Deep nonlocal low-rank regularization for complex-domain pixel super-resolution
Optics letters(2023)
摘要
Pixel super-resolution (PSR) has emerged as a promising technique to break the sampling limit for phase imaging systems. However, due to the inherent nonconvexity of phase retrieval problem and super-resolution process, PSR algorithms are sensitive to noise, leading to reconstruction quality inevitably deteriorating. Following the plug-and-play framework, we introduce the nonlocal lowrank (NLR) regularization for accurate and robust PSR, achieving a state-of-the-art performance. Inspired by the NLR prior, we further develop the complex-domain nonlocal low-rank network (CNLNet) regularization to perform nonlocal similarity matching and low-rank approximation in the deep feature domain rather than the spatial domain of conventional NLR. Through visual and quantitative comparisons, CNLNet-based reconstruction shows an average 1.4 dB PSNR improvement over conventional NLR, outperforming existing algorithms under various scenarios. (c)
更多查看译文
关键词
low-rank,complex-domain,super-resolution
AI 理解论文
溯源树
样例
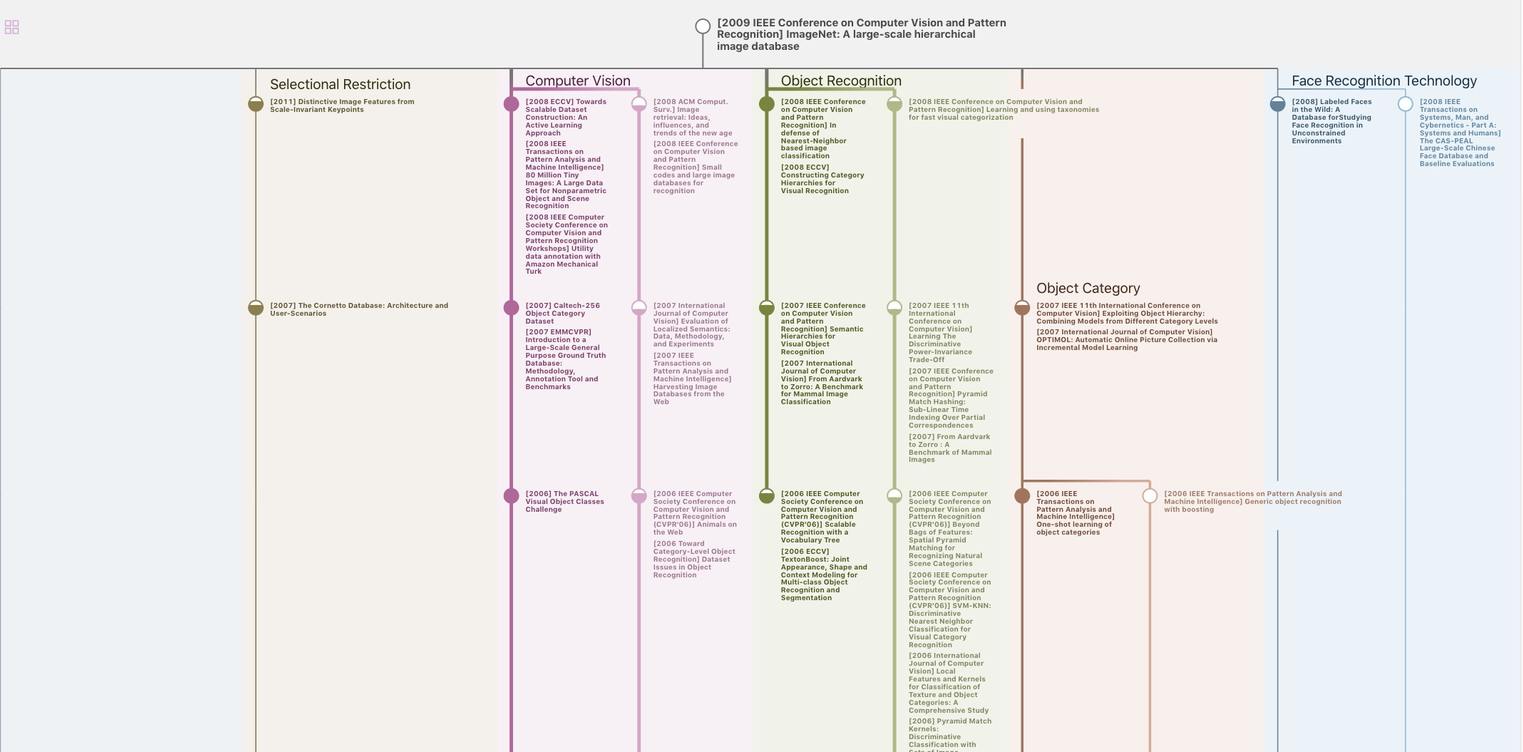
生成溯源树,研究论文发展脉络
Chat Paper
正在生成论文摘要