Multimodal Federated Learning in Healthcare: a Review
CoRR(2023)
摘要
Recent advancements in multimodal machine learning have empowered the
development of accurate and robust AI systems in the medical domain, especially
within centralized database systems. Simultaneously, Federated Learning (FL)
has progressed, providing a decentralized mechanism where data need not be
consolidated, thereby enhancing the privacy and security of sensitive
healthcare data. The integration of these two concepts supports the ongoing
progress of multimodal learning in healthcare while ensuring the security and
privacy of patient records within local data-holding agencies. This paper
offers a concise overview of the significance of FL in healthcare and outlines
the current state-of-the-art approaches to Multimodal Federated Learning (MMFL)
within the healthcare domain. It comprehensively examines the existing
challenges in the field, shedding light on the limitations of present models.
Finally, the paper outlines potential directions for future advancements in the
field, aiming to bridge the gap between cutting-edge AI technology and the
imperative need for patient data privacy in healthcare applications.
更多查看译文
关键词
learning,healthcare
AI 理解论文
溯源树
样例
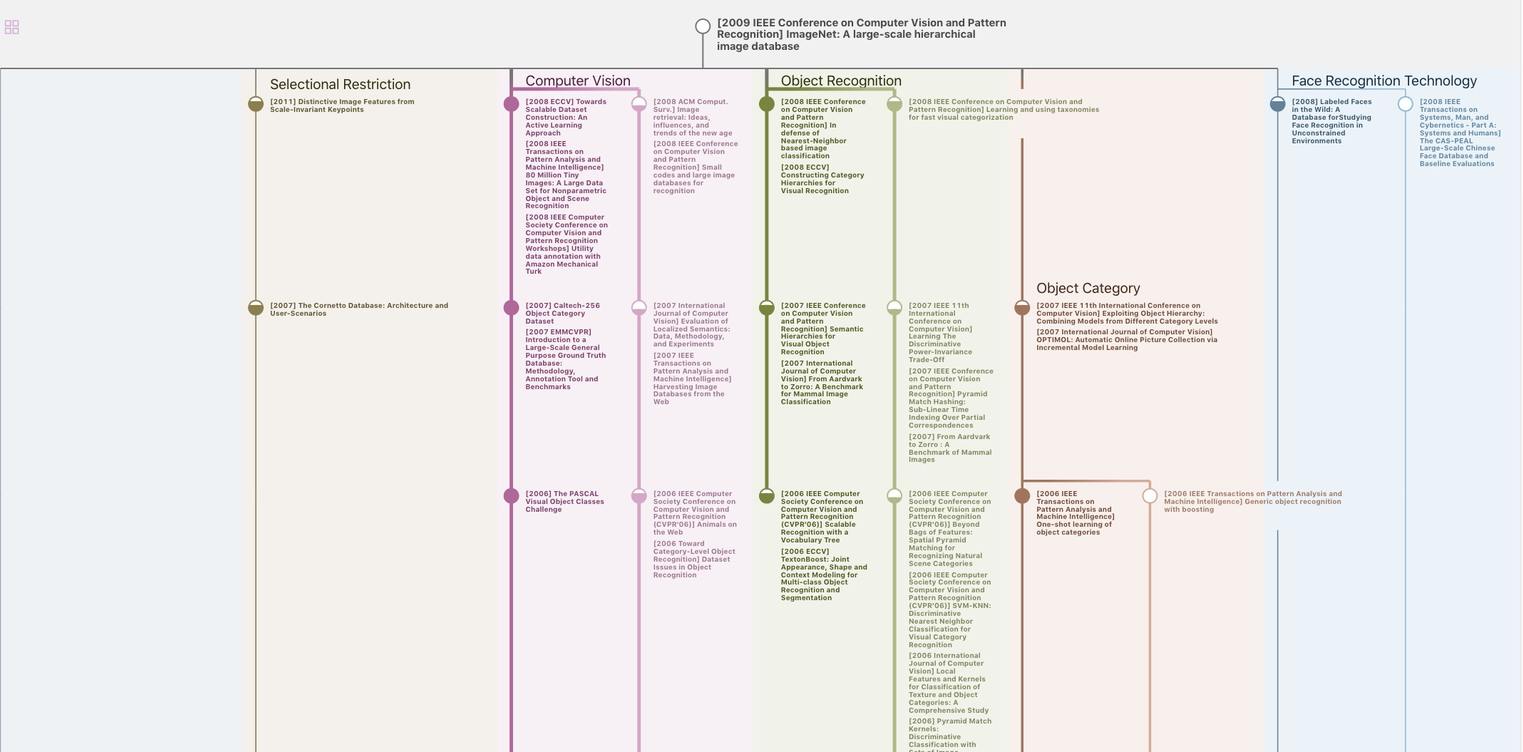
生成溯源树,研究论文发展脉络
Chat Paper
正在生成论文摘要