Mechanism Design for Large Language Models
CoRR(2023)
摘要
We investigate auction mechanisms to support the emerging format of AI-generated content. We in particular study how to aggregate several LLMs in an incentive compatible manner. In this problem, the preferences of each agent over stochastically generated contents are described/encoded as an LLM. A key motivation is to design an auction format for AI-generated ad creatives to combine inputs from different advertisers. We argue that this problem, while generally falling under the umbrella of mechanism design, has several unique features. We propose a general formalism -- the token auction model -- for studying this problem. A key feature of this model is that it acts on a token-by-token basis and lets LLM agents influence generated contents through single dimensional bids. We first explore a robust auction design approach, in which all we assume is that agent preferences entail partial orders over outcome distributions. We formulate two natural incentive properties, and show that these are equivalent to a monotonicity condition on distribution aggregation. We also show that for such aggregation functions, it is possible to design a second-price auction, despite the absence of bidder valuation functions. We then move to designing concrete aggregation functions by focusing on specific valuation forms based on KL-divergence, a commonly used loss function in LLM. The welfare-maximizing aggregation rules turn out to be the weighted (log-space) convex combination of the target distributions from all participants. We conclude with experimental results in support of the token auction formulation.
更多查看译文
关键词
large language models,large language,mechanism
AI 理解论文
溯源树
样例
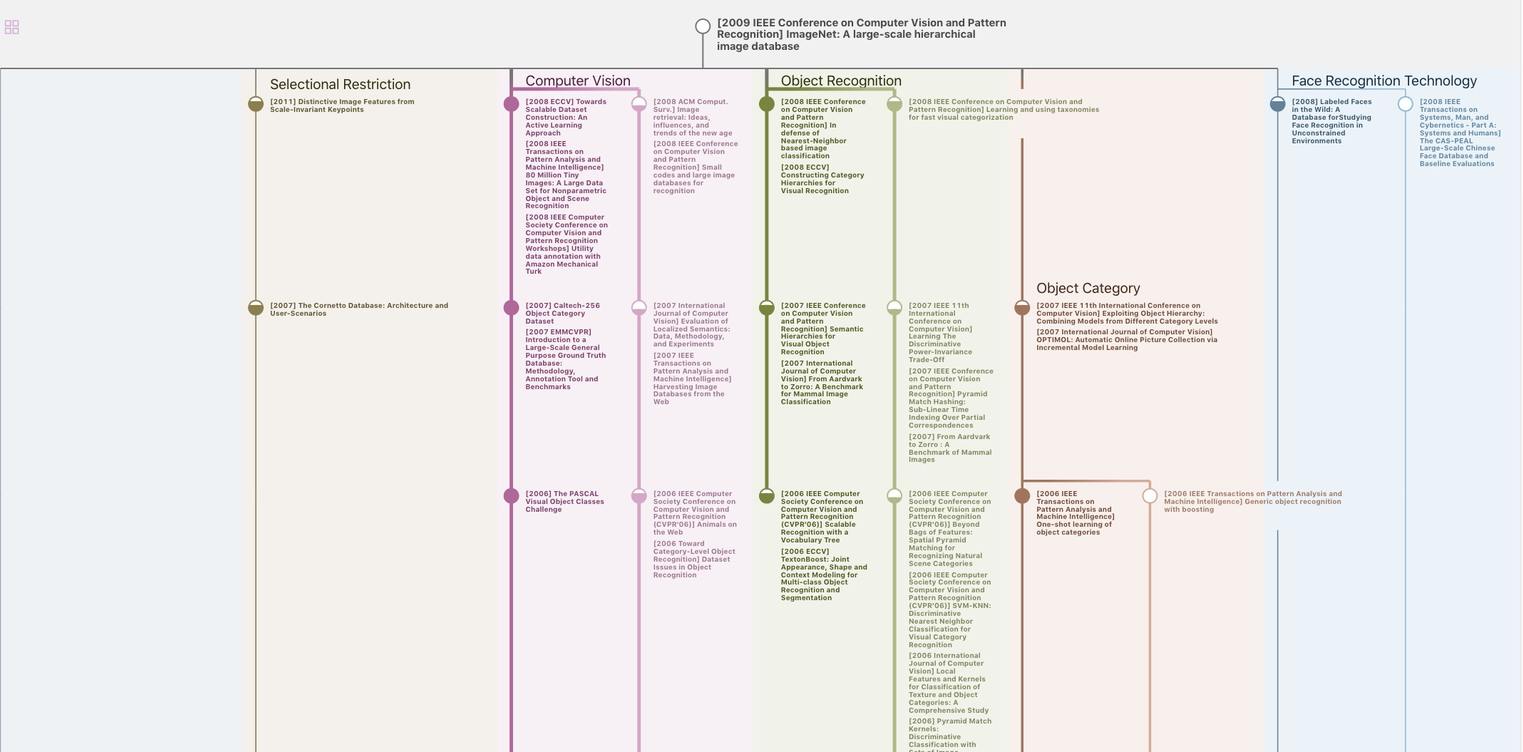
生成溯源树,研究论文发展脉络
Chat Paper
正在生成论文摘要