Generalizing Few-Shot Named Entity Recognizers to Unseen Domains with Type-Related Features.
CoRR(2023)
摘要
Few-shot named entity recognition (NER) has shown remarkable progress in identifying entities in low-resource domains. However, few-shot NER methods still struggle with out-of-domain (OOD) examples due to their reliance on manual labeling for the target domain. To address this limitation, recent studies enable generalization to an unseen target domain with only a few labeled examples using data augmentation techniques. Two important challenges remain: First, augmentation is limited to the training data, resulting in minimal overlap between the generated data and OOD examples. Second, knowledge transfer is implicit and insufficient, severely hindering model generalizability and the integration of knowledge from the source domain. In this paper, we propose a framework, prompt learning with type-related features (PLTR), to address these challenges. To identify useful knowledge in the source domain and enhance knowledge transfer, PLTR automatically extracts entity type-related features (TRFs) based on mutual information criteria. To bridge the gap between training and OOD data, PLTR generates a unique prompt for each unseen example by selecting relevant TRFs. We show that PLTR achieves significant performance improvements on in-domain and cross-domain datasets. The use of PLTR facilitates model adaptation and increases representation similarities between the source and unseen domains.
更多查看译文
关键词
unseen domains,features,few-shot,type-related
AI 理解论文
溯源树
样例
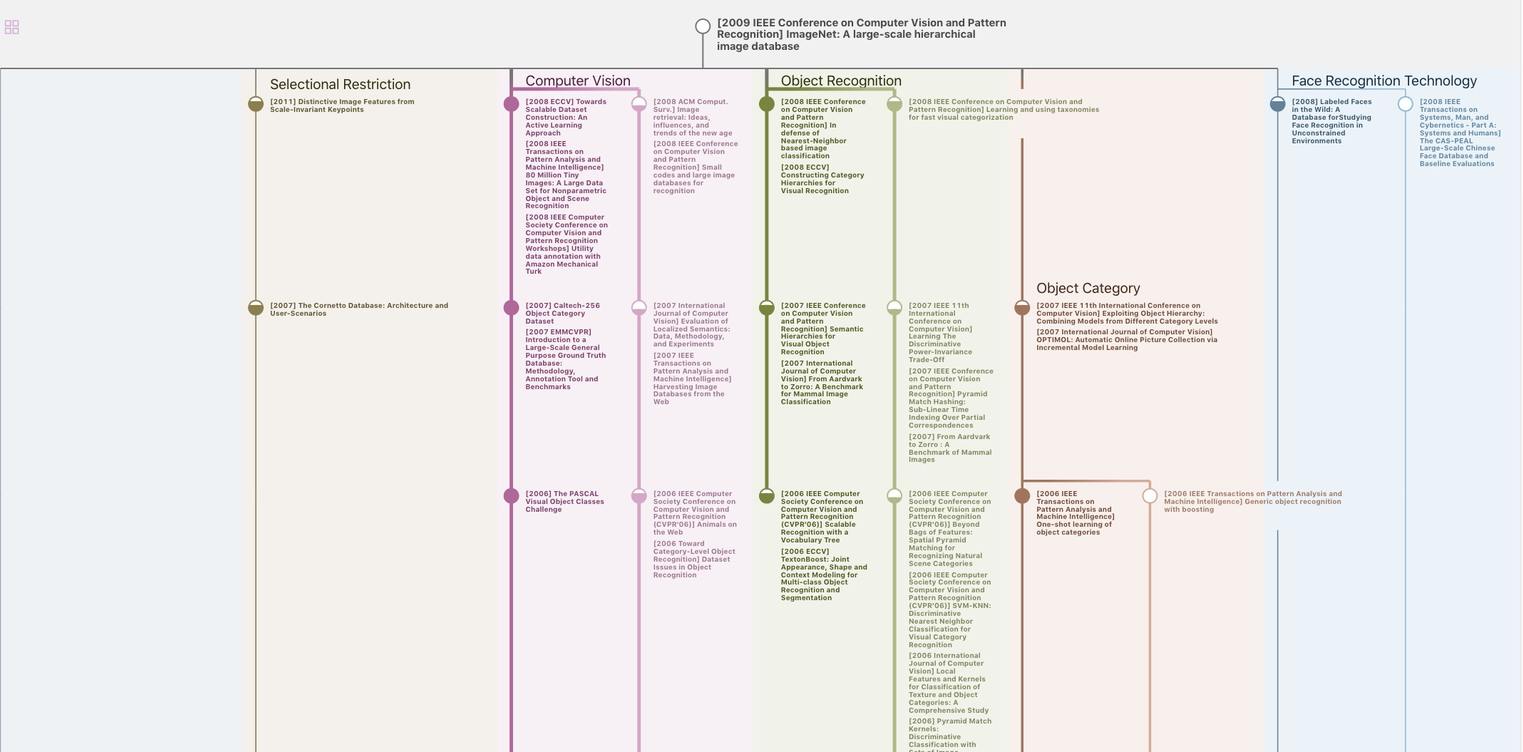
生成溯源树,研究论文发展脉络
Chat Paper
正在生成论文摘要