Accurate Data-Driven Surrogates of Dynamical Systems for Forward Propagation of Uncertainty
CoRR(2023)
摘要
Stochastic collocation (SC) is a well-known non-intrusive method of constructing surrogate models for uncertainty quantification. In dynamical systems, SC is especially suited for full-field uncertainty propagation that characterizes the distributions of the high-dimensional primary solution fields of a model with stochastic input parameters. However, due to the highly nonlinear nature of the parameter-to-solution map in even the simplest dynamical systems, the constructed SC surrogates are often inaccurate. This work presents an alternative approach, where we apply the SC approximation over the dynamics of the model, rather than the solution. By combining the data-driven sparse identification of nonlinear dynamics (SINDy) framework with SC, we construct dynamics surrogates and integrate them through time to construct the surrogate solutions. We demonstrate that the SC-over-dynamics framework leads to smaller errors, both in terms of the approximated system trajectories as well as the model state distributions, when compared against full-field SC applied to the solutions directly. We present numerical evidence of this improvement using three test problems: a chaotic ordinary differential equation, and two partial differential equations from solid mechanics.
更多查看译文
关键词
dynamical systems,uncertainty,propagation,data-driven
AI 理解论文
溯源树
样例
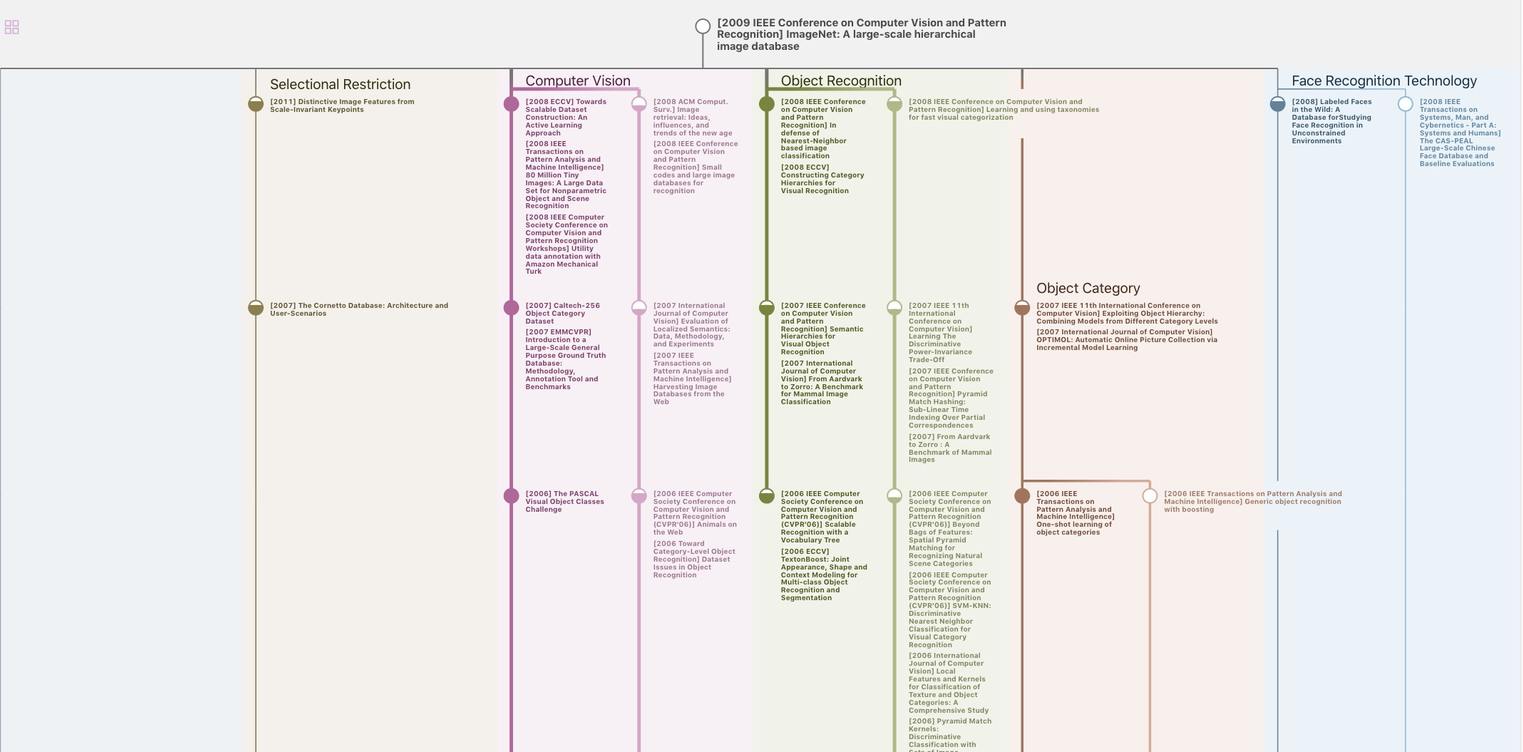
生成溯源树,研究论文发展脉络
Chat Paper
正在生成论文摘要