Modeling Missing at Random Neuropsychological Test Scores Using a Mixture of Binomial Product Experts
arXiv (Cornell University)(2023)
摘要
Multivariate bounded discrete data arises in many fields. In the setting of longitudinal dementia studies, such data is collected when individuals complete neuropsychological tests. We outline a modeling and inference procedure that can model the joint distribution conditional on baseline covariates, leveraging previous work on mixtures of experts and latent class models. Furthermore, we illustrate how the work can be extended when the outcome data is missing at random using a nested EM algorithm. The proposed model can incorporate covariate information, perform imputation and clustering, and infer latent trajectories. We apply our model on simulated data and an Alzheimer's disease data set.
更多查看译文
关键词
random neuropsychological test scores,mixture
AI 理解论文
溯源树
样例
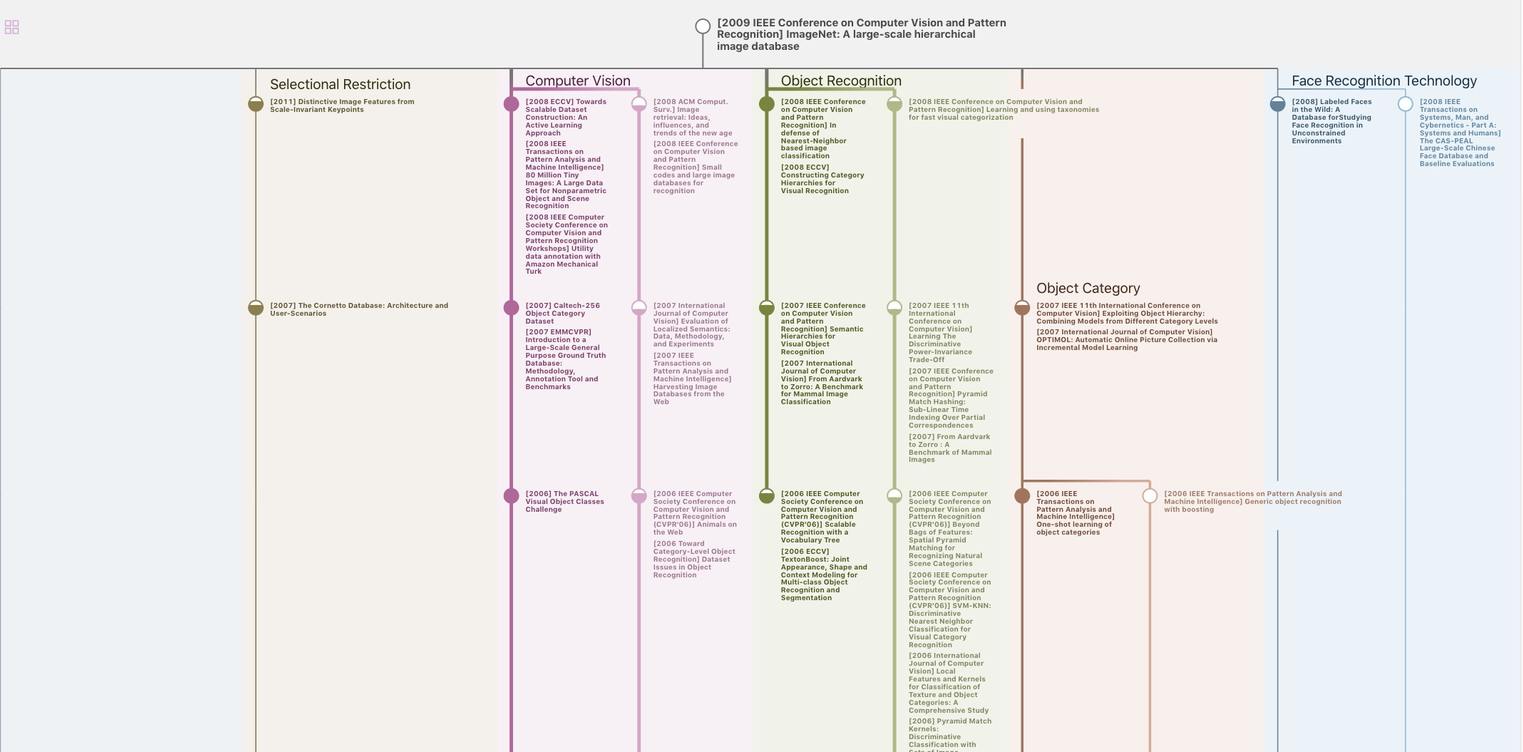
生成溯源树,研究论文发展脉络
Chat Paper
正在生成论文摘要