Self-Supervised Models of Speech Infer Universal Articulatory Kinematics
arXiv (Cornell University)(2023)
摘要
Self-Supervised Learning (SSL) based models of speech have shown remarkable performance on a range of downstream tasks. These state-of-the-art models have remained blackboxes, but many recent studies have begun "probing" models like HuBERT, to correlate their internal representations to different aspects of speech. In this paper, we show "inference of articulatory kinematics" as fundamental property of SSL models, i.e., the ability of these models to transform acoustics into the causal articulatory dynamics underlying the speech signal. We also show that this abstraction is largely overlapping across the language of the data used to train the model, with preference to the language with similar phonological system. Furthermore, we show that with simple affine transformations, Acoustic-to-Articulatory inversion (AAI) is transferrable across speakers, even across genders, languages, and dialects, showing the generalizability of this property. Together, these results shed new light on the internals of SSL models that are critical to their superior performance, and open up new avenues into language-agnostic universal models for speech engineering, that are interpretable and grounded in speech science.
更多查看译文
关键词
models,speech,self-supervised
AI 理解论文
溯源树
样例
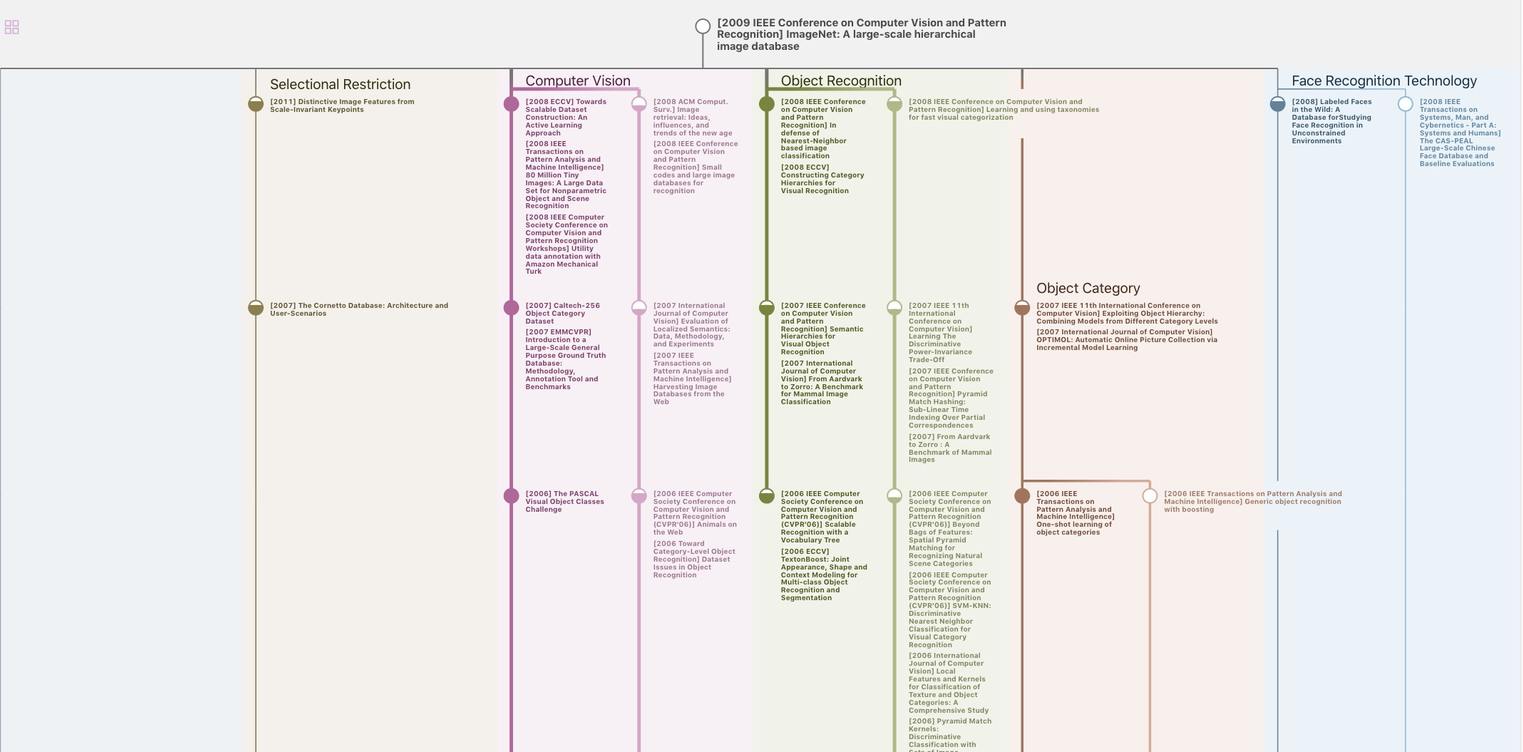
生成溯源树,研究论文发展脉络
Chat Paper
正在生成论文摘要