Making Multimodal Generation Easier: When Diffusion Models Meet LLMs
CoRR(2023)
摘要
We present EasyGen, an efficient model designed to enhance multimodal understanding and generation by harnessing the capabilities of diffusion models and large language models (LLMs). Unlike existing multimodal models that predominately depend on encoders like CLIP or ImageBind and need ample amounts of training data to bridge the gap between modalities, EasyGen is built upon a bidirectional conditional diffusion model named BiDiffuser, which promotes more efficient interactions between modalities. EasyGen handles image-to-text generation by integrating BiDiffuser and an LLM via a simple projection layer. Unlike most existing multimodal models that are limited to generating text responses, EasyGen can also facilitate text-to-image generation by leveraging the LLM to create textual descriptions, which can be interpreted by BiDiffuser to generate appropriate visual responses. Extensive quantitative and qualitative experiments demonstrate the effectiveness of EasyGen, whose training can be easily achieved in a lab setting. The source code is available at https://github.com/zxy556677/EasyGen.
更多查看译文
关键词
diffusion models meet llms,multimodal generation
AI 理解论文
溯源树
样例
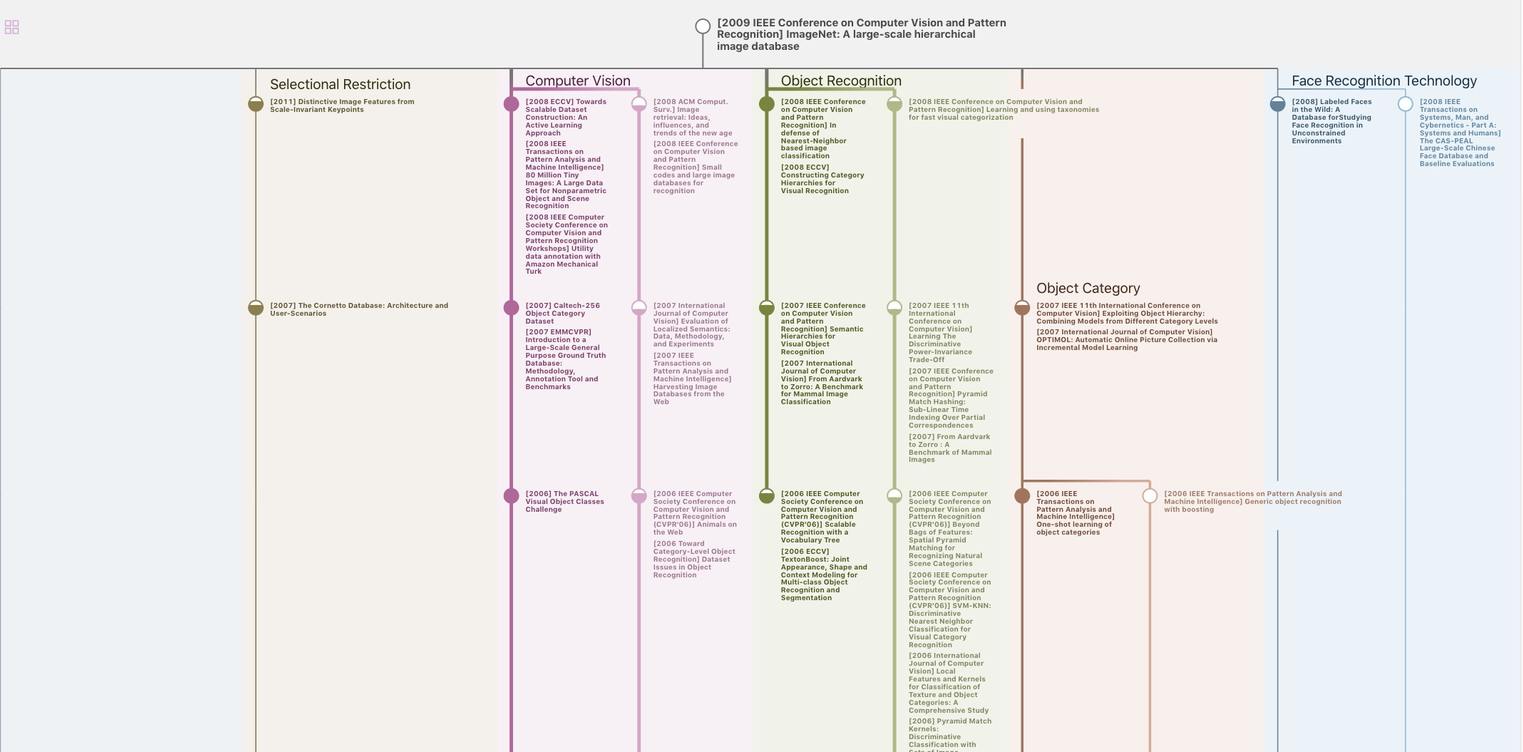
生成溯源树,研究论文发展脉络
Chat Paper
正在生成论文摘要