Low-dimensional neural ODEs and their application in pharmacokinetics
Journal of Pharmacokinetics and Pharmacodynamics(2023)
摘要
Machine Learning (ML) is a fast-evolving field, integrated in many of today’s scientific disciplines. With the recent development of neural ordinary differential equations (NODEs), ML provides a new tool to model dynamical systems in the field of pharmacology and pharmacometrics, such as pharmacokinetics (PK) or pharmacodynamics. The novel and conceptionally different approach of NODEs compared to classical PK modeling creates challenges but also provides opportunities for its application. In this manuscript, we introduce the functionality of NODEs and develop specific low-dimensional NODE structures based on PK principles. We discuss two challenges of NODEs, overfitting and extrapolation to unseen data, and provide practical solutions to these problems. We illustrate concept and application of our proposed low-dimensional NODE approach with several PK modeling examples, including multi-compartmental, target-mediated drug disposition, and delayed absorption behavior. In all investigated scenarios, the NODEs were able to describe the data well and simulate data for new subjects within the observed dosing range. Finally, we briefly demonstrate how NODEs can be combined with mechanistic models. This research work enhances understanding of how NODEs can be applied in PK analyses and illustrates the potential for NODEs in the field of pharmacology and pharmacometrics.
更多查看译文
关键词
Pharmacometrics,Pharmacokinetics,Machine learning,Neural ordinary differential equations,Neural networks
AI 理解论文
溯源树
样例
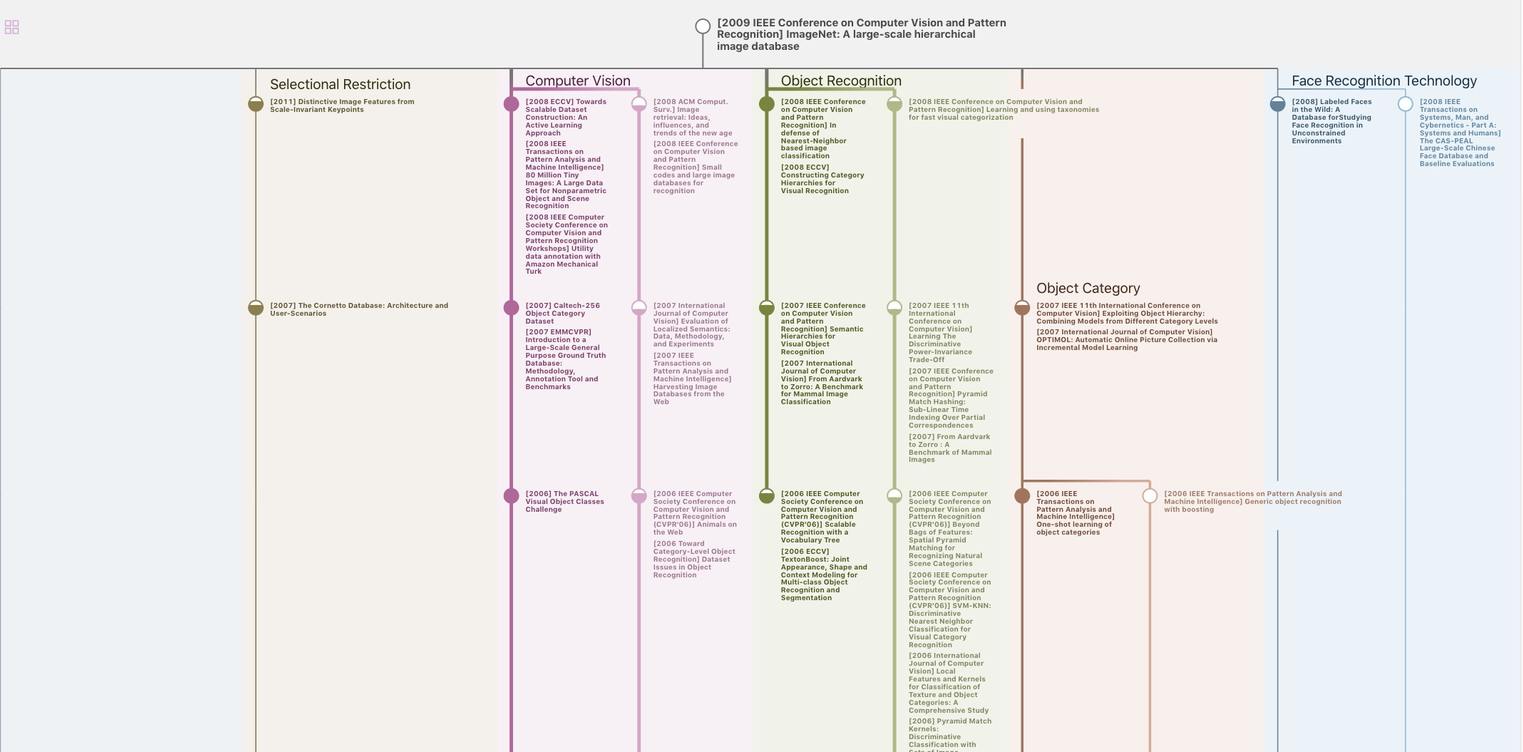
生成溯源树,研究论文发展脉络
Chat Paper
正在生成论文摘要