Multi-Scale Feature Fusion Based on PVTv2 for Deep Hash Remote Sensing Image Retrieval
Remote Sensing(2023)
摘要
For high-resolution remote sensing image retrieval tasks, single-scale features cannot fully express the complexity of the image information. Due to the large volume of remote sensing images, retrieval requires extensive memory and time. Hence, the problem of how to organically fuse multi-scale features and enhance retrieval efficiency is yet to be resolved. We propose an end-to-end deep hash remote sensing image retrieval model (PVTA_MSF) by fusing multi-scale features based on the Pyramid Vision Transformer network (PVTv2). We construct the multi-scale feature fusion module (MSF) by using a global attention mechanism and a multi-head self-attention mechanism to reduce background interference and enhance the representation capability of image features. Deformable convolution is introduced to address the challenge posed by varying target orientations. Moreover, an intra-class similarity (ICS) loss is proposed to enhance the discriminative capability of the hash feature by minimizing the distance among images of the same category. The experimental results show that, compared with other state-of-the-art methods, the proposed hash feature could yield an excellent representation of remote sensing images and improve remote sensing image retrieval accuracy. The proposed hash feature can gain an increase of 4.2% and 1.6% in terms of mAP on the UC Merced and NWPU-RESISC45 datasets, respectively, in comparison with other methods.
更多查看译文
关键词
remote sensing image retrieval,PVTv2,multi-scale feature fusion,hash learning,attention mechanism
AI 理解论文
溯源树
样例
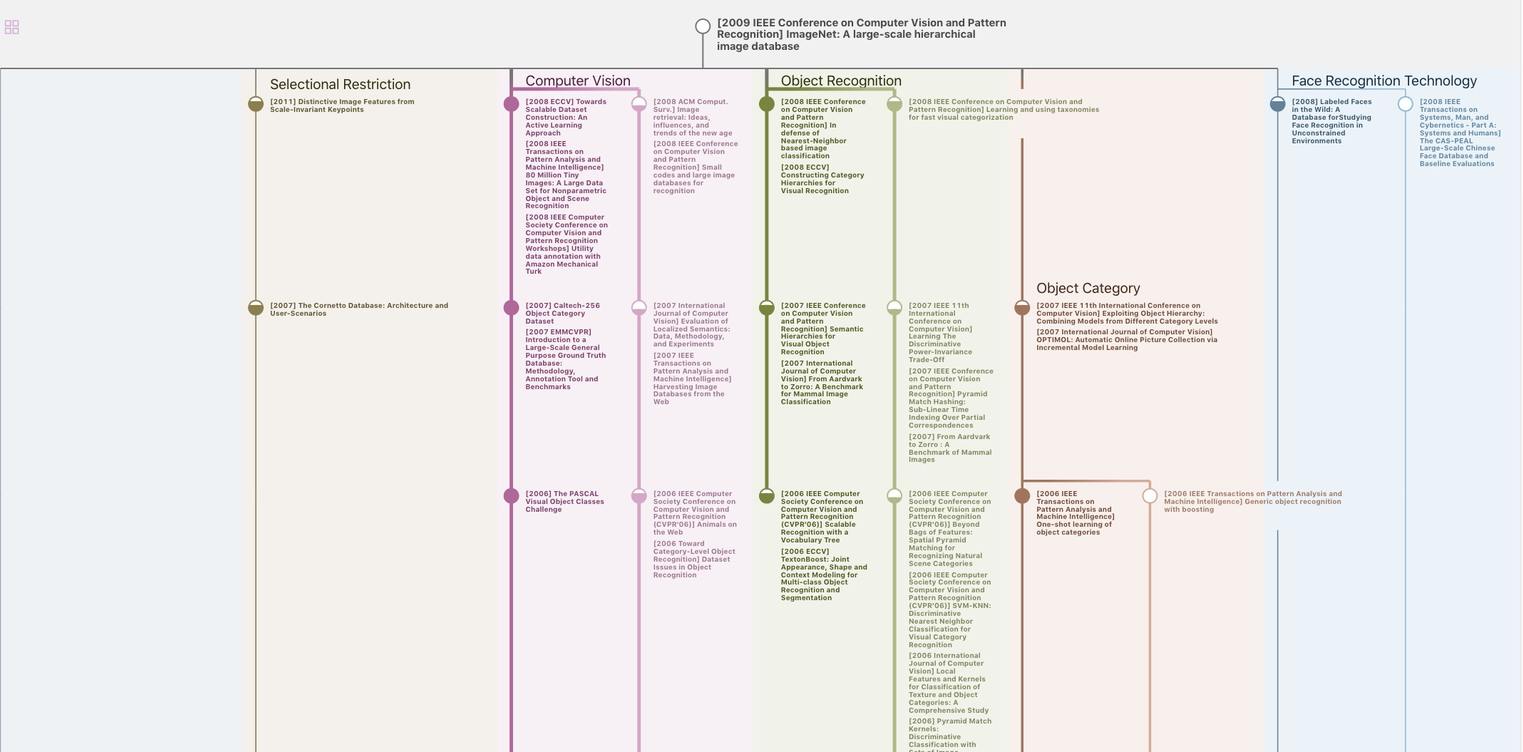
生成溯源树,研究论文发展脉络
Chat Paper
正在生成论文摘要