Towards Open World Active Learning for 3D Object Detection
CoRR(2023)
摘要
Significant strides have been made in closed world 3D object detection, testing systems in environments with known classes. However, the challenge arises in open world scenarios where new object classes appear. Existing efforts sequentially learn novel classes from streams of labeled data at a significant annotation cost, impeding efficient deployment to the wild. To seek effective solutions, we investigate a more practical yet challenging research task: Open World Active Learning for 3D Object Detection (OWAL-3D), aiming at selecting a small number of 3D boxes to annotate while maximizing detection performance on both known and unknown classes. The core difficulty centers on striking a balance between mining more unknown instances and minimizing the labeling expenses of point clouds. Empirically, our study finds the harmonious and inverse relationship between box quantities and their confidences can help alleviate the dilemma, avoiding the repeated selection of common known instances and focusing on uncertain objects that are potentially unknown. We unify both relational constraints into a simple and effective AL strategy namely OpenCRB, which guides to acquisition of informative point clouds with the least amount of boxes to label. Furthermore, we develop a comprehensive codebase for easy reproducing and future research, supporting 15 baseline methods (i.e., active learning, out-of-distribution detection and open world detection), 2 types of modern 3D detectors (i.e., one-stage SECOND and two-stage PV-RCNN) and 3 benchmark 3D datasets (i.e., KITTI, nuScenes and Waymo). Extensive experiments evidence that the proposed Open-CRB demonstrates superiority and flexibility in recognizing both novel and shared categories with very limited labeling costs, compared to state-of-the-art baselines.
更多查看译文
关键词
3d object detection,open world active learning,object detection
AI 理解论文
溯源树
样例
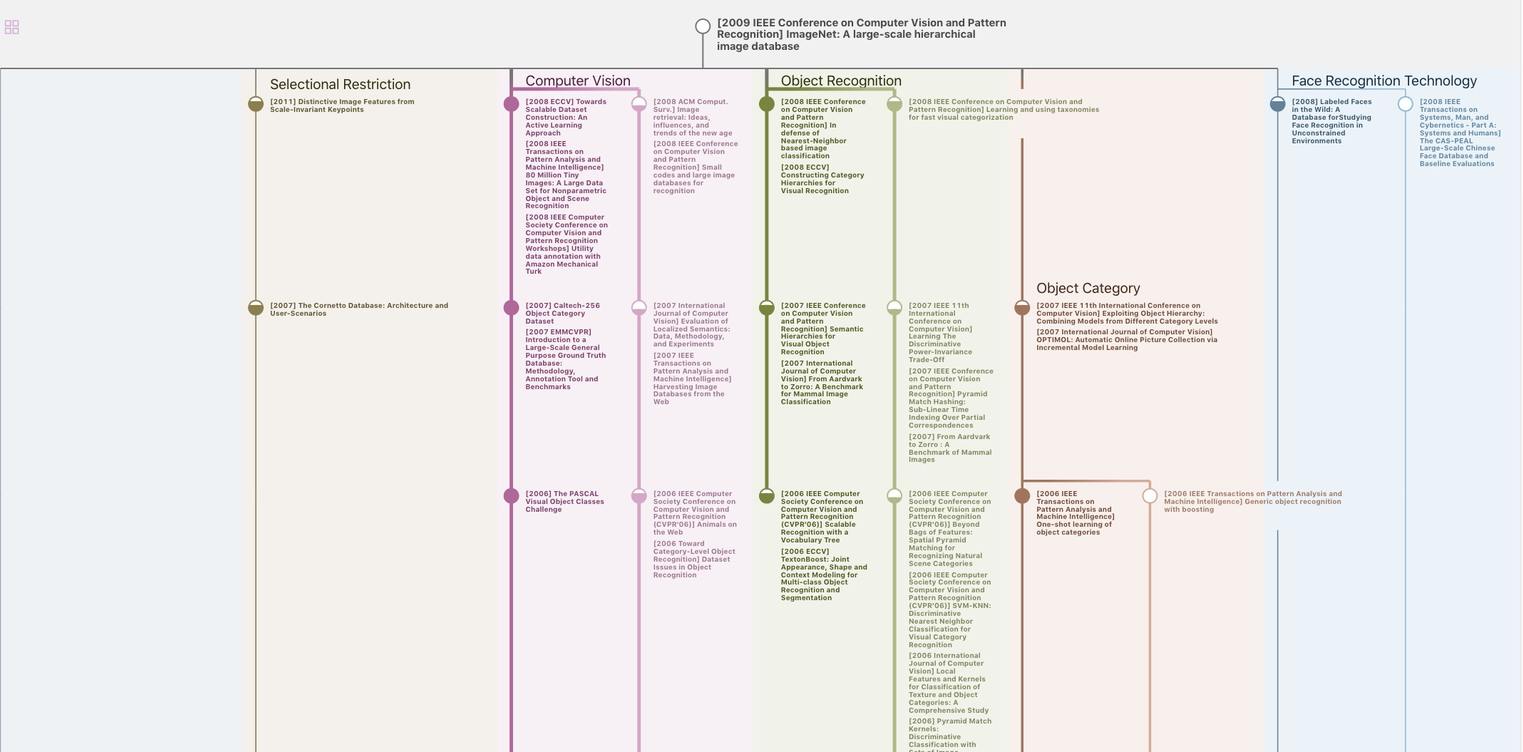
生成溯源树,研究论文发展脉络
Chat Paper
正在生成论文摘要