Generalizable Person Search on Open-world User-Generated Video Content
CoRR(2023)
摘要
Person search is a challenging task that involves detecting and retrieving individuals from a large set of un-cropped scene images. Existing person search applications are mostly trained and deployed in the same-origin scenarios. However, collecting and annotating training samples for each scene is often difficult due to the limitation of resources and the labor cost. Moreover, large-scale intra-domain data for training are generally not legally available for common developers, due to the regulation of privacy and public security. Leveraging easily accessible large-scale User Generated Video Contents (\emph{i.e.} UGC videos) to train person search models can fit the open-world distribution, but still suffering a performance gap from the domain difference to surveillance scenes. In this work, we explore enhancing the out-of-domain generalization capabilities of person search models, and propose a generalizable framework on both feature-level and data-level generalization to facilitate downstream tasks in arbitrary scenarios. Specifically, we focus on learning domain-invariant representations for both detection and ReID by introducing a multi-task prototype-based domain-specific batch normalization, and a channel-wise ID-relevant feature decorrelation strategy. We also identify and address typical sources of noise in open-world training frames, including inaccurate bounding boxes, the omission of identity labels, and the absence of cross-camera data. Our framework achieves promising performance on two challenging person search benchmarks without using any human annotation or samples from the target domain.
更多查看译文
关键词
generalizable person search,video content,open-world,user-generated
AI 理解论文
溯源树
样例
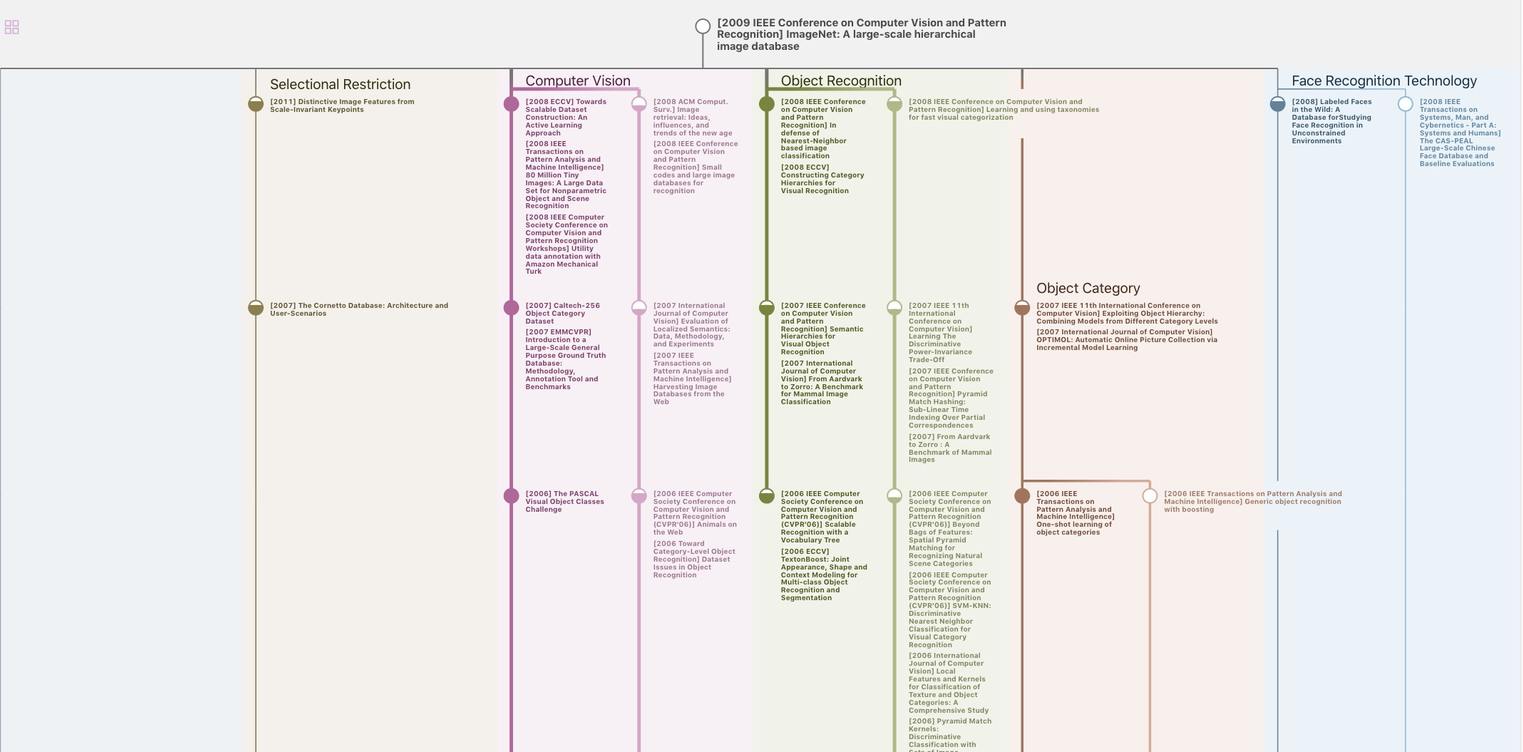
生成溯源树,研究论文发展脉络
Chat Paper
正在生成论文摘要