LMT: Longitudinal Mixing Training, a Framework to Predict Disease Progression from a Single Image
MACHINE LEARNING IN MEDICAL IMAGING, MLMI 2023, PT II(2024)
摘要
Longitudinal imaging is able to capture both static anatomical structures and dynamic changes in disease progression toward earlier and better patient-specific pathology management. However, conventional approaches rarely take advantage of longitudinal information for detection and prediction purposes, especially for Diabetic Retinopathy (DR). In the past years, Mix-up training and pretext tasks with longitudinal context have effectively enhanced DR classification results and captured disease progression. In the meantime, a novel type of neural network named Neural Ordinary Differential Equation (NODE) has been proposed for solving ordinary differential equations, with a neural network treated as a black box. By definition, NODE is well suited for solving time-related problems. In this paper, we propose to combine these three aspects to detect and predict DR progression. Our framework, Longitudinal Mixing Training (LMT), can be considered both as a regularizer and as a pretext task that encodes the disease progression in the latent space. Additionally, we evaluate the trained model weights on a down-stream task with a longitudinal context using standard and longitudinal pretext tasks. We introduce a new way to train time-aware models using t(mix), a weighted average time between two consecutive examinations. We compare our approach to standard mixing training on DR classification using OPHDIAT a longitudinal retinal Color Fundus Photographs (CFP) dataset. We were able to predict whether an eye would develop a severe DR in the following visit using a single image, with an AUC of 0.798 compared to baseline results of 0.641. Our results indicate that our longitudinal pretext task can learn the progression of DR disease and that introducing tmix augmentation is beneficial for time-aware models.
更多查看译文
关键词
Disease progression mix-up training,diabetic retinopathy,time-aware model,predictive medicine
AI 理解论文
溯源树
样例
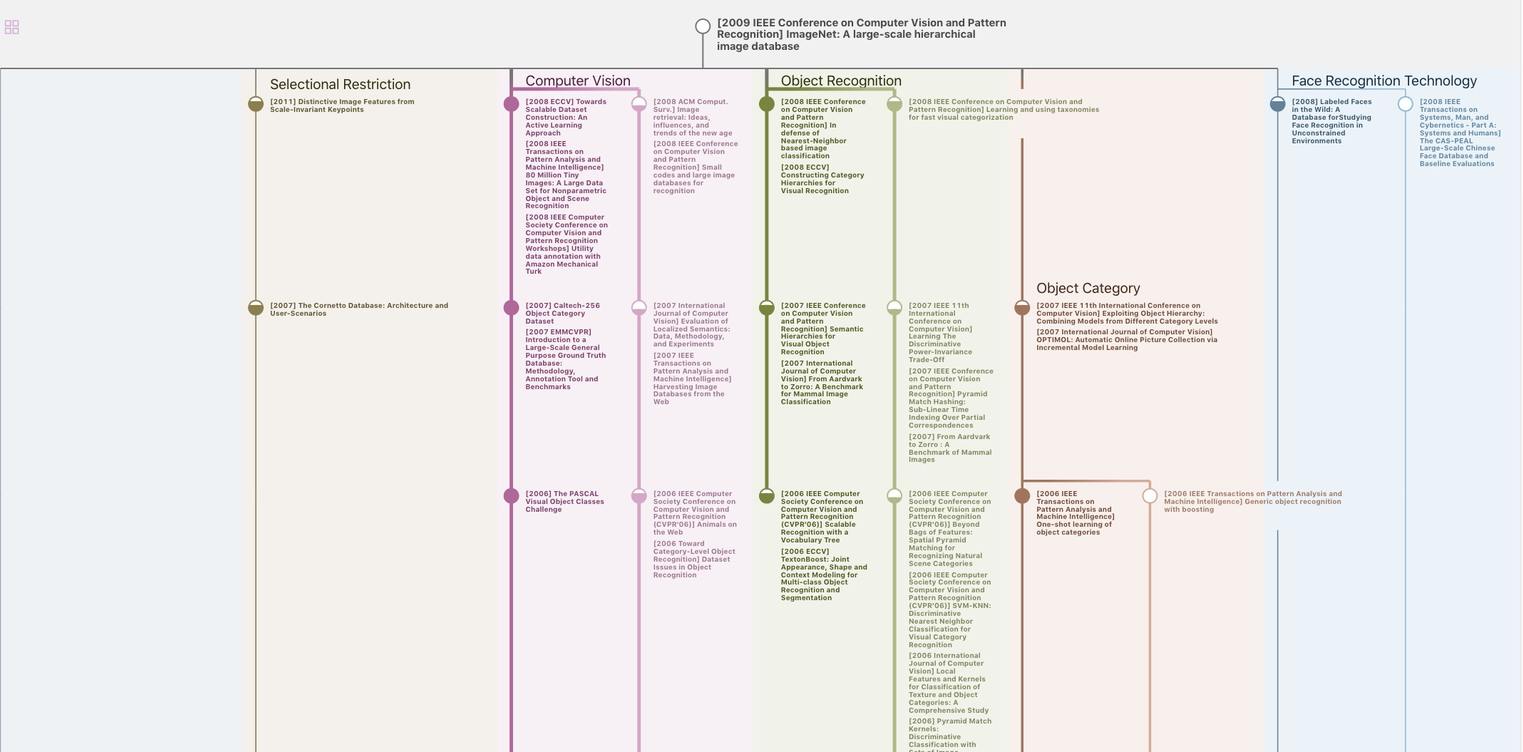
生成溯源树,研究论文发展脉络
Chat Paper
正在生成论文摘要