CodonBERT: Using BERT for Sentiment Analysis to Better Predict Genes with Low Expression
14TH ACM CONFERENCE ON BIOINFORMATICS, COMPUTATIONAL BIOLOGY, AND HEALTH INFORMATICS, BCB 2023(2023)
摘要
Synonymous codons, which encode the same amino acid in a protein, are known to be used unequally in organisms. Prior research has been able to uncover "preferred" codons that are often found in more highly expressed genes. This has enabled different computational models that can predict gene expression of protein-coding genes; however, their performance is often affected by more diverse gene expression in higher organisms, i.e., high expression in only specific tissues or cell types. In this paper, we use a Natural Language Processing (NLP) algorithm, Bidirectional Encoder Representations from Transformers (BERT), to develop a new framework for predicting gene expression. Notably, our model architecture relies on the idea of sentiment analysis, i.e., assigning an overall "emotion" (sentiment) to protein-coding sequences. Our new framework, CodonBERT, is a a pre-trained model that better captures more intrinsic relationships between sequences and their expression, and we show that our model is capable of making substantially better predictions for a diverse collection of model organisms. Additionally, we show that our model learns inherent patterns of codon usage that can be traced using explainable AI (XAI) algorithms.
更多查看译文
关键词
CUB,Expression,Sentiment Analysis,Transformers,SHAP
AI 理解论文
溯源树
样例
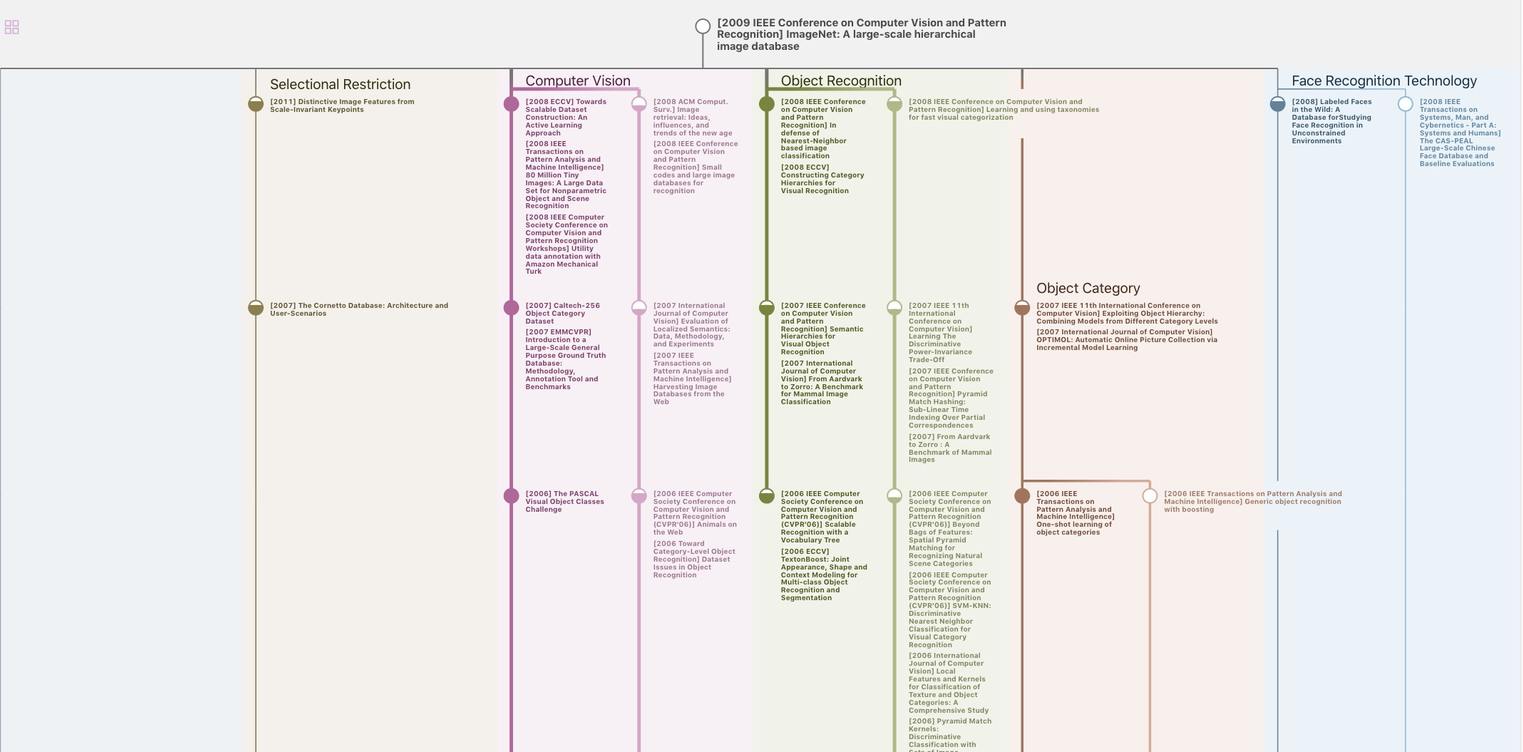
生成溯源树,研究论文发展脉络
Chat Paper
正在生成论文摘要