Cost-Awareness in Multi-Agent Active Search
ECAI 2023(2023)
摘要
Multi-agent active search requires autonomous agents to choose sensing actions that efficiently locate targets. In a realistic setting, agents also must consider the costs that their decisions incur. Previously proposed active search algorithms simplify the problem by ignoring uncertainty in the agent’s environment, using myopic decision making, and/or overlooking costs. In this paper, we introduce an online active search algorithm to detect targets in an unknown environment by making adaptive cost-aware decisions regarding the agent’s actions. Our algorithm proposes an online lookahead planner that combines priniciples from Monte Carlo Tree Search, Thompson sampling and pareto-optimal confidence bounds for decentralized multi-agent multi-objective optimization in an unknown environment. We analyze the algorithm’s performance in simulation to show its efficacy in cost-aware active search.
更多查看译文
关键词
search,cost-awareness,multi-agent
AI 理解论文
溯源树
样例
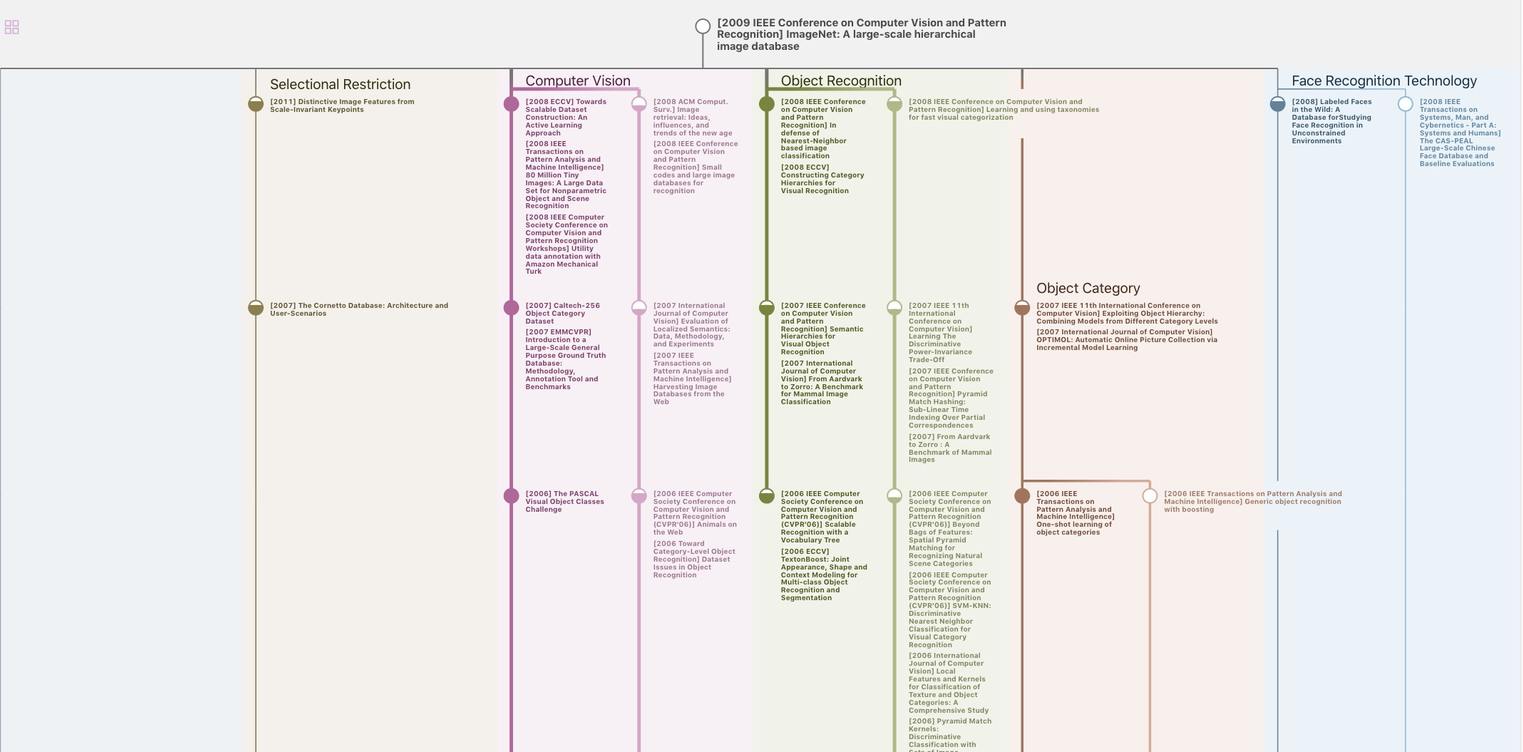
生成溯源树,研究论文发展脉络
Chat Paper
正在生成论文摘要