Functional Invariants to Watermark Large Transformers
ICASSP 2024 - 2024 IEEE International Conference on Acoustics, Speech and Signal Processing (ICASSP)(2023)
摘要
The rapid growth of transformer-based models increases the concerns about
their integrity and ownership insurance. Watermarking addresses this issue by
embedding a unique identifier into the model, while preserving its performance.
However, most existing approaches require to optimize the weights to imprint
the watermark signal, which is not suitable at scale due to the computational
cost. This paper explores watermarks with virtually no computational cost,
applicable to a non-blind white-box setting (assuming access to both the
original and watermarked networks). They generate functionally equivalent
copies by leveraging the models' invariance, via operations like dimension
permutations or scaling/unscaling. This enables to watermark models without any
change in their outputs and remains stealthy. Experiments demonstrate the
effectiveness of the approach and its robustness against various model
transformations (fine-tuning, quantization, pruning), making it a practical
solution to protect the integrity of large models.
更多查看译文
关键词
DNN watermarking,white-box,transformers
AI 理解论文
溯源树
样例
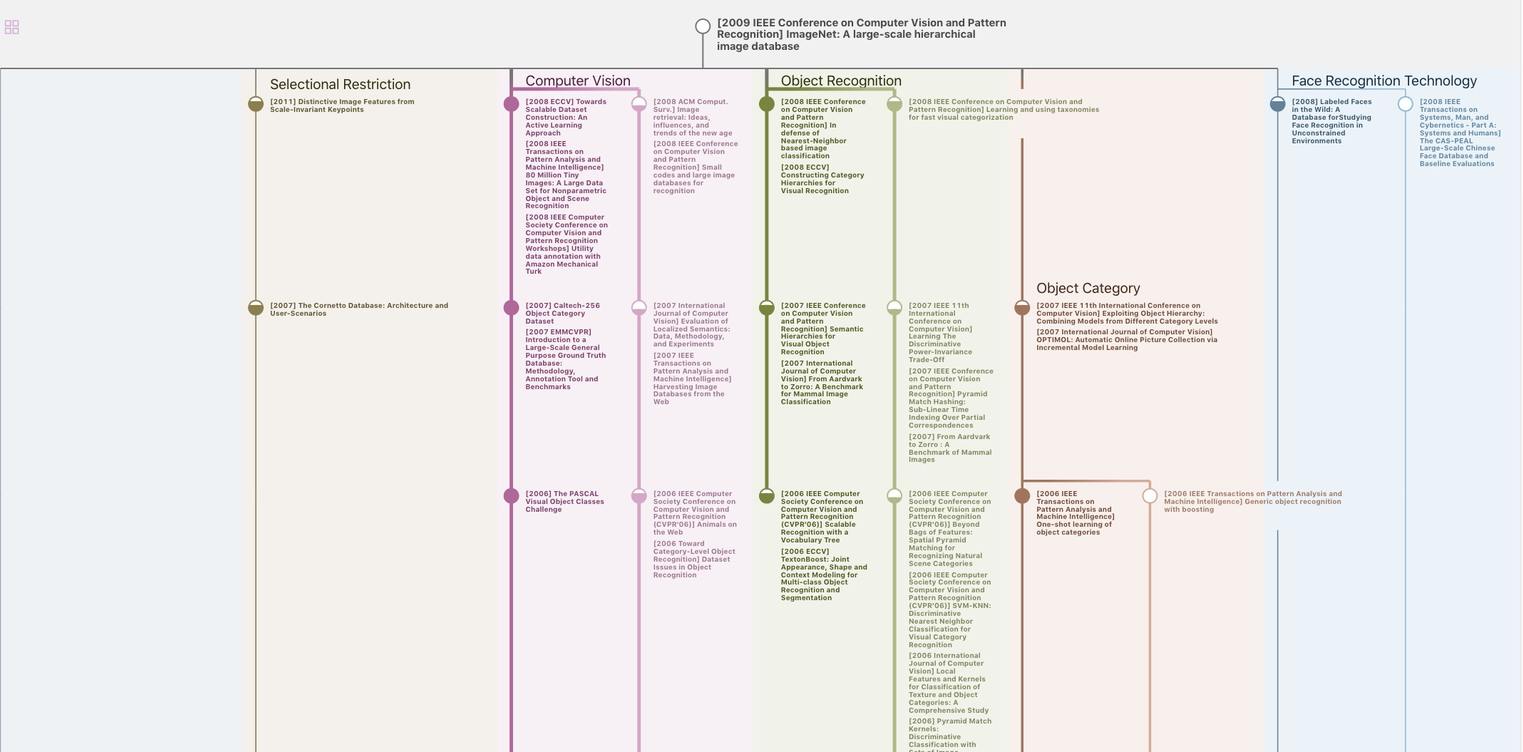
生成溯源树,研究论文发展脉络
Chat Paper
正在生成论文摘要