Locally Differentially Private Graph Embedding
CoRR(2023)
摘要
Graph embedding has been demonstrated to be a powerful tool for learning latent representations for nodes in a graph. However, despite its superior performance in various graph-based machine learning tasks, learning over graphs can raise significant privacy concerns when graph data involves sensitive information. To address this, in this paper, we investigate the problem of developing graph embedding algorithms that satisfy local differential privacy (LDP). We propose LDP-GE, a novel privacy-preserving graph embedding framework, to protect the privacy of node data. Specifically, we propose an LDP mechanism to obfuscate node data and adopt personalized PageRank as the proximity measure to learn node representations. Then, we theoretically analyze the privacy guarantees and utility of the LDP-GE framework. Extensive experiments conducted over several real-world graph datasets demonstrate that LDP-GE achieves favorable privacy-utility trade-offs and significantly outperforms existing approaches in both node classification and link prediction tasks.
更多查看译文
关键词
private graph
AI 理解论文
溯源树
样例
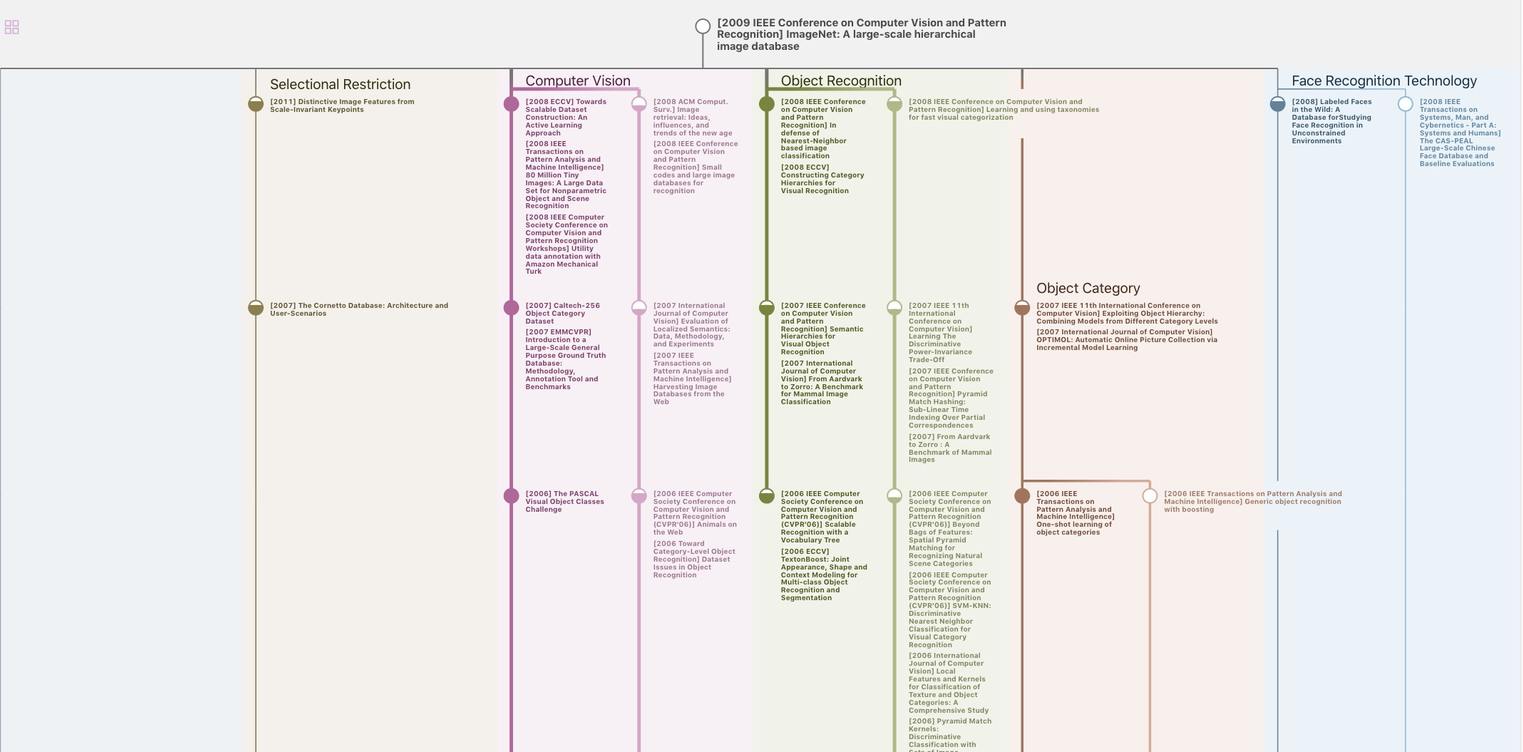
生成溯源树,研究论文发展脉络
Chat Paper
正在生成论文摘要