Goodness-of-fit testing for meta-analysis of rare binary events.
Scientific reports(2023)
摘要
Random-effects (RE) meta-analysis is a crucial approach for combining results from multiple independent studies that exhibit heterogeneity. Recently, two frequentist goodness-of-fit (GOF) tests were proposed to assess the fit of RE model. However, they tend to perform poorly when assessing rare binary events. Under a general binomial-normal framework, we propose a novel GOF test for the meta-analysis of rare events. Our method is based on pivotal quantities that play an important role in Bayesian model assessment. It further adopts the Cauchy combination idea proposed in a 2019 JASA paper, to combine dependent p-values computed using posterior samples from Markov Chain Monte Carlo. The advantages of our method include clear conception and interpretation, incorporation of all data including double zeros without the need for artificial correction, well-controlled Type I error, and generally improved ability in detecting model misfits compared to previous GOF methods. We illustrate the proposed method via simulation and three real data applications.
更多查看译文
关键词
rare,testing,goodness-of-fit,meta-analysis
AI 理解论文
溯源树
样例
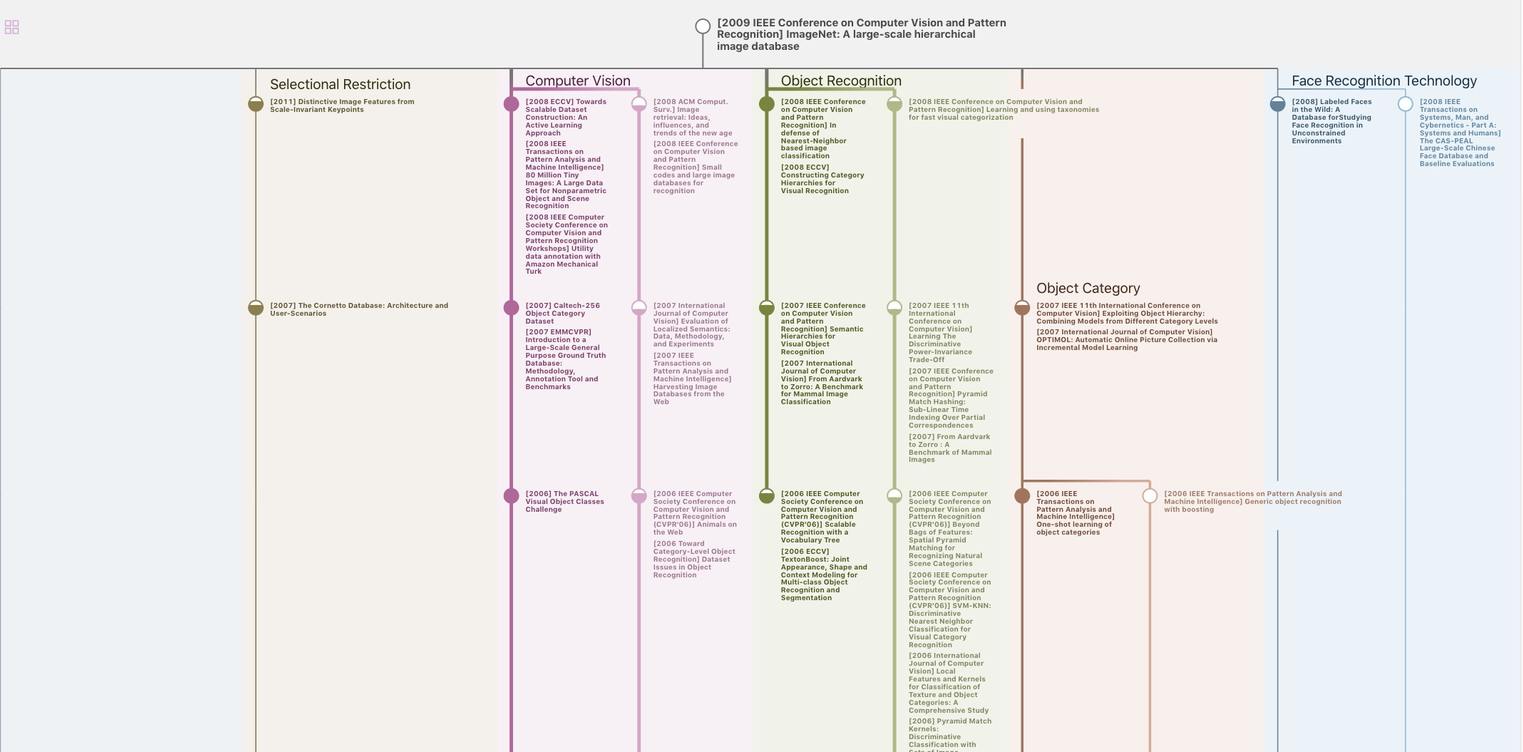
生成溯源树,研究论文发展脉络
Chat Paper
正在生成论文摘要