Using Ensemble Method to Detect Attacks in the Recommender System
IEEE Access(2023)
摘要
Shill attacks are a serious threat to the stability of filtering and recommendation systems. These attacks involve the injection of fake profiles into the system, which can compromise the reliability of system output. Several shilling attack detection techniques have been proposed, but they often have limitations in terms of accuracy. This works presents a enhanced method for detecting attacks in collaborative recommender systems. The proposed method is based on a combination of statistical and machine learning techniques. The statistical techniques are used to identify anomalous user behavior, while the machine learning techniques are used to classify users as either malicious or benign. The main contribution of the proposed method is the use of a hybrid approach that combines the strengths of statistical and machine learning techniques. The statistical techniques are able to identify anomalous user behavior that is not easily detected by machine learning techniques. The machine learning techniques are able to classify users as either malicious or benign with a high degree of accuracy. The proposed method was evaluated on a real-world dataset. The results showed that the proposed method was able to detect attacks with a high degree of accuracy. The proposed method uses a combination of ensemble learning and feature selection to achieve better accuracy than previous methods. The results of the experiments show that the proposed method can achieve an accuracy of up to 99%.
更多查看译文
关键词
Shilling attack detection, profile injection, recommender system, machine learning, ensemble method
AI 理解论文
溯源树
样例
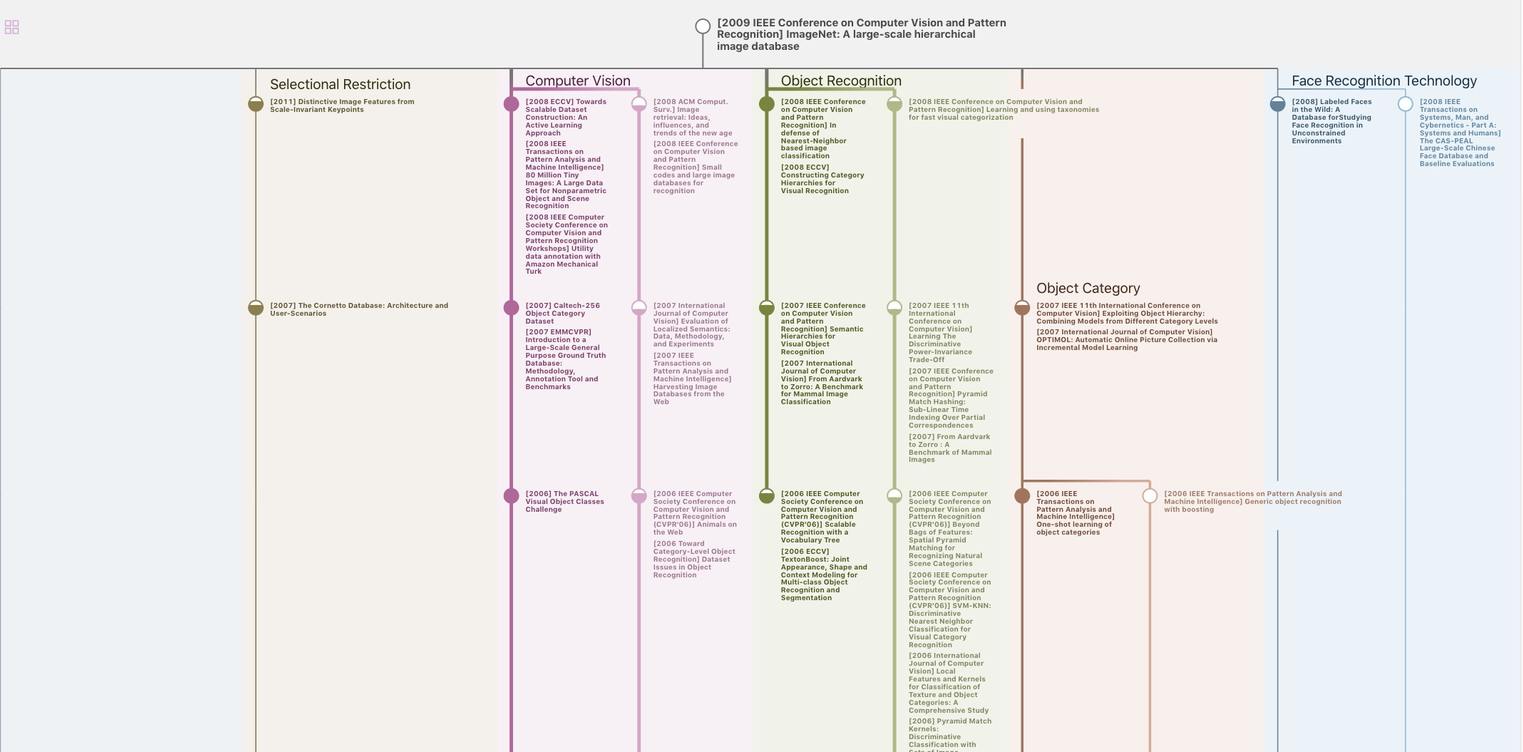
生成溯源树,研究论文发展脉络
Chat Paper
正在生成论文摘要