Pathological brain classification using multiple kernel-based deep convolutional neural network
Neural Computing and Applications(2024)
摘要
Conventionally, fine-tuning or transfer learning using a pre-trained convolutional network is adopted to design a classifier. However, when the dataset is small this can deteriorate the classifier generalization performance due to negative transfer or overfitting issues. In this paper, we suggest a flexible and high-capacity multiple kernel-based convolutional neural network (MK-CNN) to automate the pathological brain classification task. The proposed network employed different stacks of convolution with various kernels to obtain multi-scale features from the input image. The smaller kernel size provides specific information about the local features whereas the larger kernel size provides the global spatial information. Hence, the network takes into account both regional specifics and global spatial consistency thanks to this multi-scale methodology. Only the output layer is shared between each network stack. This makes it possible to specifically tweak the CNN’s weights and biases for each convolution stack and associated kernel size. The results reported on real patient data from the Harvard Whole Brain Atlas reveal that our method outperforms state-of-the-art techniques. The suggested approach may be used to help experts carry out the clinical follow-up study.
更多查看译文
关键词
Deep learning,Convolutional neural networks,Classifier,Pathological brain classification
AI 理解论文
溯源树
样例
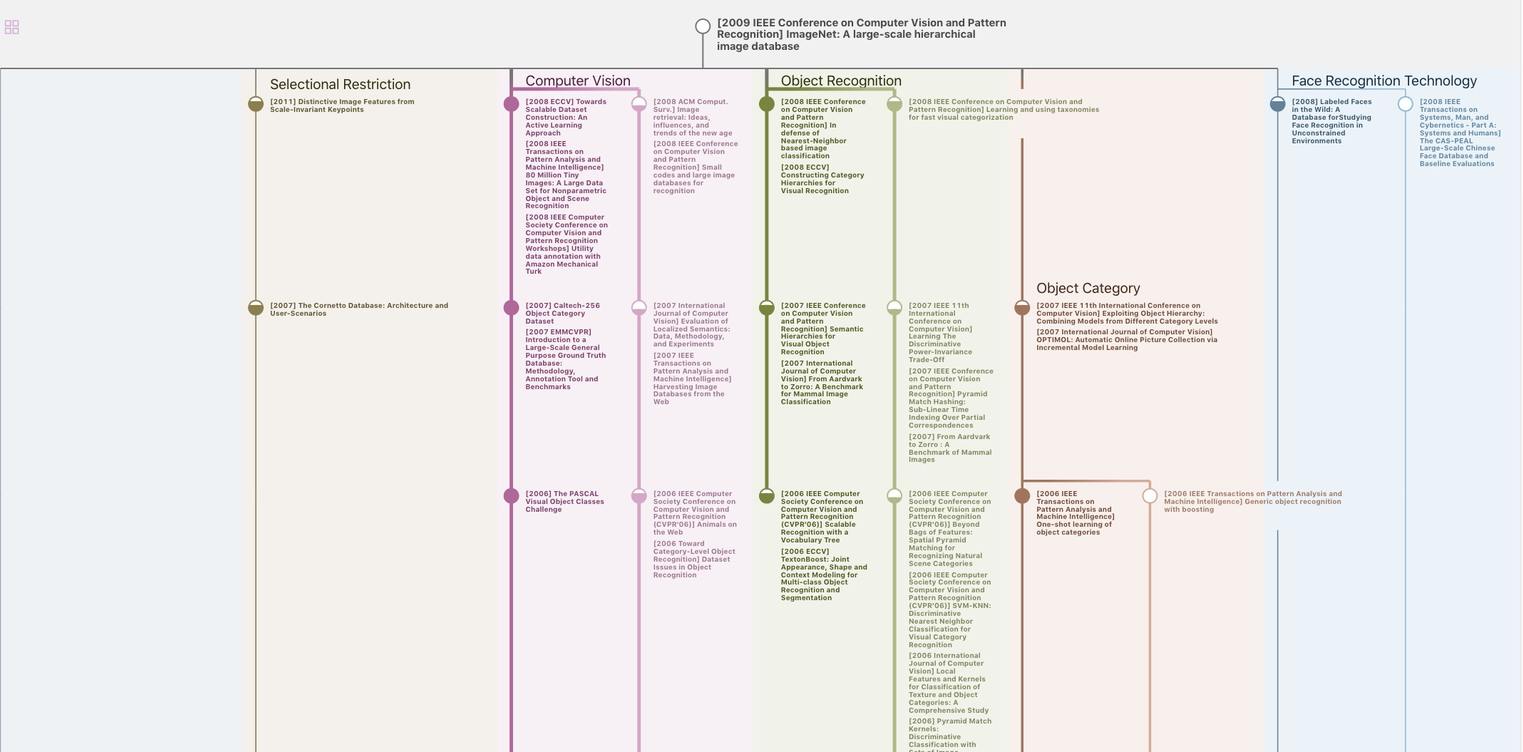
生成溯源树,研究论文发展脉络
Chat Paper
正在生成论文摘要