)
摘要
While Reinforcement Learning from Human Feedback (RLHF) aligns Large Language Models (LLMs) with general, aggregate human preferences, it is suboptimal for learning diverse, individual perspectives. In this work, we study Reinforcement Learning from Personalized Human Feedback (RLPHF) problem, wherein LLMs are aligned to multiple (sometimes conflicting) preferences by modeling alignment as a Multi-Objective Reinforcement Learning (MORL) problem. Compared to strong single-objective baselines, we show that we can achieve personalized alignment by decomposing preferences into multiple dimensions. These dimensions are defined based on personalizations that are declared as desirable by the user. In this work, we show that they can be efficiently trained independently in a distributed manner and combined effectively post-hoc through parameter merging. The code is available at https://github.com/joeljang/RLPHF.
更多查看译文
关键词
large language model alignment,personalized,post-hoc
AI 理解论文
溯源树
样例
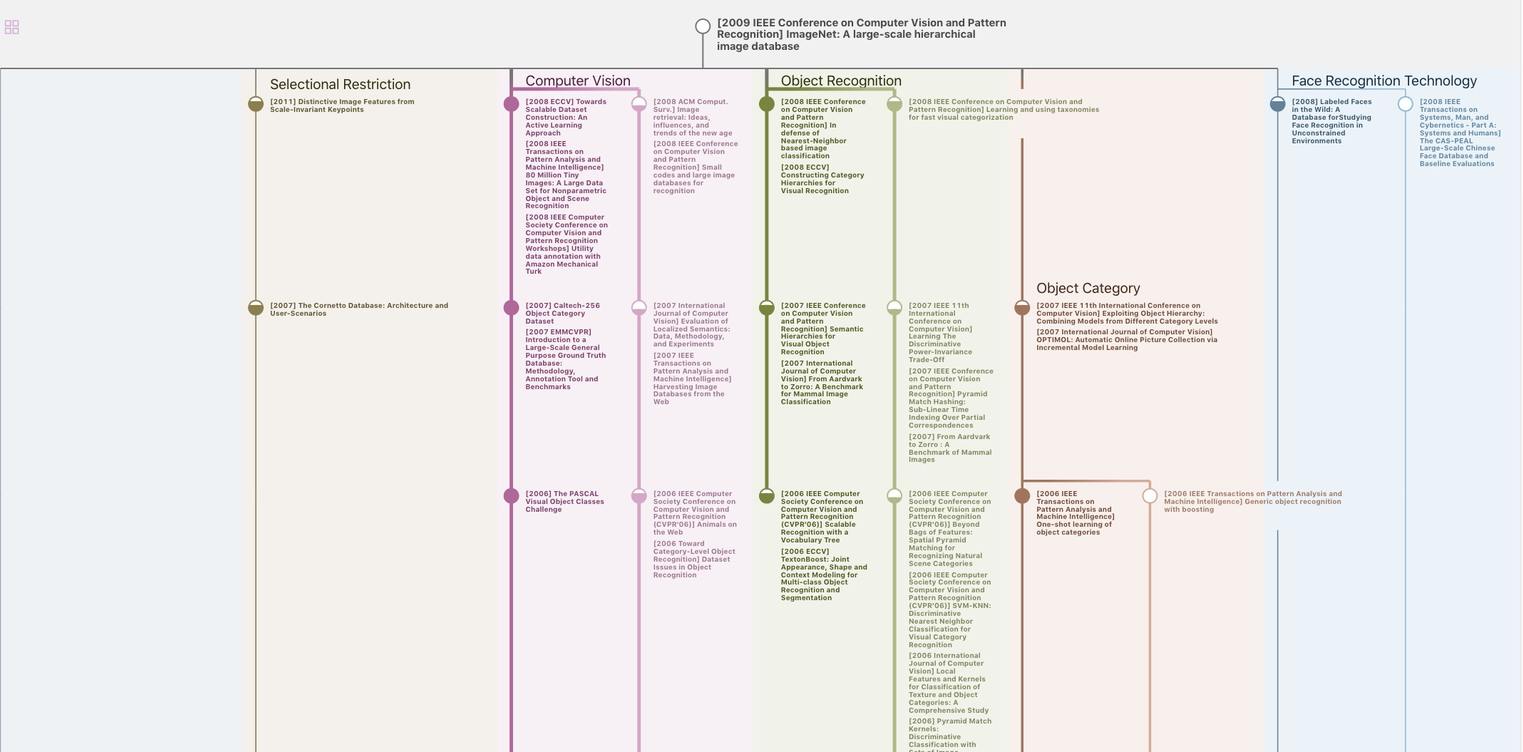
生成溯源树,研究论文发展脉络
Chat Paper
正在生成论文摘要