Causal discovery using dynamically requested knowledge
CoRR(2023)
摘要
Causal Bayesian Networks (CBNs) are an important tool for reasoning under uncertainty in complex real-world systems. Determining the graphical structure of a CBN remains a key challenge and is undertaken either by eliciting it from humans, using machine learning to learn it from data, or using a combination of these two approaches. In the latter case, human knowledge is generally provided to the algorithm before it starts, but here we investigate a novel approach where the structure learning algorithm itself dynamically identifies and requests knowledge for relationships that the algorithm identifies as uncertain during structure learning. We integrate this approach into the Tabu structure learning algorithm and show that it offers considerable gains in structural accuracy, which are generally larger than those offered by existing approaches for integrating knowledge. We suggest that a variant which requests only arc orientation information may be particularly useful where the practitioner has little preexisting knowledge of the causal relationships. As well as offering improved accuracy, the approach can use human expertise more effectively and contributes to making the structure learning process more transparent.
更多查看译文
关键词
causal discovery,knowledge
AI 理解论文
溯源树
样例
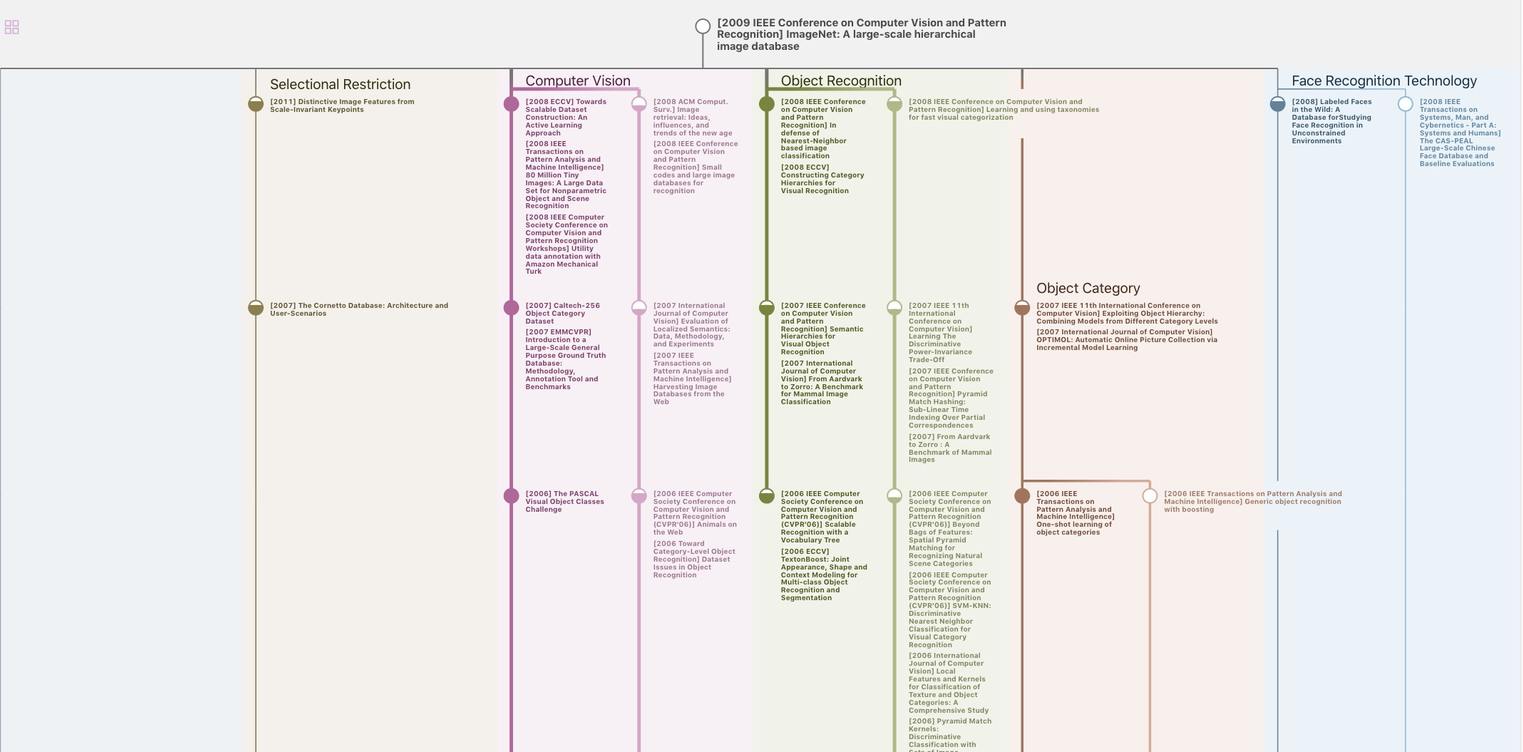
生成溯源树,研究论文发展脉络
Chat Paper
正在生成论文摘要