People Perceive Algorithmic Assessments as Less Fair and Trustworthy Than Identical Human Assessments.
Proceedings of the ACM on Human-Computer Interaction(2023)
摘要
Algorithmic risk assessments are being deployed in an increasingly broad spectrum of domains including banking, medicine, and law enforcement. However, there is widespread concern about their fairness and trustworthiness, and people are also known to display algorithm aversion, preferring human assessments even when they are quantitatively worse. Thus, how does the framing of who made an assessment affect how people perceive its fairness? We investigate whether individual algorithmic assessments are perceived to be more or less accurate, fair, and interpretable than identical human assessments, and explore how these perceptions change when assessments are obviously biased against a subgroup. To this end, we conducted an online experiment that manipulated how biased risk assessments are in a loan repayment task, and reported the assessments as being made either by a statistical model or a human analyst. We find that predictions made by the model are consistently perceived as less fair and less interpretable than those made by the analyst despite being identical. Furthermore, biased predictive errors were more likely to widen this perception gap, with the algorithm being judged even more harshly for making a biased mistake. Our results illustrate that who makes risk assessments can influence perceptions of how acceptable those assessments are - even if they are identically accurate and identically biased against subgroups. Additional work is needed to determine whether and how decision aids should be presented to stakeholders so that the inherent fairness and interpretability of their recommendations, rather than their framing, determines how they are perceived.
更多查看译文
关键词
algorithm aversion,bias,fairness,risk assessment
AI 理解论文
溯源树
样例
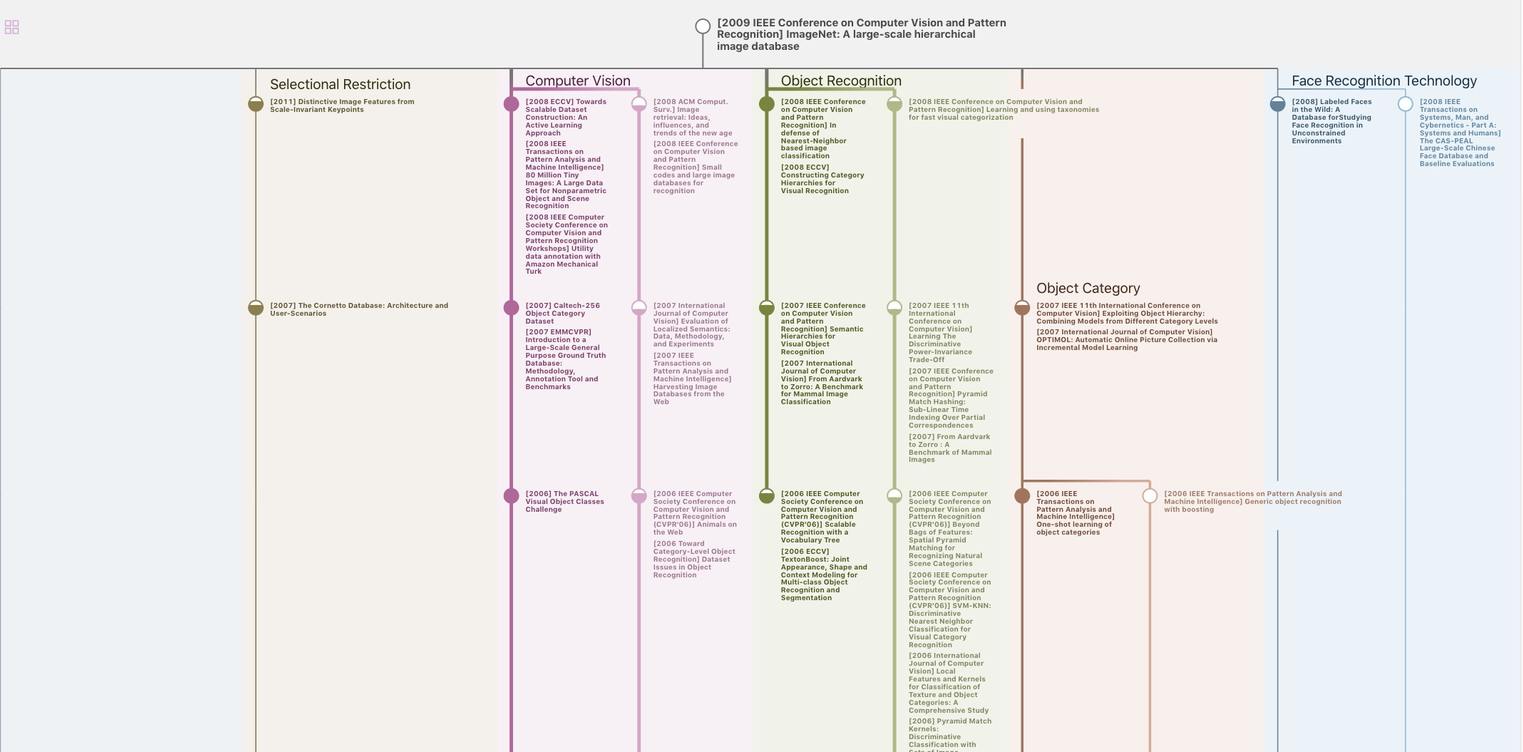
生成溯源树,研究论文发展脉络
Chat Paper
正在生成论文摘要