Nearly Optimal Approximation Rates for Deep Super ReLU Networks on Sobolev Spaces
CoRR(2023)
摘要
This paper introduces deep super ReLU networks (DSRNs) as a method for approximating functions in Sobolev spaces measured by Sobolev norms $W^{m,p}$ for $m\in\mathbb{N}$ with $m\ge 2$ and $1\le p\le +\infty$. Standard ReLU deep neural networks (ReLU DNNs) cannot achieve this goal. DSRNs consist primarily of ReLU DNNs, and several layers of the square of ReLU added at the end to smooth the networks output. This approach retains the advantages of ReLU DNNs, leading to the straightforward training. The paper also proves the optimality of DSRNs by estimating the VC-dimension of higher-order derivatives of DNNs, and obtains the generalization error in Sobolev spaces via an estimate of the pseudo-dimension of higher-order derivatives of DNNs.
更多查看译文
关键词
deep super relu networks,optimal approximation rates,sobolev spaces
AI 理解论文
溯源树
样例
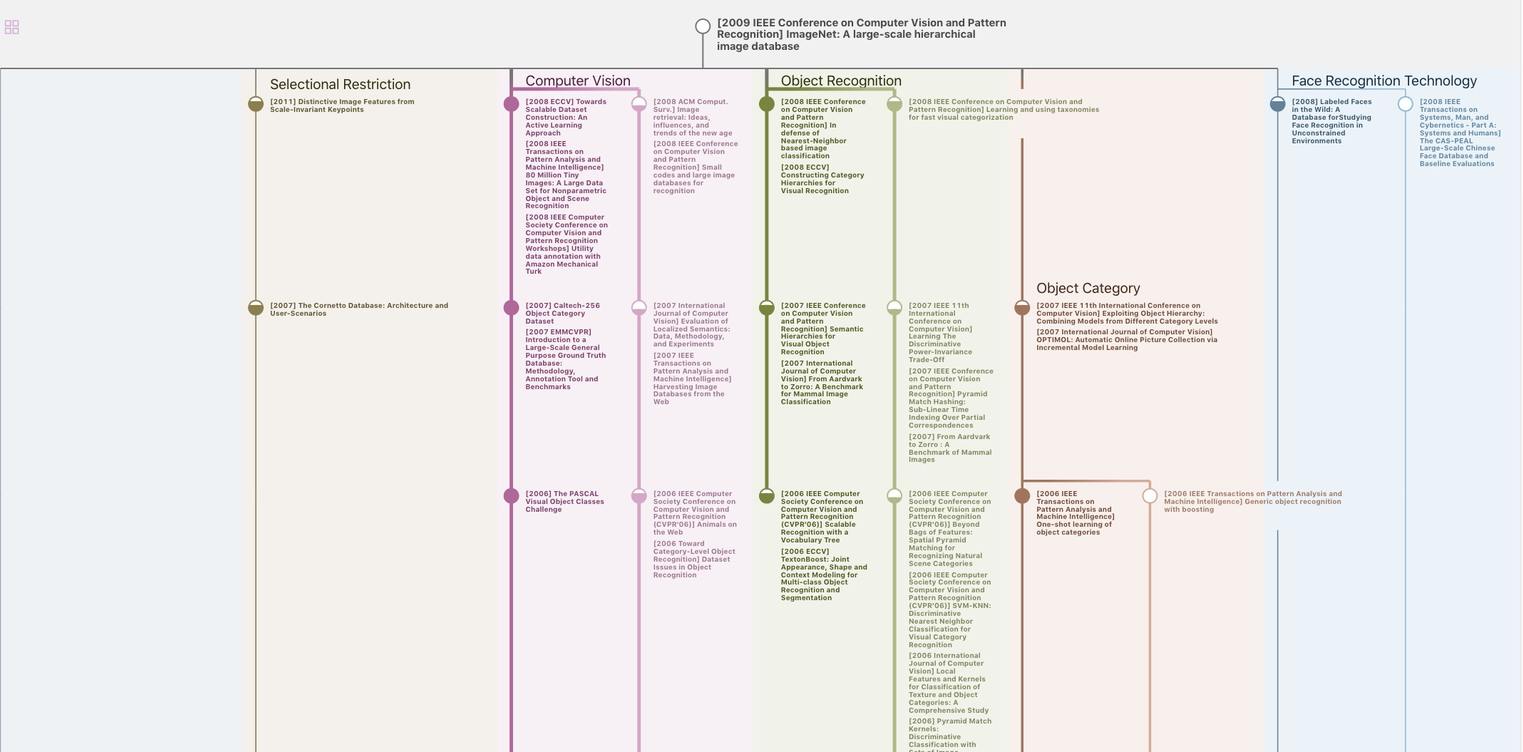
生成溯源树,研究论文发展脉络
Chat Paper
正在生成论文摘要