Learning to Sample Better
CoRR(2023)
摘要
These lecture notes provide an introduction to recent advances in generative modeling methods based on the dynamical transportation of measures, by means of which samples from a simple base measure are mapped to samples from a target measure of interest. Special emphasis is put on the applications of these methods to Monte-Carlo (MC) sampling techniques, such as importance sampling and Markov Chain Monte-Carlo (MCMC) schemes. In this context, it is shown how the maps can be learned variationally using data generated by MC sampling, and how they can in turn be used to improve such sampling in a positive feedback loop.
更多查看译文
关键词
sample,learning
AI 理解论文
溯源树
样例
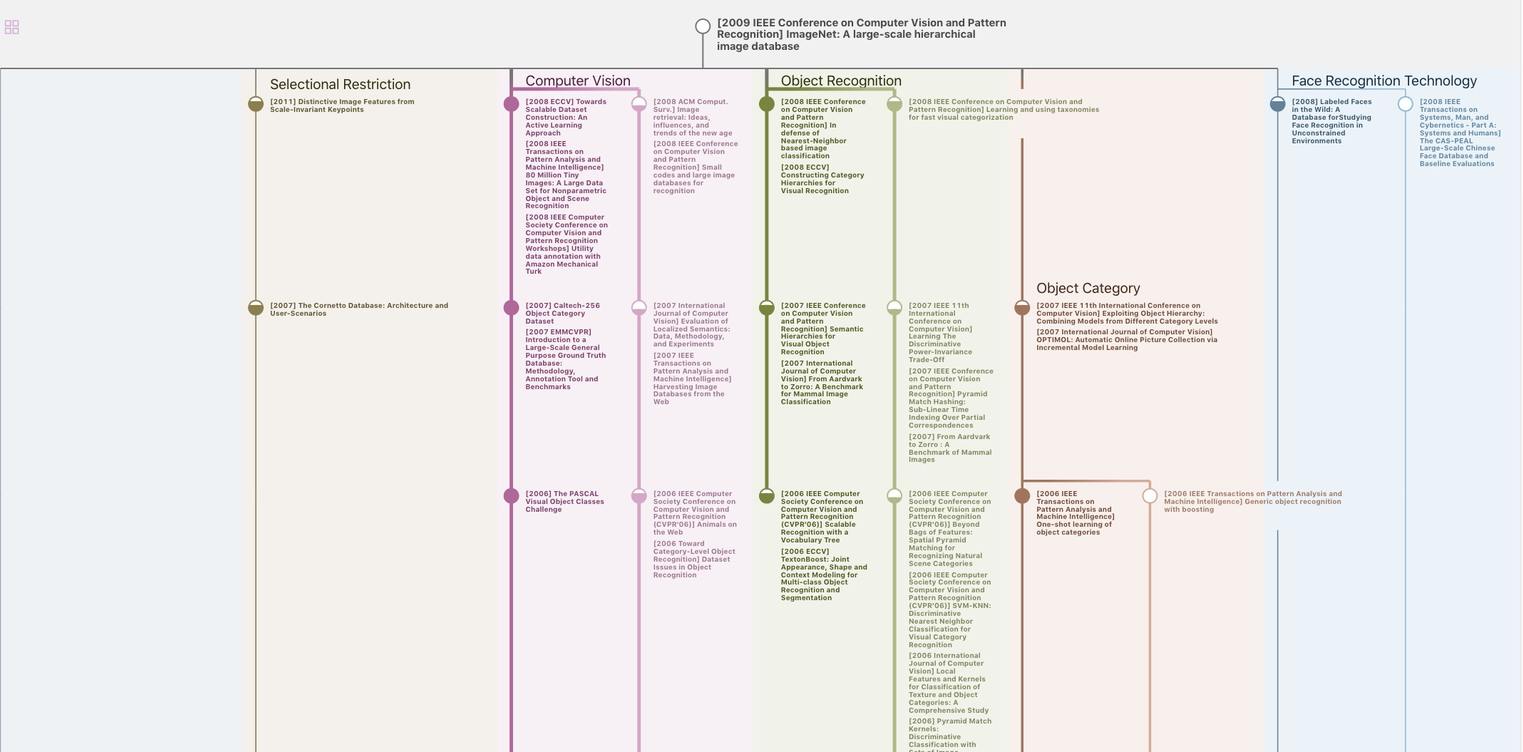
生成溯源树,研究论文发展脉络
Chat Paper
正在生成论文摘要