Tabular Representation, Noisy Operators, and Impacts on Table Structure Understanding Tasks in LLMs
CoRR(2023)
摘要
Large language models (LLMs) are increasingly applied for tabular tasks using in-context learning. The prompt representation for a table may play a role in the LLMs ability to process the table. Inspired by prior work, we generate a collection of self-supervised structural tasks (e.g. navigate to a cell and row; transpose the table) and evaluate the performance differences when using 8 formats. In contrast to past work, we introduce 8 noise operations inspired by real-world messy data and adversarial inputs, and show that such operations can impact LLM performance across formats for different structural understanding tasks.
更多查看译文
关键词
table structure,tabular representation,llms,understanding tasks
AI 理解论文
溯源树
样例
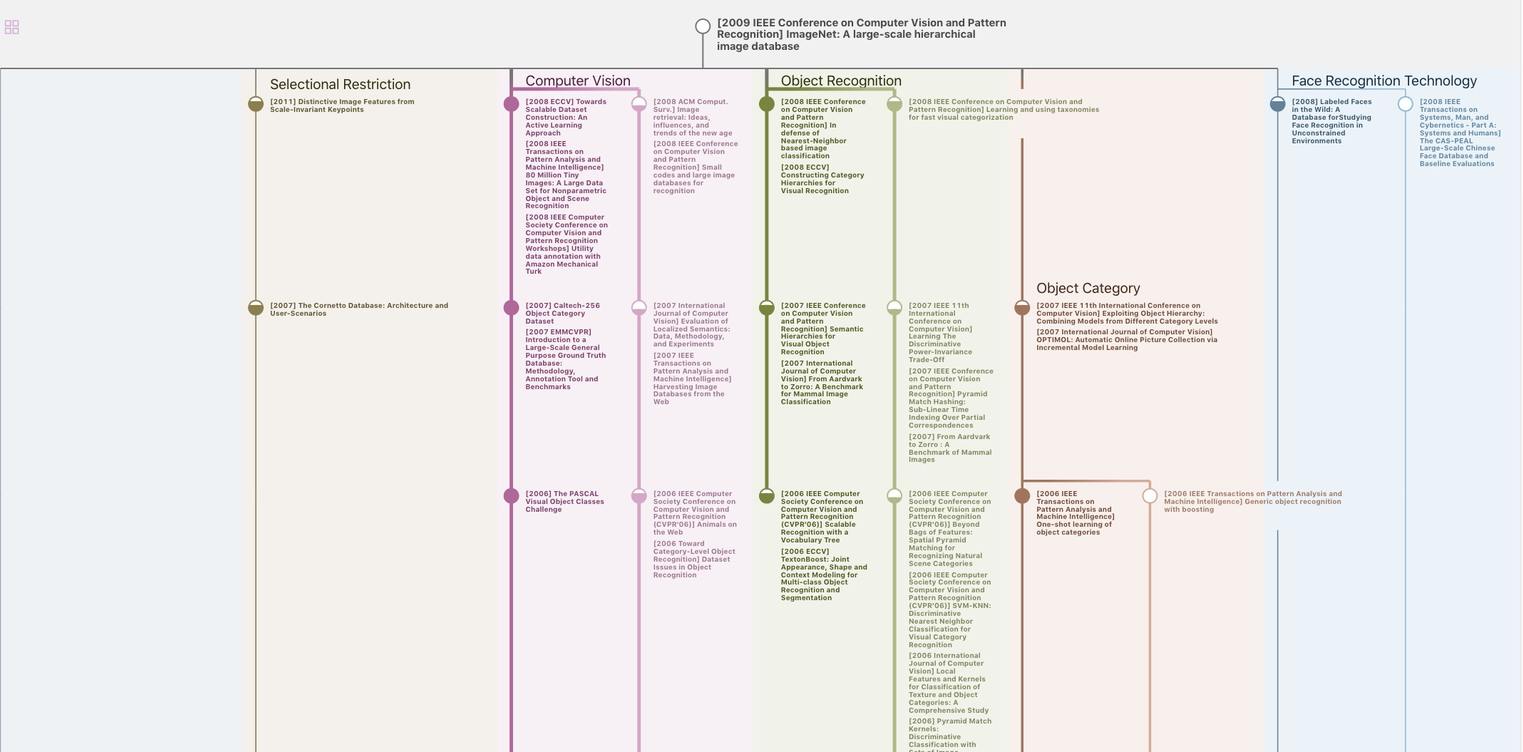
生成溯源树,研究论文发展脉络
Chat Paper
正在生成论文摘要