Revisiting multi-GNSS Navigation for UAVs An Equivariant Filtering Approach
2023 21ST INTERNATIONAL CONFERENCE ON ADVANCED ROBOTICS, ICAR(2023)
摘要
In this work, we explore the recent advances in equivariant filtering for inertial navigation systems to improve state estimation for uncrewed aerial vehicles (UAVs). Traditional state-of-the-art estimation methods, e.g., the multiplicative Kalman filter (MEKF), have some limitations concerning their consistency, errors in the initial state estimate, and convergence performance. Symmetry-based methods, such as the equivariant filter (EqF), offer significant advantages for these points by exploiting the mathematical properties of the system - its symmetry. These filters yield faster convergence rates and robustness to wrong initial state estimates through their error definition. To demonstrate the usability of EqFs, we focus on the sensor-fusion problem with the most common sensors in outdoor robotics: global navigation satellite system (GNSS) sensors and an inertial measurement unit (IMU). We provide an implementation of such an EqF leveraging the semi-direct product of the symmetry group to derive the filter equations. To validate the practical usability of EqFs in real-world scenarios, we evaluate our method using data from all outdoor runs of the INSANE Dataset. Our results demonstrate the performance improvements of the EqF in real-world environments, highlighting its potential for enhancing state estimation for UAVs.
更多查看译文
关键词
Unmanned Aerial Vehicles,Equivalency,Kalman Filter,Aerial Vehicles,Real-world Scenarios,Inertial Measurement Unit,Global Navigation Satellite System,Symmetry Group,Real-world Environments,Inertial System,Inertial Navigation,Semidirect Product,System Dynamics,State Space,Angular Velocity,Local Coordinate,Sensor Locations,Linear Accelerator,Sensor Calibration,Extended Kalman Filter,Global Frame,Lie Group,Robust Filter,Lie Algebra,Linear Error,Filter Design,Mathematical Preliminaries,Form Of Filter
AI 理解论文
溯源树
样例
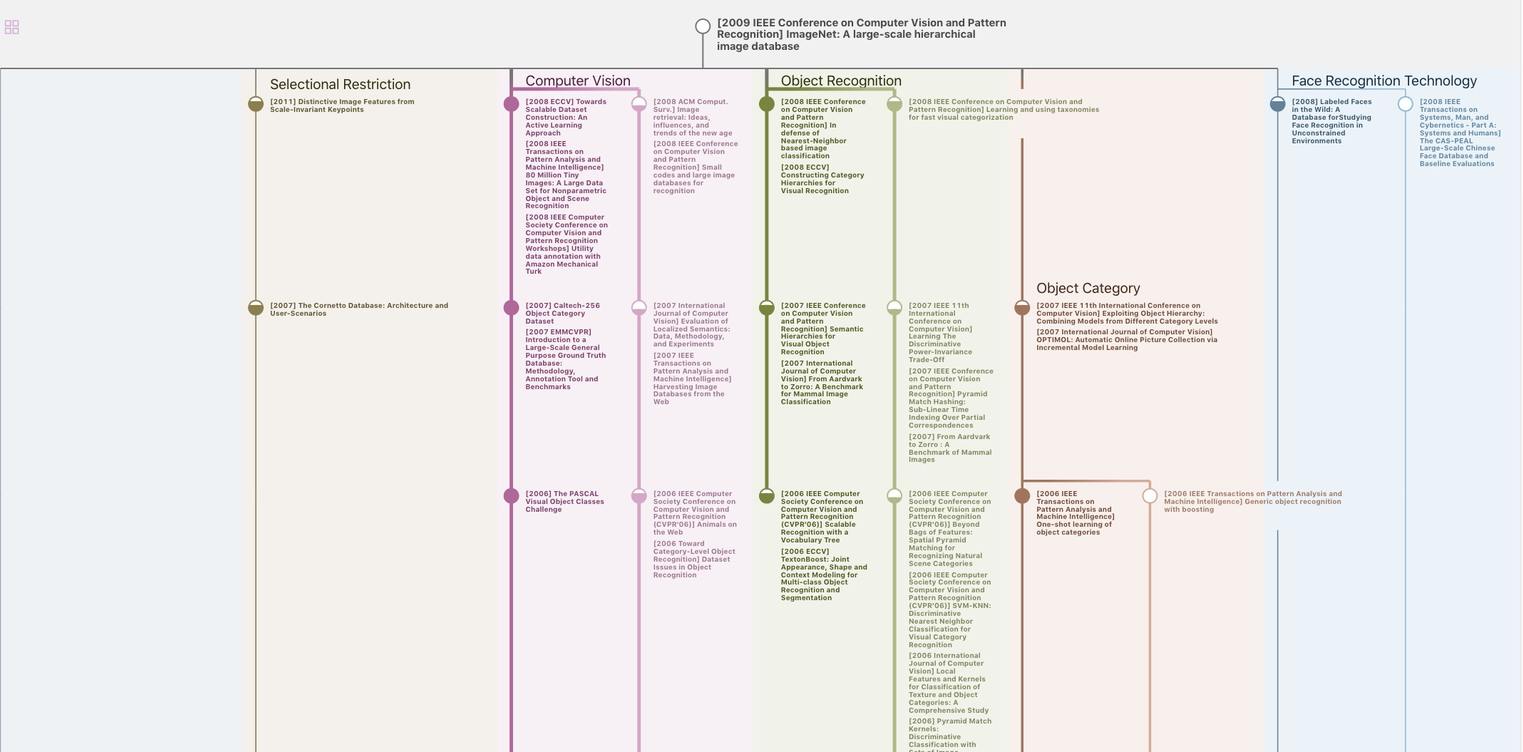
生成溯源树,研究论文发展脉络
Chat Paper
正在生成论文摘要