Theoretical remarks on feudal hierarchies and reinforcement learning
ECAI 2023(2023)
摘要
Hierarchical reinforcement learning is an increasingly demanded resource for learning to make sequential decisions towards long term goals with successful credit assignment and temporal abstraction. Feudal hierarchies are among the most deployed frameworks. However, there is lack of formalism over the hierarchical structure and of theoretical guarantees. We formalize the common two-level feudal hierarchy as two Markov decision processes, with the one on the high-level being dependent on the policy executed at the low-level. Despite the non-stationarity raised by the dependency, we show that each of the processes presents stable behavior. We then build on the first result to show that, regardless of the convergent learning algorithm used for the low-level, convergence of both prediction and control algorithms at the high-level is guaranteed with probability 1. Our results contribute with theoretical support for the use of feudal hierarchies in combination with standard reinforcement learning methods at each level.
更多查看译文
关键词
feudal hierarchies,reinforcement,theoretical remarks,learning
AI 理解论文
溯源树
样例
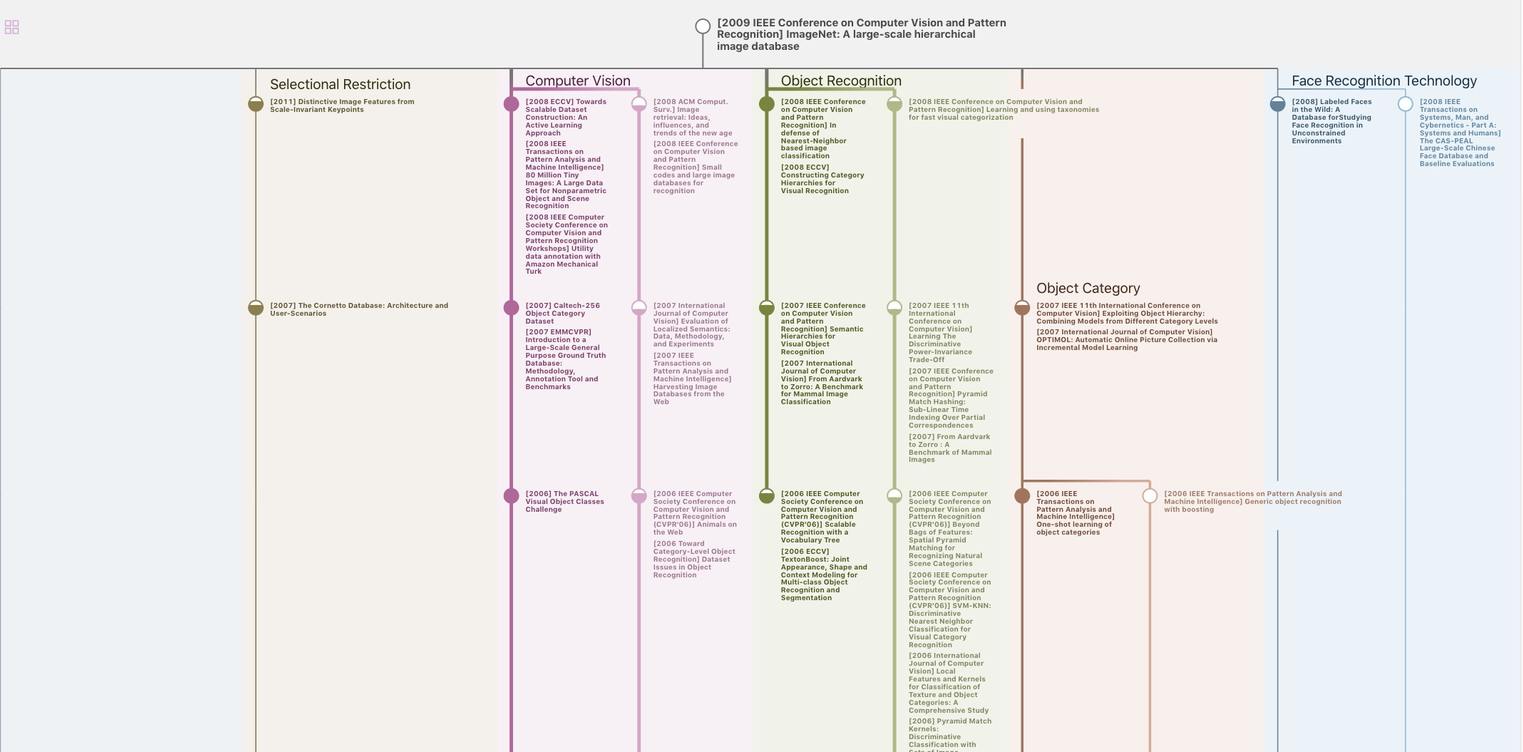
生成溯源树,研究论文发展脉络
Chat Paper
正在生成论文摘要