LoSS: Local Structural Separation Hypergraph Convolutional Neural Network
ECAI 2023(2023)
摘要
Graph classification is a classic problem with practical applications in many real-life scenes. Existing graph neural networks, including GCN, GAT, and GIN, are proposed to extract useful features from complex graph structures. However, most existing methods’ feature extraction and aggregation inevitably mix the useful and redundant features, which will disturb the final classification performance. In this paper, to handle the above drawback, we put forward the Local Structural Separation Hypergraph Convolutional Neural Network (LoSS) based on two discoveries: most graph classification tasks only focus on a few groups of adjacent nodes, and different categories have their specific high response bits in graph embeddings. In LoSS, we first decouple the original graph into different hypergraphs and aggregate the features in each substructure, which aims to find useful features for the final classification. Next, the low-correlation feature suppression strategy is devised to suppress the irrelevant node-level and bit-level features in the forward inference process, effectively reducing the disturbance of redundant features. Experiments on five datasets show that the proposed LoSS can effectively locate and aggregate useful hypergraph features and achieve SOTA performance compared with existing methods.
更多查看译文
关键词
convolutional neural network,neural network
AI 理解论文
溯源树
样例
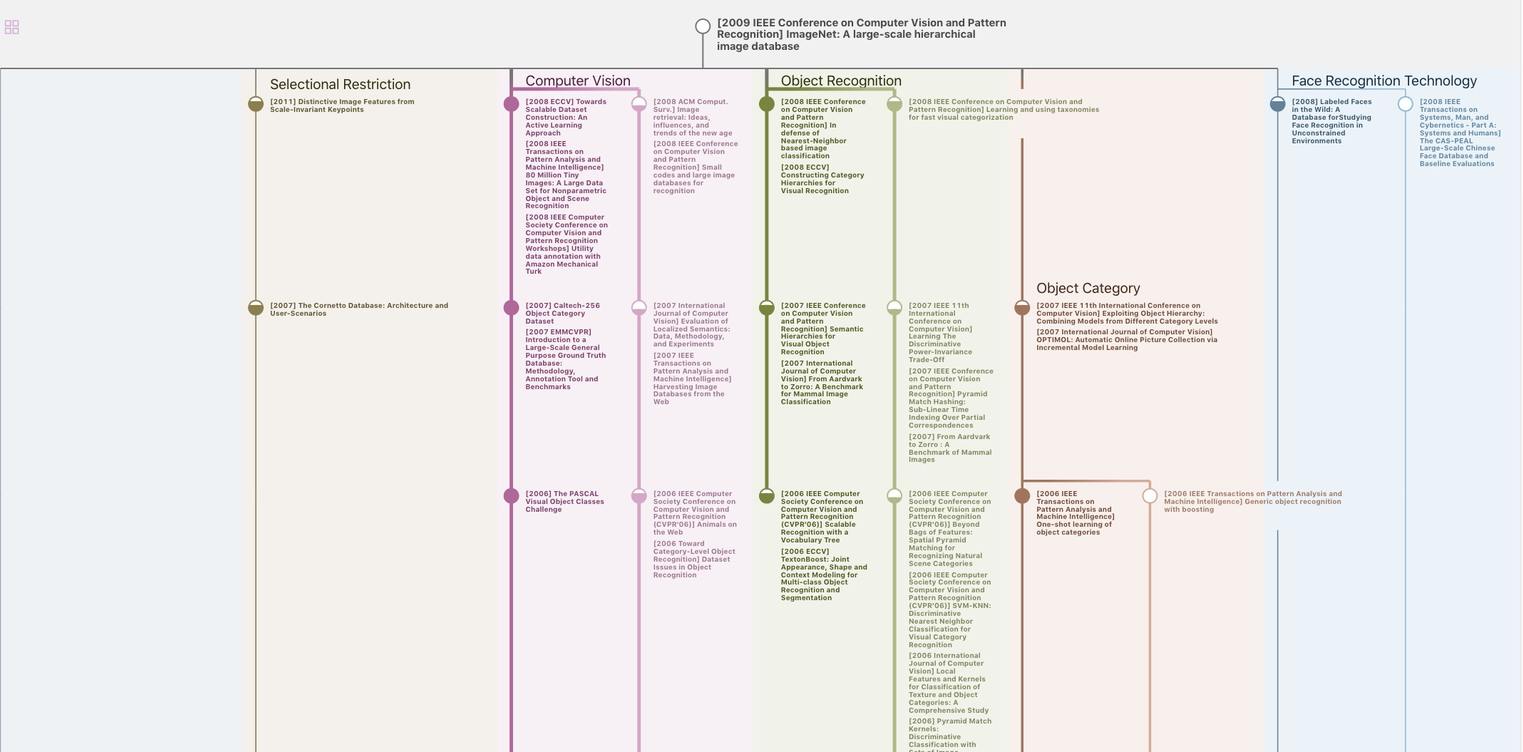
生成溯源树,研究论文发展脉络
Chat Paper
正在生成论文摘要