Subspace clustering based on a multichannel attention mechanism
International Journal of Machine Learning and Cybernetics(2024)
摘要
Existing self-representation models based on multilayer perceptrons (MLPs) have gained widespread attention for their outstanding clustering performance in subspace clustering. However, when images contain rich spatial information, the use of fully connected neural networks that only accept vector inputs results in a significant loss of spatial information, thereby greatly reducing the clustering performance of the models. To address the clustering problem of data with rich spatial information, this paper proposes a multichannel subspace clustering method based on a self-representation network (CGSNet). This method incorporates modules capable of mining spatial features into the self-representation network to highlight different data characteristics and supplement the spatial information lost by the MLP. CGSNet successfully uncovers the latent features within the input data samples and extracts the spatial relationships among different image features by employing channel and spatial attention modules. Additionally, static parameterized channel mapping and spatial mapping are used to refine and filter the obtained spatial information, further enhancing the quality of self-representation. Finally, by leveraging the self-representation network, the clustering task is completed by learning the affinity matrix. The experimental results demonstrate that CGSNet outperforms the self-expressive network (SENet), achieving improvements of 1.5%, 3.3%, 0.9%, and 4.4% in terms of the clustering accuracy with the MNIST, FashionMNIST, CIFAR-10, and EMNIST datasets, respectively. CGSNet achieves the highest accuracy among competitive clustering methods, including EnSC, SENet, and 14 others.
更多查看译文
关键词
Self-expressive network,Subspace clustering,Attention mechanism,Image clustering
AI 理解论文
溯源树
样例
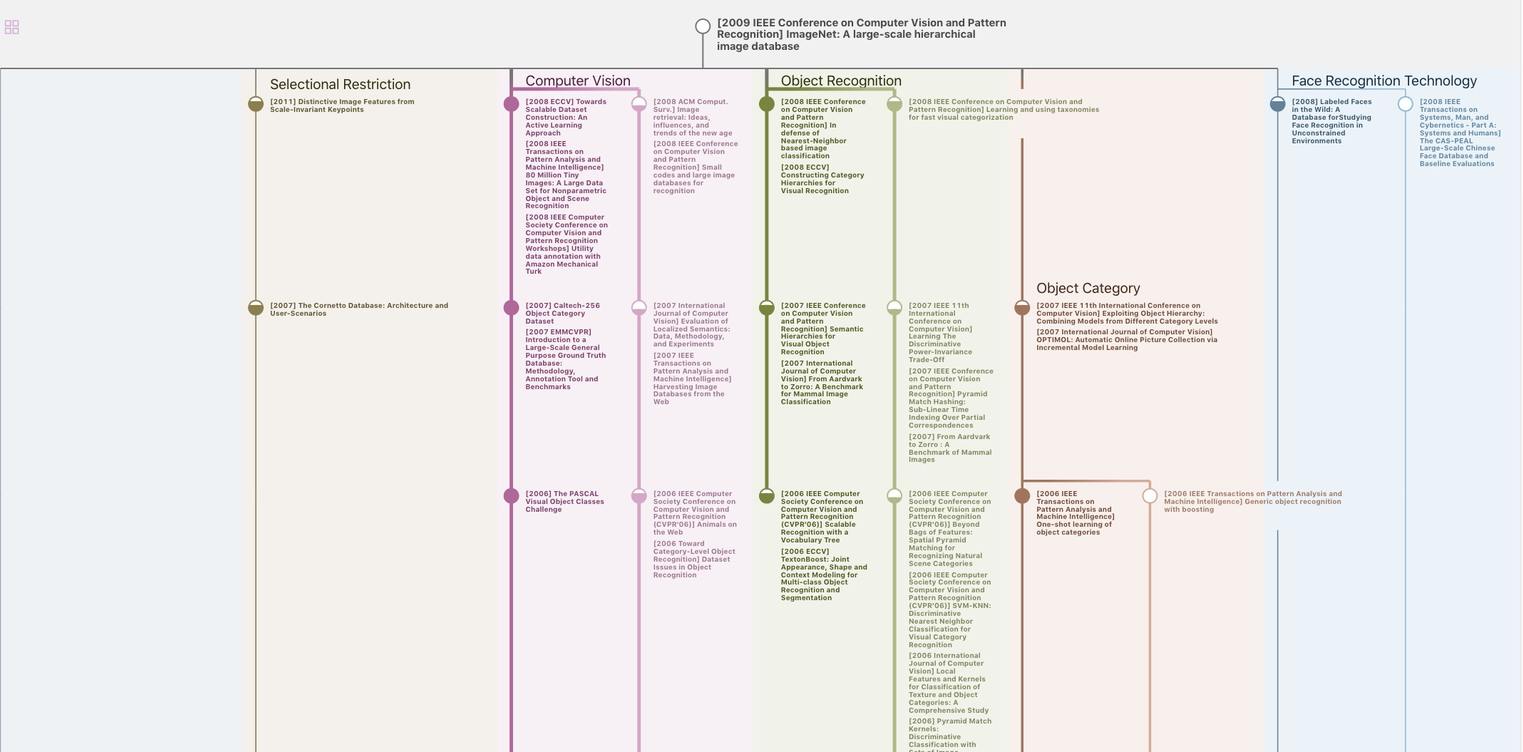
生成溯源树,研究论文发展脉络
Chat Paper
正在生成论文摘要