Risk-Adaptive Local Decision Rules
CoRR(2023)
摘要
For parameterized mixed-binary optimization problems, we construct local decision rules that prescribe near-optimal courses of action across a set of parameter values. The decision rules stem from solving risk-adaptive training problems over classes of continuous, possibly nonlinear mappings. In asymptotic and nonasymptotic analysis, we establish that the decision rules prescribe near-optimal decisions locally for the actual problems, without relying on linearity, convexity, or smoothness. The development also accounts for practically important aspects such as inexact function evaluations, solution tolerances in training problems, regularization, and reformulations to solver-friendly models. The decision rules also furnish a means to carry out sensitivity and stability analysis for broad classes of parameterized optimization problems. We develop a decomposition algorithm for solving the resulting training problems and demonstrate its ability to generate quality decision rules on a nonlinear binary optimization model from search theory.
更多查看译文
关键词
decision,rules,risk-adaptive
AI 理解论文
溯源树
样例
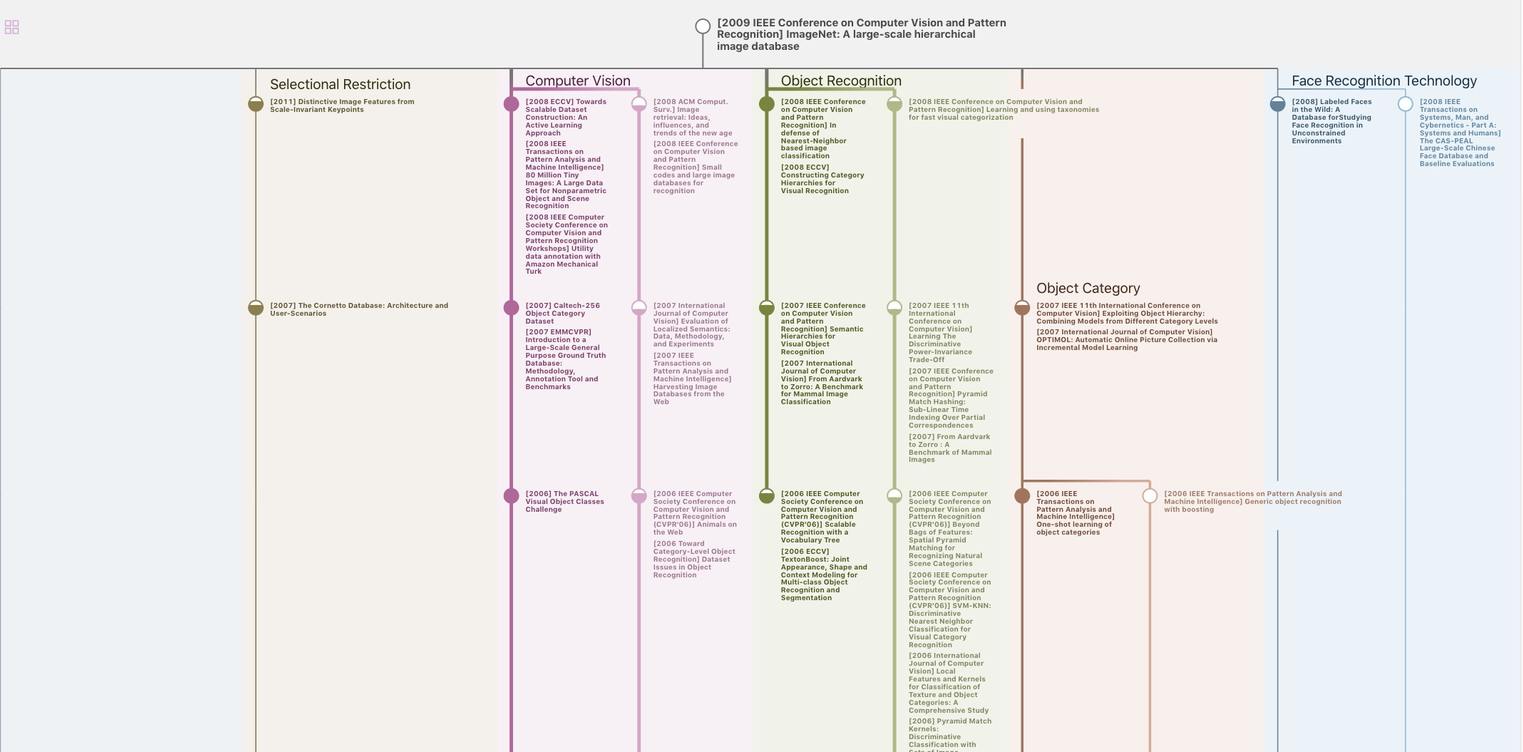
生成溯源树,研究论文发展脉络
Chat Paper
正在生成论文摘要