Multi-stage Large Language Model Correction for Speech Recognition
CoRR(2023)
摘要
In this paper, we investigate the usage of large language models (LLMs) to improve the performance of competitive speech recognition systems. Different from traditional language models that focus on one single data domain, the rise of LLMs brings us the opportunity to push the limit of state-of-the-art ASR performance, and at the same time to achieve higher robustness and generalize effectively across multiple domains. Motivated by this, we propose a novel multi-stage approach to combine traditional language model re-scoring and LLM prompting. Specifically, the proposed method has two stages: the first stage uses a language model to re-score an N-best list of ASR hypotheses and run a confidence check; The second stage uses prompts to a LLM to perform ASR error correction on less confident results from the first stage. Our experimental results demonstrate the effectiveness of the proposed method by showing a 10% ~ 20% relative improvement in WER over a competitive ASR system -- across multiple test domains.
更多查看译文
关键词
large language model,speech recognition,large language,multi-stage
AI 理解论文
溯源树
样例
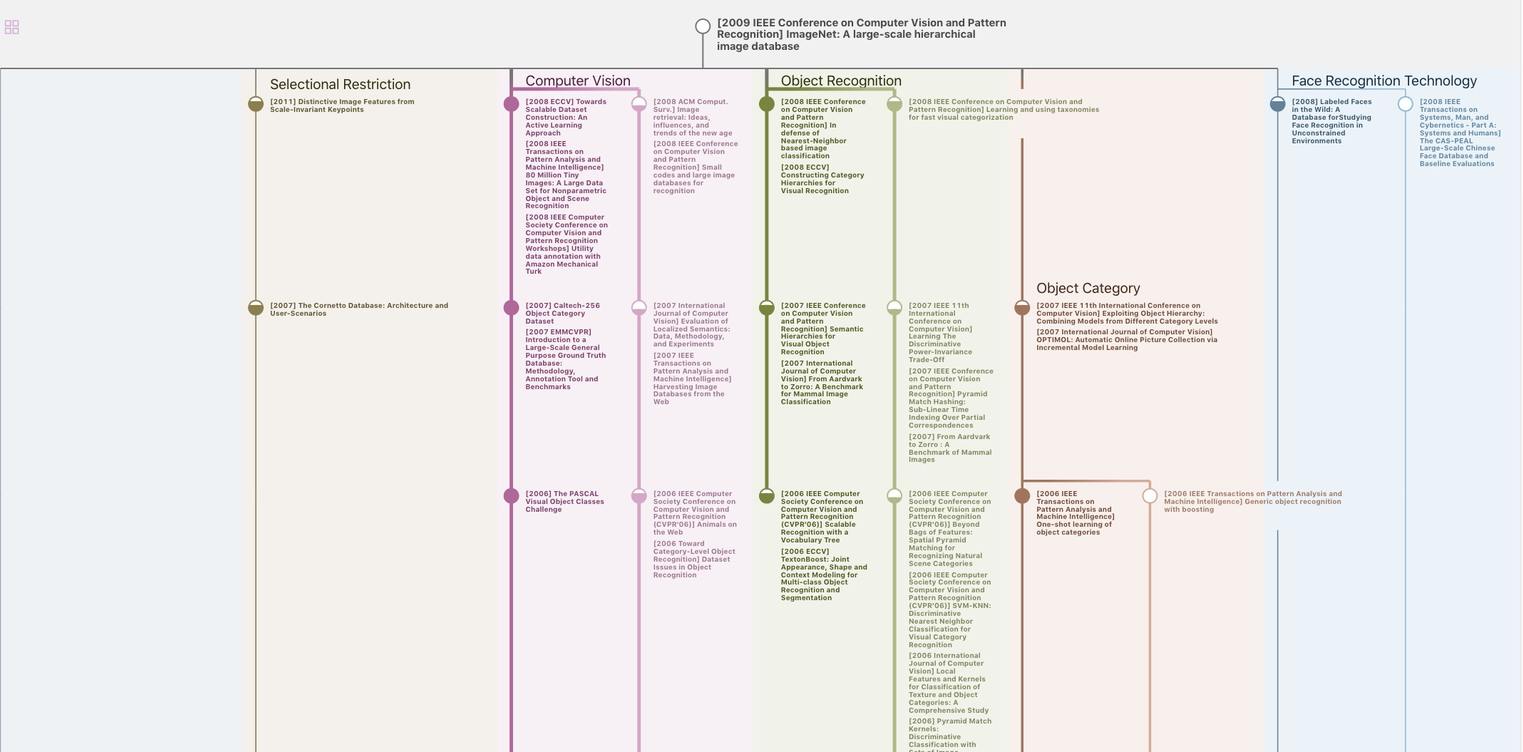
生成溯源树,研究论文发展脉络
Chat Paper
正在生成论文摘要