Synthetic IMU Datasets and Protocols Can Simplify Fall Detection Experiments and Optimize Sensor Configuration.
IEEE transactions on neural systems and rehabilitation engineering : a publication of the IEEE Engineering in Medicine and Biology Society(2024)
摘要
Falls represent a significant cause of injury among the elderly population. Extensive research has been devoted to the utilization of wearable IMU sensors in conjunction with machine learning techniques for fall detection. To address the challenge of acquiring costly training data, this paper presents a novel method that generates a substantial volume of synthetic IMU data with minimal actual fall experiments. First, unmarked 3D motion capture technology is employed to reconstruct human movements. Subsequently, utilizing the biomechanical simulation platform Opensim and forward kinematic methods, an ample amount of training data from various body segments can be custom generated. Synthetic IMU data was then used to train a machine learning model, achieving testing accuracies of 91.99% and 86.62% on two distinct datasets of actual fall-related IMU data. Building upon the simulation framework, this paper further optimized the single IMU attachment position and multiple IMU combinations on fall detection. The proposed method simplifies fall detection data acquisition experiments, provides novel venue for generating low cost synthetic data in scenario where acquiring data for machine learning is challenging and paves the way for customizing machine learning configurations.
更多查看译文
关键词
Fall detection,IMU,Opensim,Data augmentation
AI 理解论文
溯源树
样例
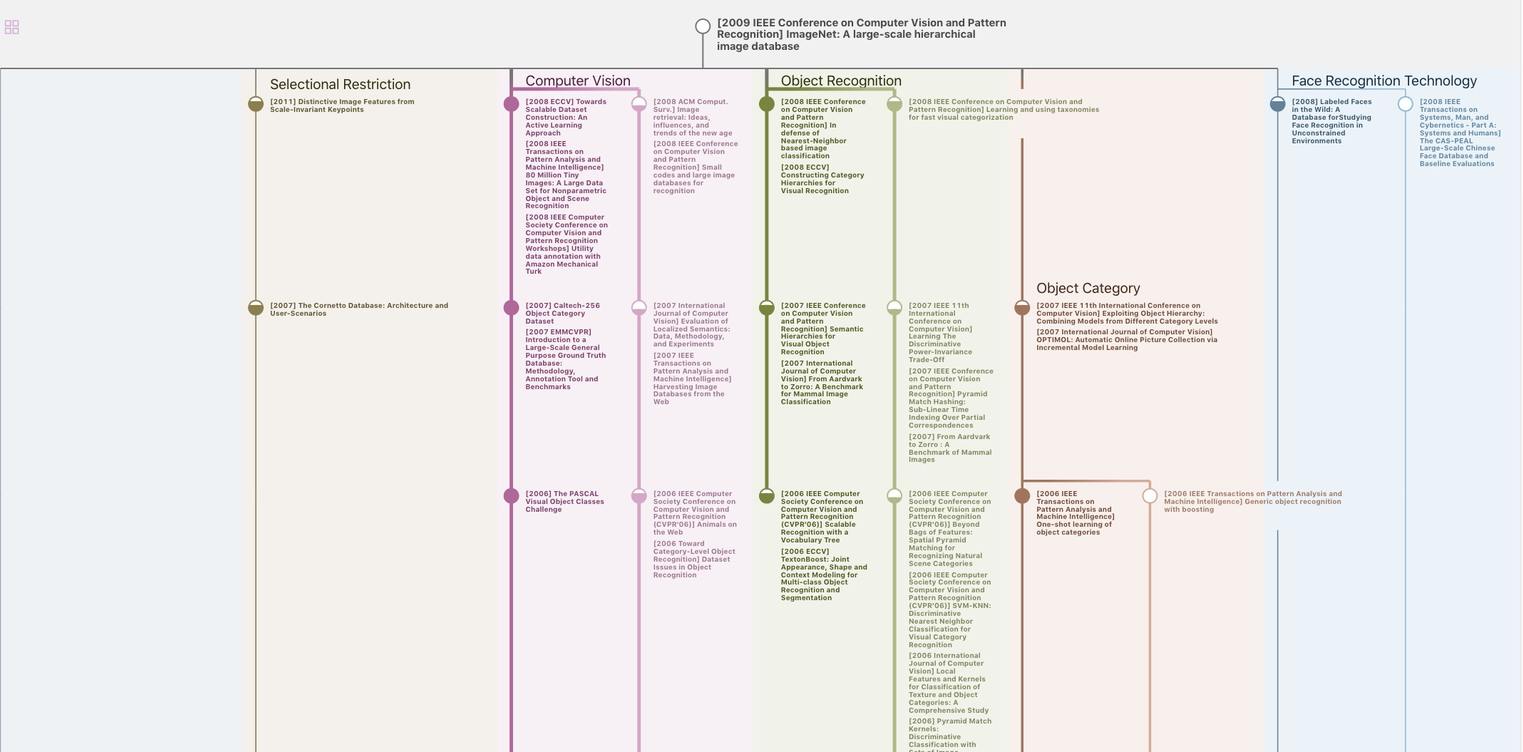
生成溯源树,研究论文发展脉络
Chat Paper
正在生成论文摘要