Application of Clustering Techniques for Improved Energy Benchmarking on Deep-Level Mines
ENERGIES(2023)
摘要
The South African mining industry relies on coal-based energy to run operations, with the sector utilising 29.6 terawatt-hours of energy in 2018. However, energy availability constraints and swelling electricity prices in the country have instilled a greater emphasis on energy management practices to reduce waste and maintain profitability. Energy benchmarking is a popular and effective energy management method applied in industry, but the current methods in the mining sector use the average energy usage of various mining shafts over different intervals to develop benchmarks. This may lead to skewed benchmarks when anomalous energy usage is present within the interval or when vastly different energy users are aggregated. However, clustering-based benchmarking techniques have been applied successfully in other industries to compare similar energy users accurately. This study developed and applied a clustering-based benchmarking method to evaluate the performance of different mining shafts at a deep-level gold mine in South Africa. The K-means unsupervised learning clustering algorithm was used to group similar energy users and benchmark different mining shafts using ordinary least squares regression equations. Compared to traditional methods, the benefit of clustered energy usage benchmarks is shown by more accurately evaluating energy performance and facilitating focused energy management responses.
更多查看译文
关键词
energy management,deep-level mine,benchmarking,unsupervised learning,clustering
AI 理解论文
溯源树
样例
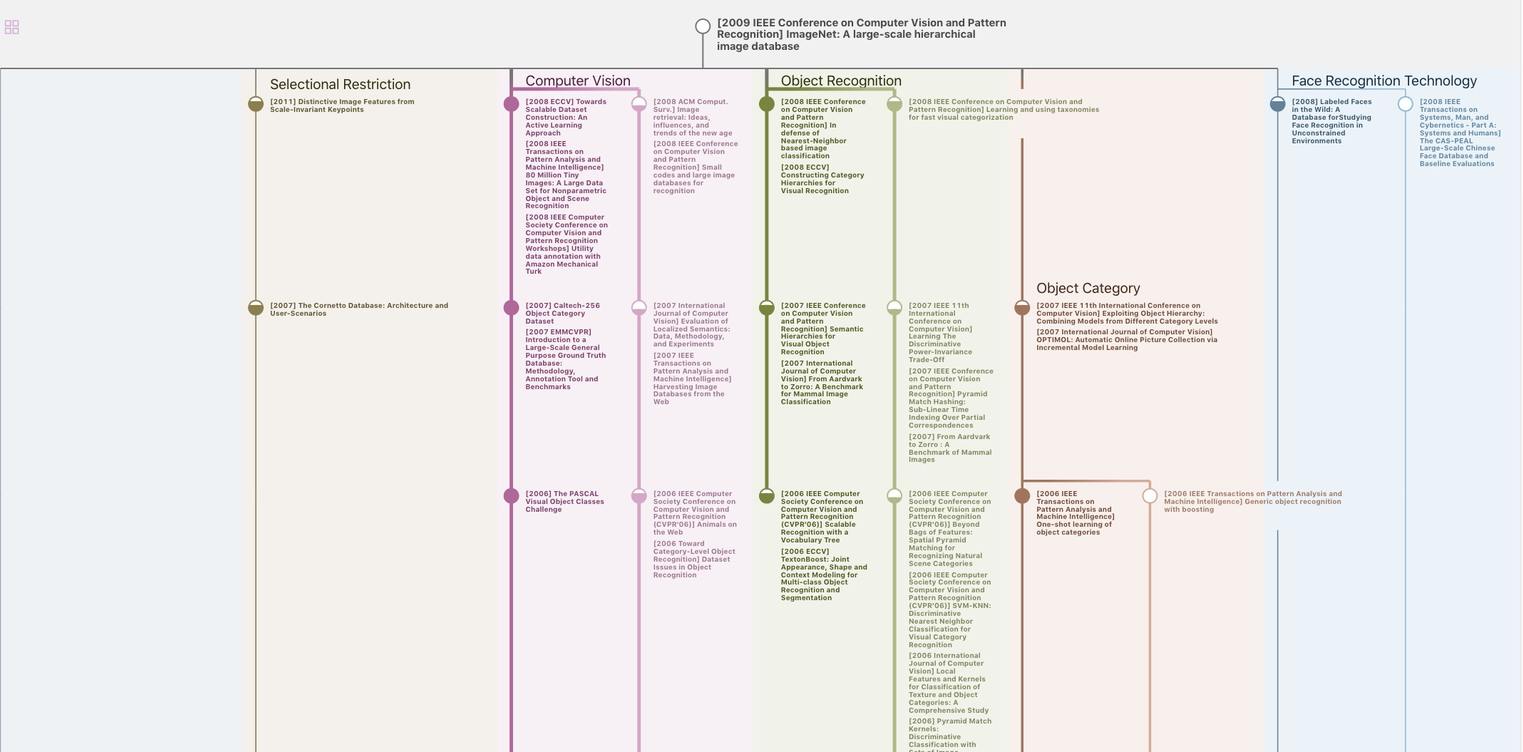
生成溯源树,研究论文发展脉络
Chat Paper
正在生成论文摘要