A robust and powerful replicability analysis for high dimensional data
arxiv(2023)
摘要
Identifying replicable signals across different studies provides stronger
scientific evidence and more powerful inference. Existing literature on high
dimensional applicability analysis either imposes strong modeling assumptions
or has low power. We develop a powerful and robust empirical Bayes approach for
high dimensional replicability analysis. Our method effectively borrows
information from different features and studies while accounting for
heterogeneity. We show that the proposed method has better power than competing
methods while controlling the false discovery rate, both empirically and
theoretically. Analyzing datasets from the genome-wide association studies
reveals new biological insights that otherwise cannot be obtained by using
existing methods.
更多查看译文
AI 理解论文
溯源树
样例
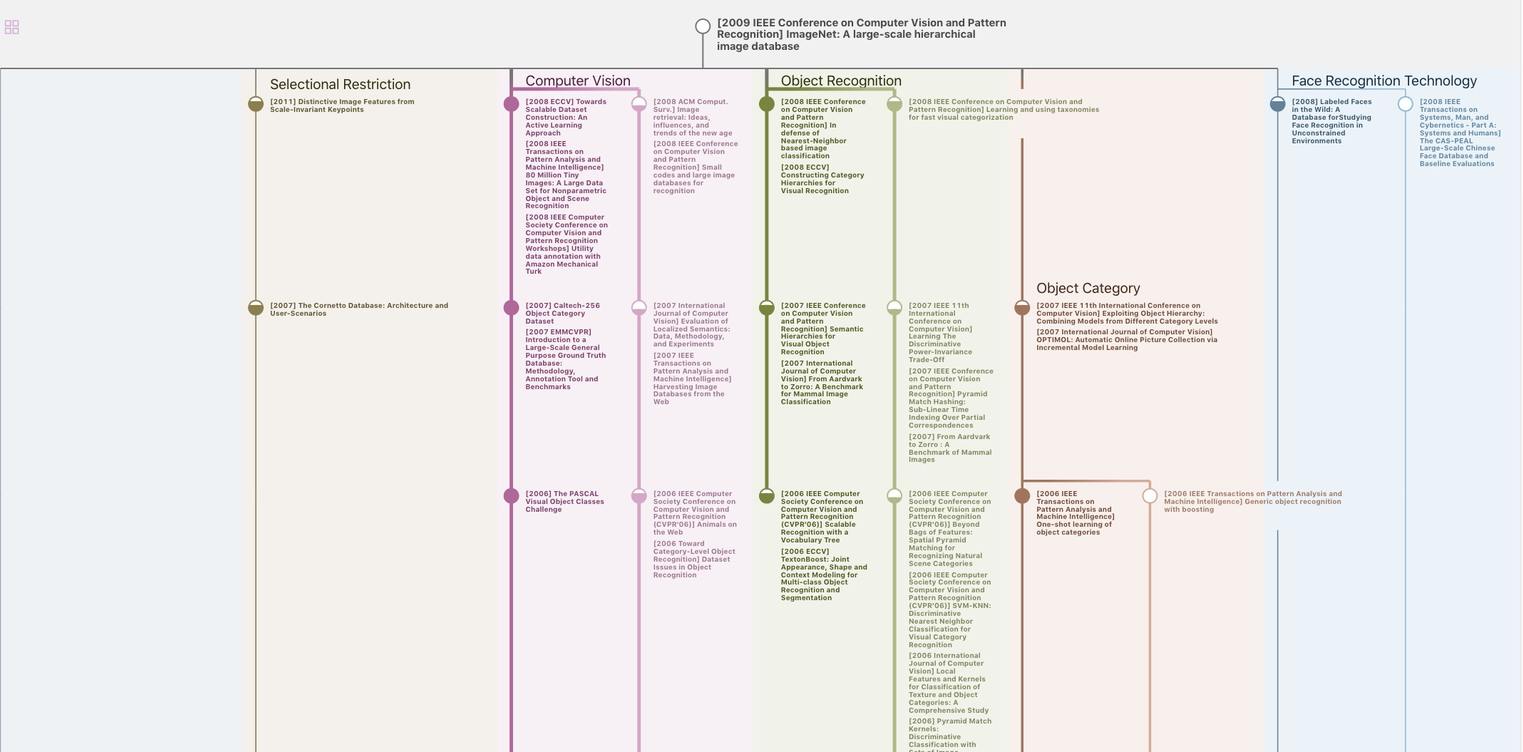
生成溯源树,研究论文发展脉络
Chat Paper
正在生成论文摘要