WeedsNet: a dual attention network with RGB-D image for weed detection in natural wheat field
PRECISION AGRICULTURE(2024)
摘要
For weed detection in wheat field, it is difficult to identify weeds against the complex field background using single-modal red–green–blue (RGB) images due to similar appearance of grass weeds and wheat. To overcome limitations of single-modal information in grass weed detection, a dual-path weed detection network (WeedsNet) based on multi-modal information is proposed. At first, the single-channel depth image is encoded as a new three-channel image having similar structures with RGB color space, so that they are suitable for feature extraction using a convolutional neural network (CNN). Then, WeedsNet comprising a dual-path feature extraction network is constructed to extract features of weeds from RGB and depth images simultaneously. Finally, weights are assigned to features in different modalities in tandem with the idea of multi-scale object detection and the attention mechanism, thus effectively fusing multi-modal information. The results of the interpretability analysis of the model demonstrate that depth information is beneficial to achieve the detection of grass weeds, and effectively improves the weed detection accuracy in wheat field by complementing with RGB image features. WeedsNet has better detection accuracy than both traditional machine learning methods and integrated learning-based weight assignment methods. The weed detection accuracy is 62.3% based on WeedsNet in natural wheat field and the detection speed of single image was about 0.5s. Weed detection software is designed and developed based on WeedsNet to achieve real-time output of weeds distribution information in natural wheat field.
更多查看译文
关键词
Weed detection,Natural wheat field,Multi-scale weed detection,Multi-modal information fusion
AI 理解论文
溯源树
样例
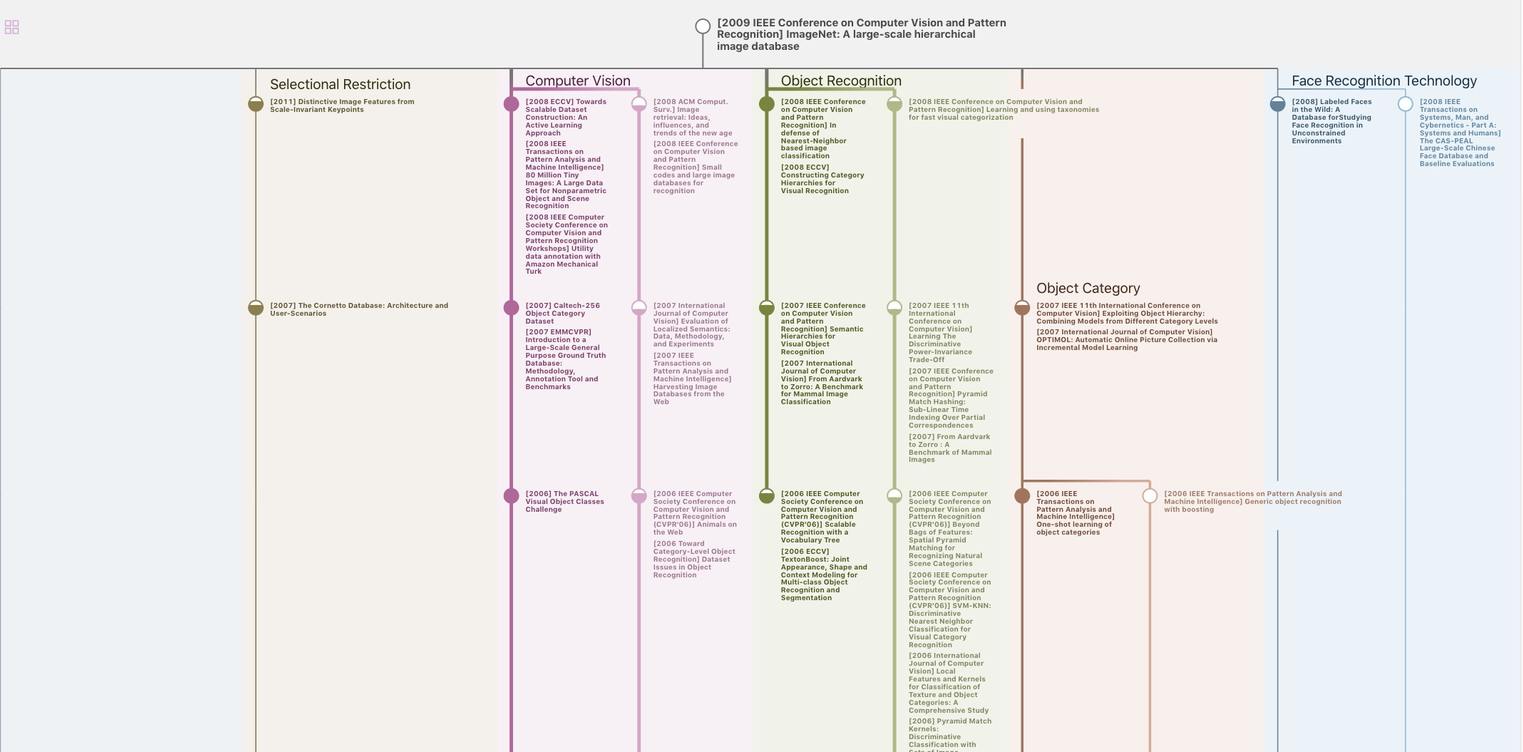
生成溯源树,研究论文发展脉络
Chat Paper
正在生成论文摘要